The term research design is used in two ways in the nursing literature. Some consider research design to be the entire strategy for the study, from identification of the problem to final plans for data collection. Others limit design to clearly defined structures within which the study is implemented. In this text, the first definition refers to the research methodology and the second is a definition of the research design. The research design of a study is the end result of a series of decisions you will make concerning how best to implement your study. The design is closely associated with the framework of the study. As a blueprint, the design is not specific to a particular study but rather is a broad pattern or guide that can be applied to many studies (see Chapter 11 for different types of quantitative research designs). Just as the blueprint for a house must be individualized to the house being built, so the design must be made specific to a study. Using the problem statement, framework, research questions, and clearly defined variables, you can map out the design to achieve a detailed research plan for collecting and analyzing data. The first assumption you must make in examining causality is that causes lead to effects. Some of the ideas related to causation emerged from the logical positivist philosophical tradition. Hume, a positivist, proposed that the following three conditions must be met to establish causality (1) there must be a strong relationship between the proposed cause and the effect; (2) the proposed cause must precede the effect in time; and (3) the cause has to be present whenever the effect occurs. Cause, according to Hume, is not directly observable but must be inferred (Kerlinger & Lee, 2000; Shadish, Cook, & Campbell, 2002). A philosophical group known as essentialists proposed that two concepts must be considered in determining causality: necessary and sufficient. The proposed cause must be necessary for the effect to occur. (The effect cannot occur unless the cause first occurs.) The proposed cause must also be sufficient (requiring no other factors) for the effect to occur. This leaves no room for a variable that may sometimes, but not always, serves as the cause of an effect. John Stuart Mill, another philosopher, added a third idea related to causation. He suggested that, in addition to the preceding criteria for causation, there must be no alternative explanations for why a change in one variable seems to lead to a change in a second variable (Campbell & Stanley, 1963). Causes are frequently expressed within the propositions of a theory. Testing the accuracy of these theoretical statements indicates the usefulness of the theory (Fawcett & Garity, 2009). A theoretical understanding of causation is considered important because it improves our ability to predict and, in some cases, to control events in the real world. The purpose of an experimental design is to examine cause and effect. The independent variable in a study is expected to be the cause, and the dependent variable is expected to reflect the effect of the independent variable. Cook and Campbell (1979) have suggested three levels of causal assertions that one must consider in establishing causality. Molar causal laws relate to large and complex objects. Intermediate mediation considers causal factors operating between molar and micro levels. Micromediation examines causal connections at the level of small particles, such as atoms. Cook and Campbell (1979) used the example of turning on a light switch, which causes the light to come on (molar). An electrician would tend to explain the cause of the light coming on in terms of wires and electrical current (intermediate mediation). However, the physicist would explain the cause of the light coming on in terms of ions, atoms, and subparticles (micromediation). Traditional theories of prediction and control are built on theories of causality. The first research designs were also based on causality theory. Nursing science must be built within a philosophical framework of multicausality and probability. The strict senses of single causality and of “necessary and sufficient” are not in keeping with the progressively complex, holistic philosophy of nursing. To understand multicausality and increase the probability of being able to predict and control the occurrence of an effect, researchers need to comprehend both wholes and parts (Fawcett & Garity, 2009; Shadish et al., 2002). Practicing nurses must be aware of the molar, intermediate mediational, and micromediational aspects of a particular phenomenon. A variety of differing approaches, reflecting both qualitative and quantitative research, are necessary to develop a knowledge base for nursing. Some see explanation and causality as different and perhaps opposing forms of knowledge. Nevertheless, the nurse must join these forms of knowledge, sometimes within the design of a single study, to acquire the knowledge needed for nursing practice (Creswell, 2009; Marshall & Rossman, 2011). Bias is of great concern in research because of the potential effect on the meaning of the study findings. Any component of the study that deviates or causes a deviation from true measure leads to error and distorted findings. Many factors related to research can be biased: the researcher, the measurement methods, the individual subjects, the sample, the data, and the statistics (Grove, 2007; Thompson, 2002; Waltz, Strickland, & Lenz, 2010). In critically appraising a study, you need to look for possible biases in these areas. An important concern in designing a study is to identify possible sources of bias and eliminate or avoid them. If they cannot be avoided, you need to design your study to control them. Designs, in fact, are developed to reduce the possibilities of bias (Shadish et al., 2002). Manipulation tends to have a negative connotation and is associated with one person underhandedly maneuvering a second person so that he or she behaves or thinks in the way the first person desires. Denotatively, to manipulate means to move around or to control the movement of something, such as manipulating a syringe to give an injection. The major role of nurses is to implement interventions that involve manipulation of events related to patients and their environment to improve their health. Manipulation has a specific meaning when used in experimental or quasi-experimental research because it is the manipulation or implementation of the study treatment or intervention. The experimental group receives the treatment or intervention during a study and the control group does not. For example, in a study on preoperative care, preoperative relaxation therapy might be manipulated so that the experimental group receives the treatment and the control group does not. In a study on oral care, the frequency of care might be manipulated to determine its effect on patient outcomes (Doran, 2011). In nursing research, when experimental designs are used to explore causal relationships, the nurse must be free to manipulate the variables under study. For example, in a study of pain management, if the freedom to manipulate pain control measures is under the control of someone else, a bias is introduced into the study. In qualitative, descriptive, and correlational studies, the researcher does not attempt to manipulate variables. Instead, the purpose is to describe a situation as it exists (Marshall & Rossman, 2011; Munhall, 2012). Control means having the power to direct or manipulate factors to achieve a desired outcome. In a study of pain management, one must be able to control interventions to relieve pain. The idea of control is important in research, particularly in experimental and quasi-experimental studies. The more control the researcher has over the features of the study, the more credible the study findings. The purpose of research designs is to maximize control factors in the study (Shadish et al., 2002). Shadish et al. (2002) have described four types of validity: statistical conclusion validity, internal validity, construct validity, and external validity. These types of design validity need to be critically appraised for strengths and possible threats in published studies. When conducting a study, you will be confronted with major decisions about the four types of design validity. To make these decisions, you must address a variety of questions, such as the following: 1. Is there a relationship between the two variables? (statistical conclusion validity) 2. Given that there is a relationship, is it possibly causal from the independent variable to the dependent variable, or would the same relationship have been obtained in the absence of any treatment or intervention? (internal validity) 3. Given that the relationship is probably causal and is reasonably known to be from one variable to another, what are the particular cause-and-effect constructs involved in the relationship? (construct validity) 4. Given that there is probably a causal relationship from construct A to construct B, can this relationship be generalized across persons, settings, and times? (external validity) (Cook & Campbell, 1979; Shadish et al., 2002) Low statistical power increases the probability of concluding that there is no significant difference between samples when actually there is a difference (Type II error, failing to reject a false null) (see Chapter 8 for discussion of the null hypothesis). A Type II error is most likely to occur when the sample size is small or when the power of the statistical test to determine differences is low (Aberson, 2010). The concept of statistical power and strategies to improve it are discussed in Chapters 15 and 21. Most statistical tests have assumptions about the data collected, such as the following: (1) the data are at least at the interval level; (2) the sample was randomly obtained; and (3) the distribution of scores was normal. If these assumptions are violated, the statistical analysis may provide inaccurate results (Corty, 2007; Grove, 2007). The assumptions of statistical tests commonly conducted in nursing studies are provided in Chapters 23, 24, and 25. A serious concern in research is incorrectly concluding that a relationship or difference exists when it does not (Type I error, rejecting a true null). The risk of Type I error increases when the researcher conducts multiple statistical analyses of relationships or differences; this procedure is referred to as fishing. When fishing is used, a given portion of the analyses shows significant relationships or differences simply by chance. For example, the t-test is commonly used to make multiple statistical comparisons of mean differences in a single sample (Kerlinger & Lee, 2000). This procedure increases the risk of a Type I error because some of the differences found in the sample occurred by chance and are not actually present in the population. Multivariate statistical techniques have been developed to deal with this error rate problem (Goodwin, 1984). Fishing and error rate problems are discussed in Chapter 21. The technique of measuring variables must be reliable to reveal true differences. A measure is a reliable measure if it gives the same result each time the same situation or factor is measured. If a scale is used to measure anxiety, it should give the same score (be reliable) if repeatedly given to the same person in a short time (unless, of course, repeatedly taking the same test causes anxiety to increase or decrease) (Waltz et al., 2010). Physiological measurement methods that consistently measure physiological variables are considered precise (Ryan-Wenger, 2010). For example, a thermometer would be precise if it showed the same temperature reading when tested repeatedly on the same patient within a limited time (see Chapter 16). Intervention reliability ensures that the research treatment is standardized and applied consistently each time it is administered in a study. In some studies, the consistent implementation of the treatment is referred to as intervention fidelity. Intervention fidelity often includes a protocol to standardize the elements of the treatment and a plan for training to ensure consistent implementation of the treatment protocol (Forbes, 2009; Santacroce, Maccarelli, & Grey, 2004). If the method of administering a research intervention varies from one person to another, the chance of detecting a true difference decreases. During the planning and implementation phases, researchers must ensure that the study intervention is provided in exactly the same way each time it is administered to prevent a threat to statistical conclusion design validity. Chapter 14 provides a detailed discussion of types of interventions, intervention development, and intervention fidelity. Internal validity is the extent to which the effects detected in the study are a true reflection of reality rather than the result of extraneous variables. Although internal validity should be a concern in all studies, it is addressed more commonly in relation to studies examining causality than in other studies. When examining causality, the researcher must determine whether the independent and dependent variables may have been affected by a third, often unmeasured, variable (an extraneous variable). Chapter 8 describes the different types of extraneous variables. The possibility of an alternative explanation of cause is sometimes referred to as a rival hypothesis (Shadish et al., 2002). Any study can contain threats to internal design validity, and these validity threats can lead to false-positive or false-negative conclusions. The researcher must ask, “Is there another reasonable (valid) explanation (rival hypothesis) for the finding other than the one I have proposed?” Threats to internal validity are described here. Selection addresses the process by which subjects are chosen to take part in a study and how subjects are grouped within a study. A selection threat is more likely to occur in studies in which randomization is not possible (Kerlinger & Lee, 2000; Thompson 2002). In some studies, people selected for the study may differ in some important way from people not selected for the study. In other studies, the threat is due to differences in subjects selected for study groups. For example, people assigned to the control group could be different in some important way from people assigned to the experimental group. This difference in selection could cause the two groups to react differently to the treatment; in this case, the treatment would not have caused the differences in group responses. The subject attrition threat is due to subjects who drop out of a study before completion. Participants’ attrition becomes a threat when (1) those who drop out of a study are different types of people from those who remain in the study or (2) there is a difference between the kinds of people who drop out of the experimental group and the people who drop out of the control or comparison group (see Chapter 15). Construct validity examines the fit between the conceptual definitions and operational definitions of variables. Theoretical constructs or concepts are defined within the study framework (conceptual definitions). These conceptual definitions provide the basis for the operational definitions of the variables. Operational definitions (methods of measurement) must validly reflect the theoretical constructs. (Theoretical constructs are discussed in Chapter 7; conceptual and operational definitions of concepts and variables are discussed in Chapter 8.) Is use of the measure a valid inference about the construct? By examining construct validity, we can determine whether the instrument actually measures the theoretical construct it purports to measure. The process of developing construct validity for an instrument often requires years of scientific work. When selecting methods of measurement, the researcher must determine the previous development of instrument construct validity (DeVon et al., 2007; Waltz et al., 2010). The threats to construct validity are related both to previous instrument development and to the development of measurement techniques as part of the methodology of a particular study. Threats to construct validity are described here. Mono-operation bias occurs when only one method of measurement is used to assess a construct. When only one method of measurement is used, fewer dimensions of the construct are measured. Construct validity greatly improves if the researcher uses more than one instrument (Waltz et al., 2010). For example, if anxiety were a dependent variable, more than one measure of anxiety could be used. It is often possible to apply more than one measurement of the dependent variable with little increase in time, effort, or cost.
Understanding Quantitative Research Design
http://evolve.elsevier.com/Grove/practice/
Concepts Important to Design
Causality
Multicausality
Causality and Nursing Philosophy
Bias
Manipulation
Control
Study Validity
Statistical Conclusion Validity
Low Statistical Power
Violated Assumptions of Statistical Tests
Fishing and the Error Rate Problem
Reliability of Measures
Reliability of Intervention Implementation
Internal Validity
Selection
Subject Attrition
Construct Validity
Mono-Operation Bias
Stay updated, free articles. Join our Telegram channel
Full access? Get Clinical Tree
Understanding Quantitative Research Design
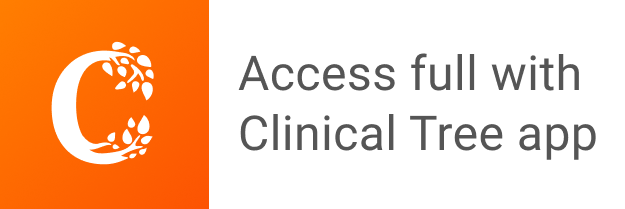