Measurement in Experimental-Type Research
Key Terms
Continuous variable
Discrete variable
Guttman scale
Interval
Level of measurement
Likert-type scale
Measurement
Nominal
Ordinal
Proxy
Random error
Ratio
Reliability
Scales
Semantic differential scale
Systematic error
Validity
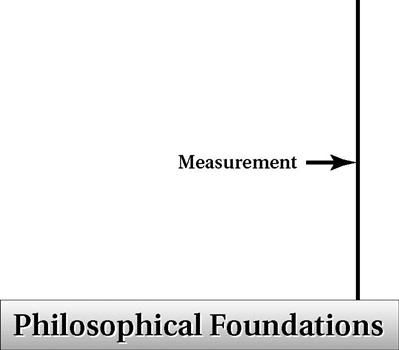
Think about the nature and scope of experimental-type designs. What would you say is the purpose of data collection using these research designs? This is the question that is addressed in this chapter.
In experimental-type designs, the purpose of data collection is to learn about an “objective reality.” In this tradition, the investigator is viewed as a separate entity from that which is being studied, and an attempt is made to ensure that the researcher’s own perspective and biases are not interjected in the conduct of the research. There must be strict adherence to a data collection protocol. A “protocol” refers to a series of procedures and techniques designed to remove the influence of the investigator from the data collection process and ensure a nonbiased and uniform approach to obtaining information. These data are made more “objective” (unbiased, a singular reality known by scientific method) by the assignment of numerical values, which are submitted to statistical procedures to test relationships, hypotheses, and population descriptors. These descriptions are viewed as representing objective reality. Therefore, in experimental-type research, the process of quantifying information or measurement is a primary concern. The investigator must develop instruments that are reliable and valid or have a degree of correspondence to an objective world or truth.
In this chapter, we examine the measurement process and concepts such as reliability and validity that are critical to the experimental-type research tradition.
Measurement process
What is measurement? In research, measurement has a precise meaning. Broadly speaking, measurement can be defined as the translation of observations into numerical values or numbers. It is a vital action process in experimental-type research that links the researcher’s abstractions or theoretical concepts to concrete variables that can be empirically or objectively examined. In experimental-type research, concepts must be made operational; in other words, they must be put into a format such as a questionnaire or interview schedule that permits structured, controlled observation and measurement. The measurement process involves a number of steps that include both conceptual and operational considerations (Figure 16-1).
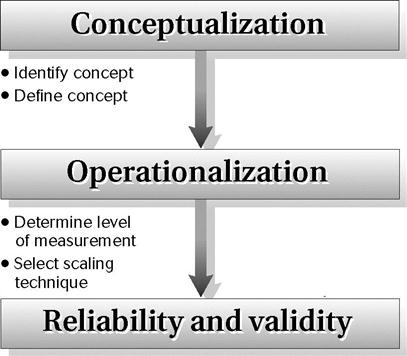
The first step in measurement involves conceptual work by the researcher. The researcher must identify and define what is to be measured. Although this may seem obvious, it is often a difficult initial first step that involves asking, “How will I conceptualize the phenomenon I want to study?” or “How will I define these concepts in words?” Consider such basic concepts as attitude, depression, anxiety, self-mastery, function, disability, wellness, quality of life, or adaptation. Each has been defined in many ways in the research literature. After the researcher identifies a concept, such as “anxiety” or “depression,” the literature must be thoroughly reviewed to decide on an appropriate definition.
The second step in measurement involves developing an operational definition of the concept. This operational step involves asking, “What kind of an indicator will I use as a gauge of this concept?” or “How will I classify and quantify what I observe?” A “measure” is an empirical representation of an underlying concept. Because, by definition, a “concept” is never directly observable, the strength of the relationship between an “indicator” and an underlying concept is critical. This relationship is referred to as “validity.” In other words, does the measurement measure what it aims to measure? Also, it is important for the indicator to measure consistently the underlying concept. The consistency of a measure is referred to as its “reliability.” The greater the reliability and validity, the more desirable is the instrument. Reliability and validity are two fundamental properties of indicators. Researchers engage in major efforts to evaluate the strength of reliability and validity to determine the desirability and value of a measure, as discussed later.
Information gathering in experimental-type research, an important action process, must begin with identifying key concepts. This step is followed by developing conceptual (lexical) and operational definitions of these concepts. On the basis of these definitions, specific indicators (scales, questionnaires, rating forms) are specified or developed. These indicators represent the source of information or data that will be collected.
Levels of measurement
The first step in developing an indicator involves specifying how a variable will be operationalized. This action process involves determining the numerical level at which the variable will be measured. The level of measurement refers to the properties and meaning of the number assigned to an observation. There are four levels of measurement: nominal, ordinal, interval, and ratio. Each level of measurement leads to different types of manipulations. That is, the way in which a variable is measured will have a direct bearing on the type of statistical analysis that can be performed. The decision regarding the level of measurement of a variable is thus a critical component of the measurement process. It is important to understand that the way in which you measure a particular concept dictates the type of analytical approach you will be able to pursue.
The level of measurement, whether you use age categories or the full range of possible ages measured in years, will determine the types of analytical approaches you can use, as you will learn here and in Chapter 19.
Several principles are used to determine the level of measurement of a “variable.” The first principle is that every variable must have two qualities. The first quality is that a variable must be exhaustive of every possible observation; that is, the variable should be able to classify every observation in terms of one or more of its attributes.
The second quality of a variable is that the attributes or categories must be mutually exclusive.
The second principle in determining a variable’s level of measurement is that variables can be characterized as being either discrete or continuous. A discrete variable is one with a finite number of distinct values. Again, gender is a good example of a discrete variable. Gender, as classically defined, has either a male or a female value. There is no “in-between” category. A continuous variable, in contrast, has an infinite number of values.1,2 Age (measured in years) and height (measured metrically or in feet and inches) are two examples of continuous variables in that they can be measured along a numerical continuum. It is possible to measure a continuous variable using discrete categories, such as the classification of the age variable as young, middle-aged, or old or the height variable as tall, medium, or short.
Discrete and continuous structures represent the natural characteristics of a variable. These characteristics have a direct bearing on the level of measurement that can be applied. We now examine each level in greater detail.
Nominal
Measuring gender as female or male represents the most basic, simplest, or lowest level of measurement, which is called nominal. This level involves classifying observations into mutually exclusive categories. Nominal means “name”; therefore, at this level of measurement, numbers are used, in essence, to name attributes of a variable. This level merely names or labels attributes, and these attributes are not ordered in any particular way.
Variables at the nominal level are discrete. For example, we may classify individuals according to their political or religious affiliation, gender, or ethnicity. In each of these examples, membership in one category excludes membership in another, but there is no order to the categories. One category is not higher or lower than another. Box 16-1 provides examples of mutually exclusive categories.
For data analysis, the researcher assigns a numerical value to each nominal category. For example, “male” may be assigned a value of 1, whereas “female” may be assigned a value of 2. The assignment of numbers is purely arbitrary, and no mathematical functions or assumptions of magnitude or ranking are implied or can be performed. Many survey analyses, conducted by telephone, mail, or face-to-face, use nominal level questions. The intent of these surveys is to describe the distribution of responses along these discrete categories. This level of measurement therefore is also referred to as “categorical” because the assignment of a nominal number denotes category membership.
Ordinal
The next level of measurement is ordinal. This level involves the ranking of phenomena. Ordinal means “order” and thus can be remembered as the numerical value that assigns an order to a set of observations. Variables that are discrete and conceptualized as having an inherent order at the theoretical level can be operationalized in a rank-order format. Variables operationalized at the ordinal level have the same properties as nominal categories in that each category is mutually exclusive of the other. In addition, ordinal measures have a fixed order so that the researcher can rank one category higher or lower than another.
The assignment of a numerical value is symbolic and arbitrary, as in the case of nominal variables, because the distance or spacing between each category is not numerically equivalent. However, the numbers imply magnitude; that is, one is greater than the other. Because there are no equal intervals between ordinal numbers, mathematical functions such as adding, subtracting, dividing, and multiplying cannot be performed. The researcher can merely state that one category is higher or lower, stronger or weaker, or greater or lesser.
Many scales useful to health and human service researchers are composed of variables measured at the ordinal level. For example, the concept of self-rated health is ranked from 1 = very poor to 5 = excellent. Although the numbers imply a state of being in which 5 is greater than 1, it is not possible to say how much greater the distance is between responses. Also, the difference in magnitude between 5 (excellent) to 4 (very good) may be perceived differently from person to person. Thus, you must be careful not to make any assumptions about the degree of difference between numerical values.
We want to raise a caution here to remind you that ordinal data must denote mutually exclusive phenomena. A common mistake is the inclusion of the extreme points of an interval in more than one category. For example, let’s say a questionnaire asks us about our age using categories as 1–10, 10–20, 20–30, and so on. What category do you check if you are 10, 20, or 30 years old? These numbers incorrectly appear in two categories. The investigator should have specified mutually exclusive intervals, such as 1–10, 11–20, 21–30, and so on.
Interval
The next level of measurement shares the characteristics of ordinal and nominal measures but also has the characteristic of equal spacing between categories. This level of measurement indicates how much categories differ. Interval measures are continuous variables in which the 0 point is arbitrary. Although interval measures are a higher order than ordinal and nominal measures, the absence of a true 0 point does not allow statements to be made concerning ratios. However, the equidistance between points allows the researcher to say that the difference between scores of 50 and 70 is equivalent to the difference between scores of 20 and 40.
In the social and behavioral sciences, there is considerable debate as to whether behavioral scales represent interval levels of measurement. Typically, such scales have a Likert-type response format in which a study participant responds to one of four to seven categories, such as strongly agree, agree, uncertain, disagree, or strongly disagree. Researchers who accept this type of scaling as an interval measure argue that the distance between “strongly agree” and “agree” is equivalent to the distance between “disagree” and “strongly disagree.” Others argue that there is no empirical justification for making this assumption and that the data generated should be considered ordinal. In the actual practice of research, many investigators assume such scales are at the interval level to use more sophisticated and powerful statistical procedures that are only possible with interval and ratio data. You should be aware that this issue continues to be controversial among experimental-type researchers. If you choose this type of scaling, think about the most purposive assignment of numeric levels to your response set.
Ratio
Ratio measures represent the highest level of measurement. Such measures have all the characteristics of the previous levels and, in addition, have an absolute 0 point. Income is an example of a ratio measure. Instead of classifying income into ordinal categories, as in the previous example, it can be described in terms of dollars. Income is a ratio measurement because someone can have an income of 0, and we can say that an income of $40,000 is twice as high as an income of $20,000.
Determining Appropriate Level
Table 16-1 summarizes the characteristics of each level of measurement.
TABLE 16-1
Characteristics of Experimental-Type Levels of Measurement
Level of Measurement | ||||
Characteristic | Nominal | Ordinal | Interval | Ratio |
Mutually exclusive categories | X | X | X | X |
Fixed ordering | X | X | X | |
Equal spacing | X | X | ||
Absolute 0 | X |
Experimental-type researchers usually strive to measure a variable at its highest possible level. However, the level of measurement also reflects the researcher’s concepts. If political affiliation, gender, and ethnicity are the concepts of interest, for example, nominal measurement may be the most appropriate level because magnitude does not typically apply to these concepts.
Measurement scales
Now that we have discussed the properties of numbers, let us examine the different methods by which data are collected and transformed into numbers in experimental-type research.
Scales are tools for the quantitative measurement of the degree to which individuals possess a specific attribute or trait. Box 16-2 lists examples of scales frequently used by health and human service professionals in research.
Scaling techniques measure the extent to which respondents possess an attribute or personal characteristic. Experimental-type researchers use three primary scaling formats: Likert approach to scales, Guttman scales, and semantic differential scales.10 Each has its merits and disadvantages. The researcher who is developing a scale needs to make basic formatting decisions about response-set structure, whereas the researcher selecting an existing scale must evaluate the format of the measure for his or her project.
Likert-Type Scale
In the Likert-type scale, the researcher develops a series of items (usually between 10 and 20) worded favorably and unfavorably regarding the underlying construct that is to be assessed. Respondents indicate a level of agreement or disagreement with each statement by selecting one of several response alternatives (usually five to seven). Researchers may combine responses to the questions to obtain a summated score, examine each item separately, or summate scores on specific groups of items to create subindices. In developing a Likert-type format, the researcher must decide how many response categories to allow and whether the categories should be even or odd in number. Even choices force a positive or negative response, whereas odd numbers allow the respondent to select a neutral or middle response.
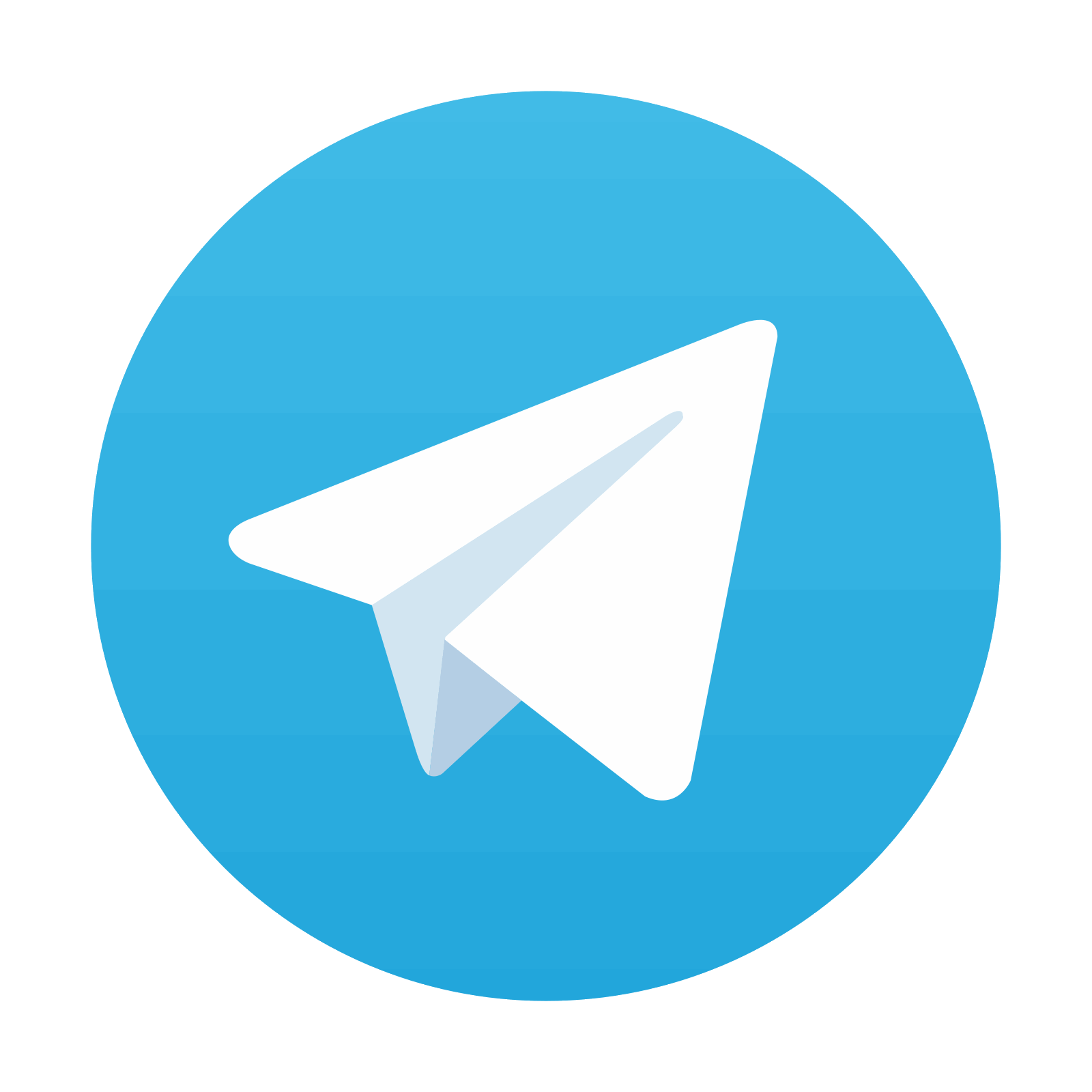
Stay updated, free articles. Join our Telegram channel

Full access? Get Clinical Tree
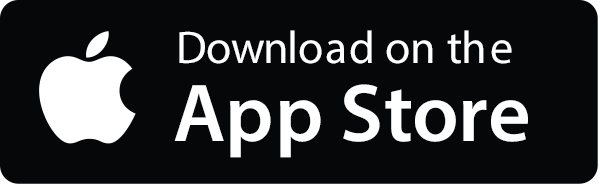
