Experimental-Type Designs
Key terms
Clinical trial methodology
Counterbalance designs
Effect size
Epidemiology
Factorial designs
Manipulation
Meta-analysis
Nonexperimental designs
Pre-experimental designs
Quasi-experimental designs
Randomization
Solomon four-group designs
True-experimental designs
Using the language introduced in Chapter 8, we are ready to examine the characteristics of designs in the experimental-type research tradition. Experimental designs have traditionally been classified as true-experimental, quasi-experimental, pre-experimental, and nonexperimental. The true experiment is the criterion by which all other methodological approaches are judged. Most people think that the only real research or science is the experimental method. Of all the experimental-type designs, the true-experimental design offers the greatest degree of control and internal validity. It is this design and its variations that are used to reveal causal relationships between independent and dependent variables. Also, this design is often upheld as the highest level of scientific evidence, particularly in evidence-based practice model research and ratings that are applied to studies for the development of clinical guidelines as we discuss subsequently.
Although the true-experimental design is continually upheld as the most “objective” and “true” scientific approach, we believe it is important to recognize that every design in the experimental-type tradition has merit and value. The merit and value of a design are based on how well the design answers the research question that is being posed and the level of rigor that the investigator brings to the plan and conduct of the inquiry. This view of research differs from the perspective of the many experimental-type researchers who present the true experiment as the only design of choice and suggest that other designs are deficient or limited.1,2
We suggest that a design in the experimental-type tradition should be chosen purposively because it fits the question, level of theory development, and setting or environment in which the research will be conducted. True experimentation is the best design to use to predict causal relationships, but it may be inappropriate for other forms of inquiry in health and human service settings. In other words, not all research questions seek to predict causal relationships between independent and dependent variables. Moreover, in some cases, using a true-experimental design may present critical ethical concerns such that other design strategies may be more appropriate.
True-experimental designs
To express the structural relationships of true-experimental designs, we use Campbell and Stanley’s classic, widely adopted notation system to diagram a design: X represents the independent variable, O the dependent variable, and R denotes random sample selection,3 as follows:
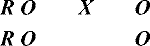
We also find it helpful to use the symbol r to refer to random group assignment in the absence of random sample selection. It is often difficult and frequently inappropriate or unethical for health and human service professionals to select a sample from a larger, predefined population based on random selection (R); rather, typically subjects enter studies on a volunteer basis. Such a sample is one of convenience in which subjects are then randomly assigned to either the experimental or the control group. The addition of “r” denotes this important structural distinction. Designs in which samples are not randomly selected but are randomly assigned still meet the criteria for true experimentation, but as we discuss later, are limited in their external validity, the degree to which the sample represents the population from which it was selected.
True-experimental designs are most commonly thought about when beginning researchers and laypersons hear the word “research.” True-experimental design refers to the classic two-group design in which subjects are randomly selected and randomly assigned (R) to either an experimental or control group condition. Before the experimental condition, all subjects are pretested or observed on a dependent measure (O). In the experimental group the independent variable or experimental condition is imposed (X), and it is withheld in the control group. After the experimental condition, all subjects are posttested or observed on the dependent variable (O).
In this design, the investigator expects to observe no difference between the experimental and control groups on the dependent measure at pretest. In other words, subjects are chosen randomly from a larger pool of potential subjects and then assigned to a group on a “chance-determined” basis; therefore, subjects in both groups are expected to perform similarly. In the example, we would expect that subjects in experimental and control group conditions would have similar shoulder range of motion scores at the first baseline or pretest assessment. However, the investigator anticipates or hypothesizes that differences will occur between experimental and control group subjects on the posttest scores. This expectation is expressed as a null hypothesis, which states that no difference is expected. In a true-experimental design, the investigator always states a null hypothesis that forms the basis for statistical testing. Usually in research reports, however, the alternative (working) hypothesis is stated (i.e., an expected difference). If the investigator’s data analytical procedures reveal a significant difference (one that does not occur by chance) between experimental and control group scores at posttest, the investigator can fail to accept the null hypothesis with a reasonable degree of certainty. In failing to accept the null hypothesis, the investigator accepts with a certain level of confidence that the independent variable or experimental condition (X) caused the outcome observed at posttest time in the experimental group. In other words, the investigator infers that the difference at posttest time is not the result of chance but is caused by participation in the experimental condition.
Three major characteristics of the true-experimental design allow this causal claim to be made (Box 9-1).
Randomization
Randomization occurs at the sample selection phase, the group assignment phase, or both. If random sample selection is accomplished, the design notation appears as presented earlier (R). If randomization occurs only at the group assignment phase, we represent the design as such (r):
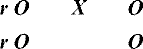
As we noted earlier, this variation has implications for external validity. Remember that a true-experimental design that does not use random sample selection is limited in the extent to which conclusions can be generalized to the population from which the sample is selected. Because subjects are not drawn by chance from a larger identified pool, the generalizability or external validity of findings is limited. However, such a design variation is common in experimental research and can still be used to reveal causal relationships within the sample itself.
Although random sample selection is often not possible to achieve, random assignment of subjects to group conditions based on chance is essential in true experimentation. It enhances the probability that subjects in experimental and control groups will be theoretically equivalent on all major dependent variables at the pretest occasion. Randomization, in principle, equalizes subjects or provides a high degree of assurance that subjects in both experimental and control groups will be comparable at pretest or the initial, baseline measure. How is this possible? By randomizing, people are assigned by chance, and as such, the researcher does not introduce any systematic order to the selection and assignment of the sample. Thus, any influence on one group theoretically will similarly affect the other group as well. In the absence of any other differences that could influence outcome, an observed change in the experimental group at posttest then can be attributed with a reasonable degree of certainty to the experimental condition.
Randomization is a powerful technique that increases control and eliminates bias by neutralizing the effects of extraneous influences on the outcome of a study. For example, the threats to internal validity by historical events and maturation are theoretically eliminated. Based on probability theory, such influences should affect subjects equally in both the experimental and the control group. Without randomization of subjects, you will not have a true-experimental design.
Control Group
We now extend the concept of control introduced in Chapter 8 to refer to the inclusion of a control group in a study. The control group allows the investigator to see what the sample will be without the influence of the experimental condition or independent variable. A control group theoretically performs or remains the same relative to the independent variable at pretest and posttest, since the control group has not had the chance of being exposed to the experimental (or planned change) condition. Therefore, the control group represents the characteristics of the experimental group before being changed by participation in the experimental condition.
The control group is also a mechanism that allows the investigator to examine what has been referred to as the “attention factor,” “Hawthorne effect,” or “halo effect.”4 These three terms all refer to the phenomenon of the subject experiencing change as a result of simply participating in a research project. For example, being the recipient of personal attention from an interviewer during pretesting and posttesting may influence how a subject feels and responds to interview questions. A change in scores in the experimental group may then occur, independent of the effect of the experimental condition. Without a control group, investigators are not able to observe the presence or absence of this phenomenon and are unable to judge the extent to which differences on posttest scores of the experimental group reflect experimental effect, not additional attention.
Interestingly, this attention phenomenon was discovered in the process of conducting research. In 1934, a group of investigators were examining productivity in the Hawthorne automobile plant in Chicago. The research involved interviewing workers. To improve productivity, the investigators recommended that the lighting of the facility be brightened. The researchers noted week after week that productivity increased after each subsequent increase in illumination. To confirm the success of their amazing findings, the researchers then dimmed the light. To their surprise, productivity continued to increase even under this circumstance. In reexamining the research process, they concluded that it was the additional attention given to the workers through the ongoing interview process and their inclusion in the research itself, not the lighting, that caused an increased work effort.5
Manipulation
In a true-experimental design, the independent variable is manipulated either by having it present (in the experimental group) or absent (in the control group). Manipulation is the ability to provide and withhold the independent variable that is unique to the true experiment.
According to Campbell and Stanley,3 true experimentation theoretically controls for each of the seven major threats to internal validity (see Chapter 8). However, when such a true-experimental design is implemented in the health care or human service environment, certain influences may remain as internal threats, thereby decreasing the potential for the investigator to support a cause-and-effect relationship. For example, the selection of a data collection instrument with a learning effect based on repeated testing could pose a significant threat to a study, regardless of how well the experiment is structured. It is also possible for experimental “mortality” to affect outcome, particularly if the groups become nonequivalent as a result of attrition from the experiment. The health or human service environment is complex and does not offer the same degree of control as a laboratory setting. Therefore, in applying the true-experimental design to the health or human service environment, the researcher must carefully examine the particular threats to internal validity and how each can be resolved.
True-experimental design variations
Many design variations of the true experiment have been developed to enhance its internal validity. For example, to assess the effect of the attention factor, a researcher may develop a three-group design. In this structure, subjects are randomly assigned to (1) an experimental condition, (2) an attention control group that receives an activity designed to equalize the attention that subjects receive in the experimental group, or (3) a silent control group that receives no attention other than that obtained naturally during data collection efforts. The term “silent control group” can also refer to the situation in which information is collected on subjects who have no knowledge of their own participation.
Let us examine four basic design variations of the true experiment.
Posttest-Only Designs
Posttest-only designs conform to the norms of experimentation in that they contain the three elements of random assignment, control group, and manipulation. The difference between classic true-experimental design and this variation is the absence of a pretest. The basic design notation for a posttest-only experiment follows for randomly selected and randomly assigned samples:

In a posttest-only design, groups are considered equivalent before the experimental condition as a result of random assignment. Theoretically, randomization should yield equivalent groups. However, the absence of the pretest makes it impossible to determine whether random assignment successfully achieved equivalence between the experimental and control groups on the major dependent variables of the study. Some researchers assume that the control group posttest scores are equivalent to pretest scores for both control and experimental groups. However, caution is advised, especially considering the influence of attention on subjects that we discussed earlier. There are a number of variations of this basic design. For example, the researcher may add other posttest occasions or different types of experimental or control groups.
Posttest-only designs are most valuable when pretesting is not possible or appropriate but the research purpose is to seek causal relationships. Also, a posttest-only design might be chosen if the threat to learning is highly likely with repeated testing using the same measure of the dependent variable.
Solomon Four-Group Designs
More complex experimental structures than those previously discussed, Solomon four-group designs combine the true-experiment and posttest-only designs into one design structure. The strength of this design is that it provides the opportunity to test the potential influence of the test–retest learning phenomenon by adding the posttest-only two-group design to the true-experimental design. This design is noted as follows if random selection occurs:
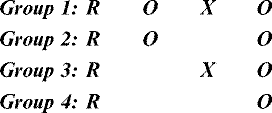
If random assignment without random selection occurs, the notation r would be noted in lower case.
As shown in this notation, Group 3 does not receive the pretest but participates in the experimental condition. Group 4 also does not receive the pretest but serves as a control group. By comparing the posttest scores of all groups, the investigator can evaluate the effect of testing on scores on the posttest and interaction between the test and the experimental condition. The key benefit of this design is its ability to detect interaction effects. An interaction effect refers to changes that occur in the dependent variable as a consequence of the combined influence or interaction of taking the pretest and participating in the experimental condition.
The following example illustrates the power of the Solomon four-group design and the nature of interaction effects.
This design allows the investigator to determine the strength of the independent effects of the intervention and the strength of the effect of pretesting on outcomes. If the groups that have been pretested show a testing effect, statistical procedures can be used to correct it, if necessary.
As you can see, the Solomon four-group design offers increased control and the possibility of understanding complex outcomes. Because it requires the addition of two groups, however, it is a costly, time-consuming alternative to the true-experimental design and infrequently used in health and human service inquiry.
Factorial Designs
These designs offer even more opportunities for multiple comparisons and complexities in analysis. In factorial designs, the investigator evaluates the effects of two or more independent variables (X1 and X2) or the effects of an intervention on different factors or levels of a sample or study variables. The interaction of these parts, as well as the direct relationship of one part to another, is examined.
The factorial design enables you to examine not only direct relationships but interactive relationships as well. You can determine the combined effects of two or more variables that may be quite different from each direct effect. For example, you may want to examine whether the less healthy exerciser scores higher on a life satisfaction assessment than the less healthy nonexerciser. To evaluate statistically all the possible configurations in this design, you need to have a large sample size to ensure that each cell or block (Figure 9-1) contains an adequate number of scores for analysis.
Of course, you can develop more complex factorial designs in which both independent and dependent variables have more than two values or levels. With more complexity, however, increasingly large sample sizes are necessary to ensure sufficient numbers in each variation to permit analysis.
Counterbalance Designs
When more than one intervention is being tested and when the order of participation is manipulated, counterbalance designs are often used. This design allows the investigator to determine the combined effects of two or more interventions and the effect of order on study outcomes. Although there are many variations, the basic design (with random sample selection) is as follows:

Again, lower case r would denote random assignment and would still qualify for this true-experimental design.
Note the subscript numbers on the independent variables. The reversal of the conditions is characteristic of counterbalance designs in which both groups experience both conditions but in different orders. This reversal is called “crossover.” In a crossover study, one group is assigned to the experimental group first and to the control condition later; the reverse order is assigned for the other group. Measurements occur before the first set of conditions, before the second set, and after the experiment. Such a study allows you to eliminate the threats to internal validity caused by the interaction of the experimental variable and other aspects of the study.
True-Experimental Design Summary
True-experimental designs must contain three essential characteristics: random assignment, control group, and manipulation. The classic true experiment and its variations all contain these elements and thus are capable of producing knowledge about causal relationships among independent and dependent variables. The four design strategies in the true-experimental classification are appropriate for an experimental-type Level 3 research question in which the intent is to predict and reveal a cause. Each true-experimental-type design controls the influences of the basic threats to internal validity and theoretically eliminates unwanted or extraneous phenomena that can confound a causal study and invalidate causal claims. The three criteria for using a true-experimental design or its variation are (1) sufficient theory to examine causality, (2) a Level 3 causal question, and (3) conditions and ethics that permit randomization, use of a control group, and manipulation.
Developing even the most straightforward experimental design, such as the two-group randomized trial, involves many other considerations. It is important to consult with a statistician about the possible approaches to randomization. A statistician can help you determine the best approach to randomizing subjects based on your study design and the basic characteristics of the subjects that you plan to enroll. There are many ways to randomize to ensure that subjects are assigned to groups by chance. In complex designs or large clinical trials, a statistician is usually responsible for setting up a randomization scheme and placing group assignments in sealed, opaque envelopes so that the investigators or research team members cannot influence the group assignment.
Quasi-experimental designs
Although true experiments have been upheld as the ideal or prototype in research, such designs may not be appropriate for many reasons, as we have indicated throughout this book. First, in health and human service research, it may not be possible, appropriate, or ethical to use randomization or to manipulate the introduction and withholding of an experimental intervention. Second, and perhaps most important, all inquiries do not ask causal questions, and thus the experimental design is not appropriate for questions that do not seek answers about cause-and-effect relationships. So what can you do when it is not possible or appropriate to use randomization, manipulation, or a control group?
You can select other design options that do not contain the three elements of true experimentation to generate valuable knowledge. Even though the language of the experimental-type tradition implies that these designs are “missing something,” we suggest that these designs are not “inferior” but rather that they produce different knowledge, interpretations, and uses than true experimentation.
As in all design decisions, the decision to use quasi-experimental designs should be based on the level of theory, type of research question asked, and constraints of the research environment. Cook and Campbell, who wrote the seminal classical work on quasi-experimentation, defined these designs as:
experiments that have treatments, outcome measures, and experimental units, but do not use random assignment to create comparison from which treatment-caused change is inferred. Instead, the comparisons depend on nonequivalent groups that differ from each other in many ways other than the presence of the treatment whose effects are being tested.6
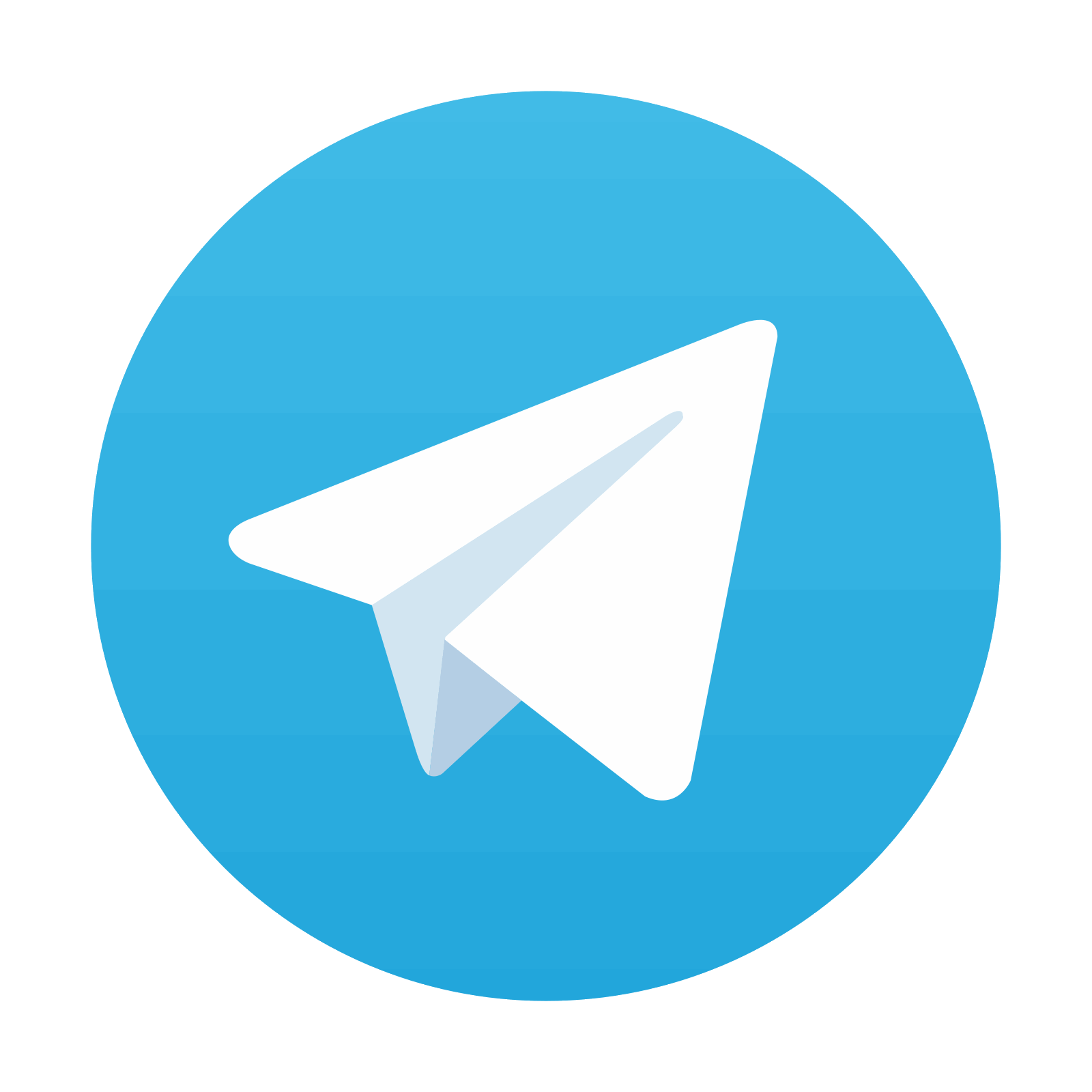
Stay updated, free articles. Join our Telegram channel

Full access? Get Clinical Tree
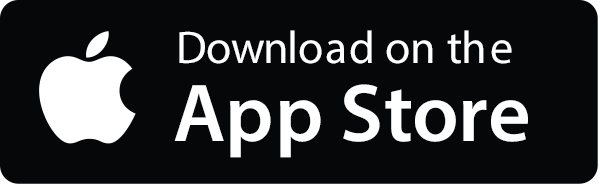
