Chapter 25 Biostatistics
Basic Concepts
Test Characteristics
1 What does the sensitivity of a diagnostic test measure?
Sensitivity can be calculated by dividing the number of true positives by the total number of people tested with the disease: true positives/(true positives + false negatives), or a/(a + c) in the 2 × 2 table (Table 25-1). You can also calculate sensitivity by subtracting the false negative rate from 1 (i.e., sensitivity = 1 – false negative rate); however, you will seldom be given the false negative rate on boards.
3 Quick terminology review: Cover the right column in Table 25-2 and define each of the terms in the left column
Term | Definition |
---|---|
True positive | A positive test result in someone who truly has the disease |
False positive | A positive test result in someone who truly does not have the disease |
True negative | A negative test result in someone who truly does not have the disease |
False negative | A negative test result in someone who truly does have the disease |
5 What information is given by the relative risk?
For example, in the 2 × 2 table (Table 25-3), the probability of lung cancer in smokers is 90/100 or .90. The probability of lung cancer in nonsmokers is 10/100, or .10. Therefore, the RR for smoking is .90/.10, or 9. An RR of 9 implies that smokers are 9 times more likely to get lung cancer than nonsmokers.
7 What is the difference between probability and odds and how are they measured?
Odds are measured along a continuum from 0 to infinity.
Probabilities can be easily converted to odds, and vice versa.
8 What is the positive predictive value? negative predictive value?
The positive predictive value (PPV) is the probability that, given a positive test result, the disease in question is actually present. The negative predictive value (NPV) is the probability that disease is absent if there is a negative test result. If a 2 × 2 table is provided, the PPV can be calculated by dividing the number of true positives by the total number of positive test results (a/a + b) and the NPV can be calculated by dividing the number of true negatives by the total number of negative test results (d/c + d) (Table 25-4).
10 How is the positive likelihood ratio used to calculate the positive predictive value?
Expressed in odds, 20% is 1:4, so the PPV of the exercise stress test is calculated as follows:
13 In statistical analyses of differences between groups, a P value is often included to reflect how significant the difference is. What is the meaning of this P value?
14 What are the differences between type I and type II error? How is power related to type II error?
15 What are some determinants that can be used to evaluate the existence of a causal relationship between two variables?
Consistency (e.g., the more studies that support the hypothesis, the better)
Strength of correlation (e.g., the higher the RR, the better)
Biologic plausibility (e.g., colon cancer is unlikely to be caused by ultraviolet light exposure)
Temporality (cause must precede disease outcome)
Supportive experimental studies (e.g., animal studies)
Dose-response relationship (e.g., higher exposure leads to more severe phenotype)
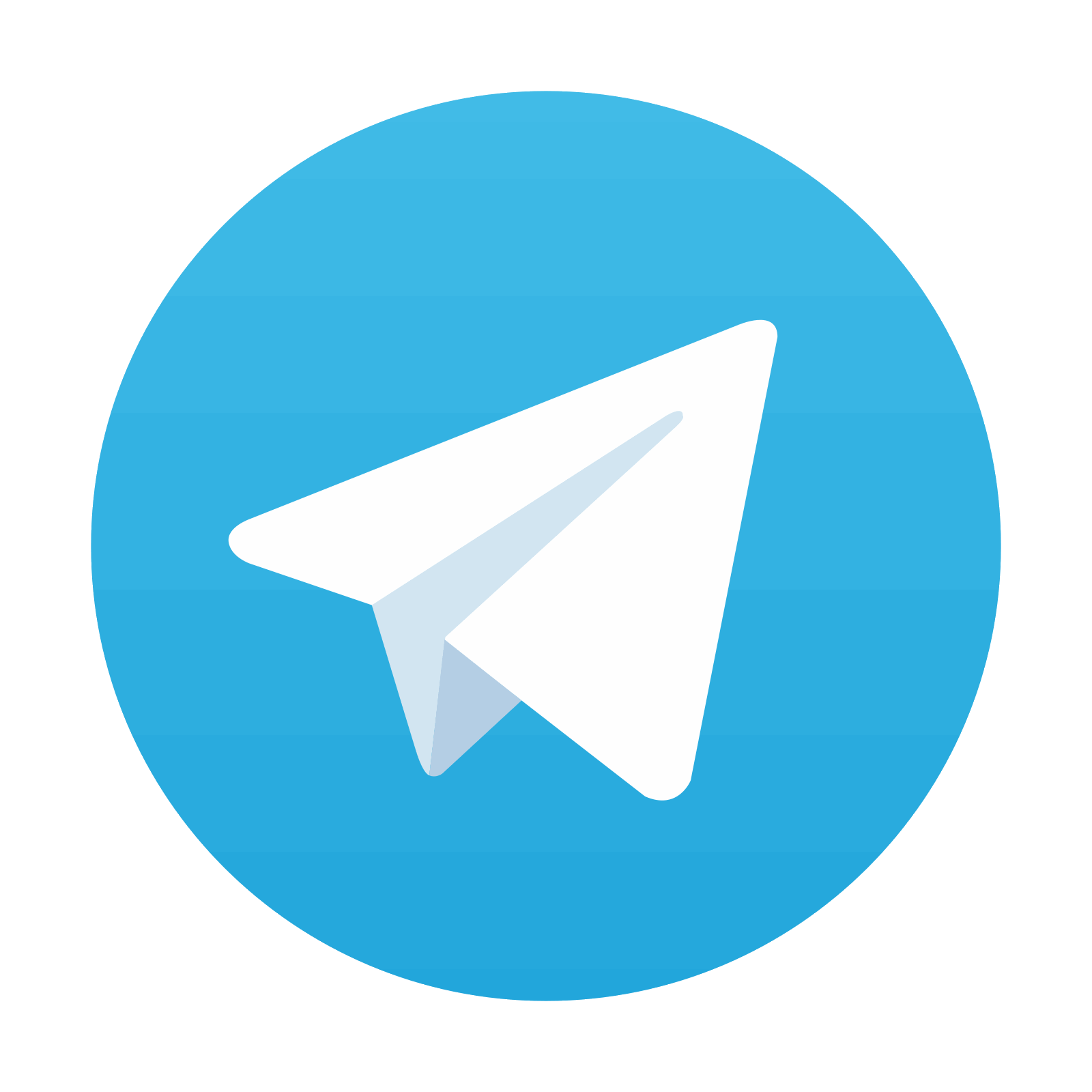
Stay updated, free articles. Join our Telegram channel

Full access? Get Clinical Tree
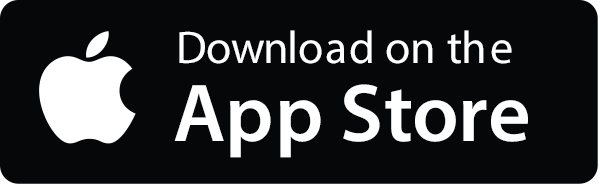
