Discuss alternative approaches to integrating research evidence and advantages to using systematic methods
Describe key decisions and steps in doing a meta-analysis and metasynthesis
Critique key aspects of a written systematic review
Define new terms in the chapter
Key Terms
Effect size (ES)
Forest plot
Frequency effect size
Intensity effect size
Manifest effect size
Meta-analysis
Meta-ethnography
Meta-summary
Metasynthesis
Primary study
Publication bias
Statistical heterogeneity
Subgroup analysis
Systematic review
In Chapter 7, we described major steps in conducting a literature review. This chapter also discusses reviews of existing evidence but focuses on systematic reviews, especially those in the form of meta-analyses and metasyntheses. Systematic reviews, a cornerstone of evidence-based practice (EBP), are inquiries that follow many of the same rules as those for primary studies, i.e., original research investigations. This chapter provides guidance in helping you to understand and evaluate systematic research integration.
RESEARCH INTEGRATION AND SYNTHESIS
A systematic review integrates research evidence about a specific research question using careful sampling and data collection procedures that are spelled out in advance. The review process is disciplined and transparent so that readers of a systematic review can assess the integrity of the conclusions.
Twenty years ago, systematic reviews usually involved narrative integration, using nonstatistical methods to synthesize research findings. Narrative systematic reviews continue to be published, but meta-analytic techniques that use statistical integration are widely used. Most reviews in the Cochrane Collaboration, for example, are meta-analyses. Statistical integration, however, is sometimes inappropriate, as we shall see.
Qualitative researchers have also developed techniques to integrate findings across studies. Many terms exist for such endeavors (e.g., meta-study, meta-ethnography), but the one that has emerged as the top term is metasynthesis.
The field of research integration is expanding steadily. This chapter provides a brief introduction to this important and complex topic.
META-ANALYSIS
Meta-analyses of randomized controlled trials (RCTs) are at the pinnacle of traditional evidence hierarchies for Therapy questions (see Fig. 2.1). The essence of a meta-analysis is that findings from each study are used to compute a common index, an effect size. Effect size values are averaged across studies, yielding information about the relationship between variables across multiple studies.
Advantages of Meta-Analyses
Meta-analysis offers a simple advantage as an integration method: objectivity. It is difficult to draw objective conclusions about a body of evidence using narrative methods when results are inconsistent, as they often are. Narrative reviewers make subjective decisions about how much weight to give findings from different studies, and so different reviewers may reach different conclusions in reviewing the same studies. Meta-analysts make decisions that are explicit and open to scrutiny. The integration itself also is objective because it uses statistical formulas. Readers of a meta-analysis can be confident that another analyst using the same data set and analytic decisions would come to the same conclusions.
Another advantage of meta-analysis concerns power, i.e., the probability of detecting a true relationship between variables (see Chapter 14). By combining effects across multiple studies, power is increased. In a meta-analysis, it is possible to conclude that a relationship is real (e.g., an intervention is effective), even when several small studies yielded nonsignificant findings. In a narrative review, 10 nonsignificant findings would almost surely be interpreted as lack of evidence of a true effect, which could be the wrong conclusion.
Despite these advantages, meta-analysis is not always appropriate. Indiscriminate use has led critics to warn against potential abuses.
Criteria for Using Meta-Analytic Techniques in a Systematic Review
Reviewers need to decide whether statistical integration is suitable. A basic criterion is that the research question should be nearly identical across studies. This means that the independent and dependent variables, and the study populations, are sufficiently similar to merit integration. The variables may be operationalized differently to be sure. Nurse-led interventions to promote healthy diets among diabetics could be a 4-week clinic-based program in one study and a 6-week home-based intervention in another, for example. However, a study of the effects of a 1-hour lecture to discourage eating “junk food” among overweight adolescents would be a poor candidate to include in this meta-analysis. This is frequently referred to as the “apples and oranges” or “fruit” problem. Meta-analyses should not be about fruit— i.e., a broad category—but rather about “apples,” or, even better, “Granny Smith apples.”
Another criterion concerns whether there is a sufficient knowledge base for statistical integration. If there are only a few studies or if all of the studies are weakly designed, it usually would not make sense to compute an “average” effect.
One other issue concerns the consistency of the evidence. When the same hypothesis has been tested in multiple studies and the results are highly conflicting, meta-analysis is likely not appropriate. As an extreme example, if half the studies testing an intervention found benefits for those in the intervention group, but the other half found benefits for the controls, it would be misleading to compute an average effect. In this situation, it would be better to do an in-depth narrative analysis of why the results are conflicting.
Example of inability to conduct a meta-analysis
Langbecker and Janda (2015) undertook a systematic review of interventions designed to improve information provision for adults with primary brain tumors. They had intended to undertake a meta-analysis, “ . . . but the heterogeneity in intervention types, outcomes, and study designs means that the data were unsuitable for this” (p. 3).
Steps in a Meta-Analysis
We begin by describing major steps in a meta-analysis so that you can understand the decisions a meta-analyst makes—decisions that affect the quality of the review and need to be evaluated.
Problem Formulation
A systematic review begins with a problem statement and a research question or hypothesis. Questions for a meta-analysis are usually narrow, focusing, for example, on a particular type of intervention and specific outcomes. The careful definition of key constructs is critical for deciding whether a primary study qualifies for the synthesis.
Example of a question from a meta-analysis
Dal Molin and colleagues (2014) conducted a meta-analysis that addressed the question of whether heparin was more effective than other solutions in catheter flushing among adult patients with central venous catheters. The clinical question was established using the PICO framework (see Chapter 2). The Population was patients using central venous catheters. The Intervention was use of heparin in the flushing, and the Comparison was other solutions (e.g., normal saline). Outcomes included obstructions, infections, and other complications.
A strategy that is gaining momentum is to undertake a scoping review to refine the specific question for a systematic review. A scoping review is a preliminary investigation that clarifies the range and nature of the evidence base, using flexible procedures. Such scoping reviews can suggest strategies for a full systematic review and can also indicate whether statistical integration (a meta-analysis) is feasible.
The Design of a Meta-Analysis
Sampling is an important design issue. In a systematic review, the sample consists of the primary studies that have addressed the research question. The eligibility criteria must be stated. Substantively, the criteria specify the population (P) and the variables (I, C, and O). For example, if the reviewer is integrating findings about the effectiveness of an intervention, which outcomes must the researchers have studied? With regard to the population, will (for example) certain age groups be excluded? The criteria might also specify that only studies that used a randomized design will be included. On practical grounds, reports not written in English might be excluded. Another decision is whether to include both published and unpublished reports.
Example of sampling criteria
Liao and coresearchers (2016) did a meta-analysis of the effects of massage on blood pressure in hypertensive patients. Primary studies had to be RCTs involving patients with hypertension or prehypertension. The intervention had to involve massage therapy, compared to a control condition (e.g., placebo, usual care, no treatment). To be included, the studies had to have blood pressure measurements as the primary outcome. Trials were excluded if they were not reported in English.
Researchers sometimes use study quality as a sampling criterion. Screening out studies of lower quality can occur indirectly if the meta-analyst excludes studies that did not use a randomized design. More directly, each potential primary study can be rated for quality and excluded if the quality score falls below a threshold. Alternatives to dealing with study quality are discussed in a later section. Suffice it to say, however, that evaluations of study quality are part of the integration process, and so analysts need to decide how to assess quality and what to do with assessment information.
Another design issue concerns the statistical heterogeneity of results in the primary studies. For each study, meta-analysts compute an index to summarize the strength of relationship between an independent variable and a dependent variable. Just as there is inevitably variation within studies (not all people in a study have identical scores on outcomes), so there is inevitably variation in effects across studies. If the results are highly variable (e.g., results are conflicting across studies), a meta-analysis may be inappropriate. But if the results are moderately variable, researchers can explore why this might be so. For example, the effects of an intervention might be systematically different for men and women. Researchers often plan to explore such differences during the design phase of the project.
The Search for Evidence in the Literature
Many standard methods of searching the literature were described in Chapter 7. Reviewers must decide whether their review will cover published and unpublished findings. There is some disagreement about whether reviewers should limit their sample to published studies or should cast as wide a net as possible and include grey literature—that is, studies with a more limited distribution, such as dissertations or unpublished reports. Some people restrict their sample to reports in peer-reviewed journals, arguing that the peer review system is a tried-and-true screen for findings worthy of consideration as evidence.
Excluding nonpublished findings, however, runs the risk of biased results. Publication bias is the tendency for published studies to systematically overrepresent statistically significant findings. This bias (sometimes called the bias against the null hypothesis) is widespread: Authors may refrain from submitting manuscripts with nonsignificant results, reviewers and editors tend to reject such reports when they are submitted, and users of evidence may ignore the findings if they are published. The exclusion of grey literature in a meta-analysis can lead to the overestimation of effects.
Meta-analysts can use various search strategies to locate grey literature in addition to the usual methods for a literature review. These include contacting key researchers in the field to see if they have done studies (or know of studies) that have not been published, hand searching the tables of contents of relevant journals, and reviewing abstracts from conference proceedings.
![]() | TIP There are statistical procedures to detect and correct for publication biases, but opinions vary about their utility. A brief explanation of methods for assessing publication bias is included in the supplement to this chapter on the |
Example of a search strategy from a systematic review
Al-Mallah and colleagues (2016) did a meta-analysis of the effect of nurse-led clinics on the mortality and morbidity of patients with cardiovascular diseases. Their search strategy included a search of eight bibliographic databases, scrutiny of the bibliographies of all identified studies, and a hand search of relevant specialized journals.
Evaluations of Study Quality
In systematic reviews, the evidence from primary studies needs to be evaluated to assess how much confidence to place in the findings. Rigorous studies should be given more weight than weaker ones in coming to conclusions about a body of evidence. In meta-analyses, evaluations of study quality sometimes involve overall ratings of evidence quality on a multi-item scale. Hundreds of rating scales exist, but the use of such scales has been criticized. Quality criteria vary from instrument to instrument, and the result is that study quality can be rated differently with different assessment scales—or by different raters using the same scale. Also, when an overall scale score is used, the meaning of the scores often are not transparent to users of the review.
The Cochrane Handbook (Higgins & Green, 2008) recommends a domain-based evaluation, that is, a component approach, as opposed to a scale approach. Individual design elements are coded separately for each study. So, for example, a researcher might code for whether randomization was used, whether participants were blinded, the extent of attrition from the study, and so on.
Quality assessments of primary studies, regardless of approach, should be done by two or more qualified individuals. If there are disagreements between the raters, there should be a discussion until a consensus has been reached or until another rater helps to resolve the difference.
Example of a quality assessment
Bryanton, Beck (one of this book’s authors), and Montelpare (2013) completed a Cochrane review of RCTs testing the effects of structured postnatal education for parents. They used the Cochrane domain approach to capture elements of trial quality. The first two authors completed assessments, and disagreements were resolved by discussion.
Extraction and Encoding of Data for Analysis
The next step in a meta-analysis is to extract and record relevant information about the findings, methods, and study characteristics. The goal is to create a data set amenable to statistical analysis.
Basic source information must be recorded (e.g., year of publication, country where data were collected). Important methodologic features include sample size, whether participants were randomized to treatments, whether blinding was used, rates of attrition, and length of follow-up. Characteristics of participants must be encoded as well (e.g., their mean age). Finally, information about findings must be extracted. Reviewers must either calculate effect sizes (discussed in the next section) or must record sufficient statistical information that computer software can compute them.
As with other decisions, extraction and coding of information should be completed by two or more people, at least for a portion of the studies in the sample. This allows for an assessment of interrater reliability, which should be sufficiently high to persuade readers of the review that the data are accurate.
Example of intercoder agreement
Chiu and colleagues (2016) conducted a meta-analysis of the effects of acupuncture on menopause-related symptoms in breast cancer survivors. Two researchers independently extracted data from seven RCTs using a standard form. Disagreements were resolved until consensus was reached.
Calculation of Effects
Meta-analyses depend on the calculation of an effect size (ES) index that encapsulates in a single number the relationship between the independent and outcome variable in each study. Effects are captured differently depending on the measurement level of variables. The three most common scenarios for meta-analysis involve comparisons of two groups such as an intervention versus a control group on a continuous outcome (e.g., blood pressure), comparisons of two groups on a dichotomous outcome (e.g., stopped smoking vs. continued smoking), or correlations between two continuous variables (e.g., between blood pressure and anxiety scores).
The first scenario, comparison of two group means, is especially common. When the outcomes across studies are on identical scales (e.g., all outcomes are measures of weight in pounds), the effect is captured by simply subtracting the mean for one group from the mean for the other. For example, if the mean postintervention weight in an intervention group were 182.0 pounds and that for a control group were 194.0 pounds, the effect size would be −8.0. Typically, however, outcomes are measured on different scales (e.g., a scale of 0–10 or 0–100 to measure pain). Mean differences across studies cannot in such situations be combined and averaged; researchers need an index that is neutral to the original metric. Cohen’s d, the effect size index most often used, transforms all effects into standard deviation units. If d were computed to be .50, it means that the group mean for one group was one-half a standard deviation higher than the mean for the other group—regardless of the original measurement scale.
![]() | TIP The term effect size is widely used for d in the nursing literature, but the term usually used for Cochrane reviews is standardized mean difference or SMD. |
When the outcomes in the primary studies are dichotomies, meta-analysts usually use the odds ratio (OR) or the relative risk (RR) index as the ES statistic. In nonexperimental studies, a common effect size statistic is Pearson’s r, which indicates the magnitude and direction of effect.
Data Analysis
After an effect size is computed for each study, as just described, a pooled effect estimate is computed as a weighted average of the individual effects. The bigger the weight given to any study, the more that study will contribute to the weighted average. A widely used approach is to give more weight to studies with larger samples.
An important decision concerns how to deal with the heterogeneity of findings—i.e., differences from one study to another in the magnitude and direction of effects. Statistical heterogeneity should be formally tested, and meta-analysts should report their results.
Visual inspection of heterogeneity usually relies on the construction of forest plots, which are often included in meta-analytic reports. A forest plot graphs the effect size for each study, together with the 95% confidence interval (CI) around each estimate. Figure 18.1 illustrates forest plots for situations in which there is low heterogeneity (panel A) and high heterogeneity (panel B) for five studies. In panel A, all five effect size estimates (here, odds ratios) favor the intervention group. The CI information indicates the intervention effect is statistically significant (does not encompass 1.0) for studies 2, 4, and 5. In panel B, by contrast, the results are “all over the map.” Two studies favor the control group at significant levels (studies 1 and 5), and two favor the treatment group (studies 2 and 4). Meta-analysis is not appropriate for the situation in panel B.
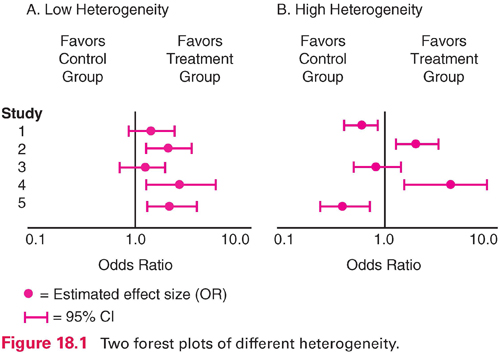
![]() | TIP Heterogeneity affects not only whether a meta-analysis is appropriate but also which statistical model should be used in the analysis. When findings are similar, the researchers may use a fixed effects model. When results are more varied, it is better to use a random effects model. ![]() Stay updated, free articles. Join our Telegram channel![]() Full access? Get Clinical Tree![]() ![]() ![]() |