Chapter 19. Single-case Studies
Rob Newell
▪ Introduction – what are single-case experiments?
▪ What are single-case experiments for?
▪ What are the basic assumptions of single-case experiments?
▪ How are single-case experiments designed?
▪ How are single-case experiments analysed?
▪ Conclusion
Introduction – what are single-case experiments?
The researcher using single-case experimental methods applies the same ideas about control of variability as in traditional group experiments, attempting to control for bias which results both from subjects and from design. Thus, the single-case experiment is an attempt to examine cause–effect relationships in a single individual with the same amount of methodological rigour as in group experiments.
From the above, it should be immediately apparent that the SCED is a quantitative approach to research. Indeed, quantification is a defining characteristic of the approach. It is the crucial distinction between the SCED and the traditional case study.
Similarly, the randomised controlled trial (RCT) is itself problematic. RCTs are the gold standard in terms of the investigation of cause and effect because of their control of bias, but they have drawbacks. They cannot be swiftly mounted, since they require considerable planning and generally take a long time to complete. They typically require large numbers of participants before meaningful conclusions can be drawn. Finally, they are probabilistic, and so cannot offer definitive guidance to the clinician in a specific situation with a specific patient (Newell & Burnard 2006). Whilst none of these difficulties is insurmountable, the debate around the use of the RCT, and around evidence-based practice based on RCTs, has continued (Newell 2003), and is, if anything, more current today than when SCEDs first came to prominence.
What are single-case experiments for?
The SCED is firstly a way of investigating the effectiveness (or otherwise!) of our interventions with patients, using a credible research approach, during our everyday clinical practice (Newell 1992). In this sense, it can be useful in three main ways. First, we can monitor patient progress reliably. Second, we can monitor the effectiveness of a known treatment approach and, if necessary, assess our own competence in using it and identify training needs. Finally, we can start to develop innovative treatments, based on theory and clinical experience, applying them in novel situations with clients for whom no evidence base for intervention currently exists.
The SCED may also be an extension of this clinical use, where we can use experimental control to build up a systematic picture of a patient’s characteristics or difficulties. This approach is rarely used in nursing, but is a mainstay of psychology, particularly where a patient presents with very unusual attributes. In such situations, group experiments would be almost impossible to mount, since sufficient numbers would simply not exist. This use of SCEDs is perhaps the most similar in intent to the traditional case study, which often seeks to document unusual cases, but once again, differs because of the inclusion of systematic measurement and control. One very accessible example from the psychology literature is To See but Not to See (Humphreys & Riddoch 1987), which uses a series of experimental approaches to arrive at a systematic description of the processes which underlie a particular case of visual agnosia.
Finally, SCEDs are a powerful experiential learning tool. It is surprising that, whilst both experiential learning and the need for adequate education in research have increased in importance and prominence in nursing, the two issues are rarely put together. This is, perhaps, because experience in research is often hard to come by, particularly for the novice. Yet research is most definitely a craft skill for nurses, in the same way that learning to give a bed bath, an injection or a flexible sigmoidoscopy are essentially nursing craft skills. Divorcing the theory from the craft leads to a view of these skills which is often inappropriate. SCEDs provide an antidote to an overly theoretical perception of the research process because they allow the novice actually to engage in research, rather than reviewing it. Whilst some qualitative projects also allow this, they do not have the power to explore cause/effect relationships, leaving SCEDs as the only viable option for the novice wishing to learn about the systematic research of treatment effectiveness through experiential learning.
What are the basic assumptions of single-case experiments?
The first basic assumption is that it is possible to examine cause and effect meaningfully in a single case. This assumption leads to the insistence on proper experimental control which we described earlier in this chapter. Thus, unlike in the case study, the treatment, the desired outcome and the assumed relationship between them are specified before the study begins, and then adhered to throughout. In experimental terms, this is the equivalent of saying that the independent variable and dependent variable are adequately operationalised and an hypothesis is stated. For the remainder of this chapter, we will use the treatment of a person with multiple sclerosis as a case example. The patient has identified two problems which are particularly affecting her life – fatigue and pain. For the moment, we will consider just pain. The dependent variable will be scores on the Multidimensional Pain Inventory (MPI) (Kerns et al 1985); the independent variable will be relaxation exercises or no treatment; the hypothesis will be that the patient will report less pain when undertaking relaxation exercises than when having no treatment.
The second assumption of SCEDs is that the research design should be flexible in response to changing circumstances. This seems, of course, to contradict the first assumption, since this flexibility usually involves changing the nature of the independent variable (treatment). However, where treatment tactics are changed in response to varying clinical needs, these changes are explicitly acknowledged, and measurement is used to attempt to isolate the point at which treatment has changed, and maintain the integrity of the cause/effect chain. The general point, however, is that SCEDs are patient centred.
The third important assumption of SCEDs is the notion of generalisability. SCEDs are criticised for having poor external validity. One aspect of this is that it is argued that individual cases are unique and that this uniqueness prevents us from drawing any conclusions about the general effectiveness of a treatment for patients with similar problems. Proponents of SCEDs have sought to overcome this objection by invoking the idea of lawfulness. This idea was borrowed from natural sciences, principally by behaviourists in the 1940s and 1950s who sought to identify governing principles or laws of human behaviour which were similar in applicability to laws in the natural sciences, which govern such issues as the diffusion of gases or the rate of cooling of a liquid. The argument is that if human behaviour is lawful, a relationship which holds true in a single person will hold true for all other persons. Naturally, the greater the similarity between the person in whom the relationship was demonstrated and others, the better the law will hold, but this is regarded as being a matter of the test conditions under which the relationship was shown to exist, rather than a breach of the law supposedly governing that relationship.
How are single-case experiments designed?
Just as different group experimental designs seek to reduce different sources of bias, so do different single-case experimental designs. There are two different classes of SCED: AB designs and multiple baseline designs. Broadly speaking, these two classes of SCED correspond to repeated measures and independent groups designs in traditional group experimental designs (Newell & Burnard 2006). As we noted earlier in this chapter, SCEDs attempt to establish good internal validity. Their various designs represent different ways of dealing with threats to that internal validity. For the rest of this section, we will follow the patient with multiple sclerosis through a number of different design approaches which address threats to external validity while remaining flexible in response to her individual needs and allowing the nurse to try different interventions to meet these. The actual scores, however, are for illustrative purposes only, and should not be taken as endorsements of any particular treatment approach.
Case example: pain and fatigue in multiple sclerosis – 1: The AB design and its variants
In all AB designs, the A phase represents baseline measurement (a period of no intervention), whilst the B phase represents the introduction of an intervention.
The AB design
In this approach, the simplest way of systematically examining treatment outcomes, a period of baseline measurement is followed by introduction of the treatment, and is really little different from what goes on in clinical practice. We observe patient problems and respond. The crucial distinction, from a research point of view, is that both the observation and the response are quantified. As we can see from Figure 19.1, the patient has measured her perception of pain on a regular basis, both before the introduction of relaxation exercises and during a period when these exercises have been practised on a regular basis. From the graphs, we infer that improvement has occurred.
![]() |
Figure 19.1 The AB design. |
The ABA design
Tempting as it is to infer improvement from the graph in Figure 19.1, this inference is oversimple, since the AB design, like the before–after design in group experimental designs, is vulnerable to maturation effects (physical or psychological changes as a result of time) and extraneous environmental effects over time. These effects confound our ability to attribute causation to the independent variable in both SCEDs and repeated measures group designs, and have led in the past to inflated estimates of treatment effectiveness. In the case of the multiple sclerosis patient, the improvement in pain might have been caused by a change in medication or the fact that multiple sclerosis is variable in its course, rather than as a result of relaxation exercises. However, it is relatively simple to test these propositions by the introduction of a further A phase – a return to baseline, during which relaxation is not practised (Fig. 19.2). One shortcoming here is that, for some interventions, return to baseline is not possible. For example, if we offer information, it is, by and large, not possible to ask a person to forget consciously that information. However, if an intervention requires continuing input (as is often the case in long-term illness, for example), then a return to baseline is a powerful way of increasing our confidence in the internal validity of the study.
![]() |
Figure 19.2 The ABA design. |
The ABAB design
In the above example, the patient’s improvement has not been maintained following return to baseline. It is sometimes argued that it is unethical to use return to baseline for this reason, but this argument is difficult to sustain, since it is important that clinicians know whether a treatment is in fact the cause of changes in the patient’s status. If it is not, and the patient continues to undertake the intervention, that is a waste of their time and effort, and therefore is itself unethical. However, if the patient does not maintain gains during the return to baseline, this is evidence for the effectiveness of the intervention, and it would, in this case, be unethical to leave the patient at the return to baseline phase. In SCEDs, the design modification reflects what we would probably do in clinical practice – we reintroduce the treatment phase, resulting in an ABAB design (Fig. 19.3).
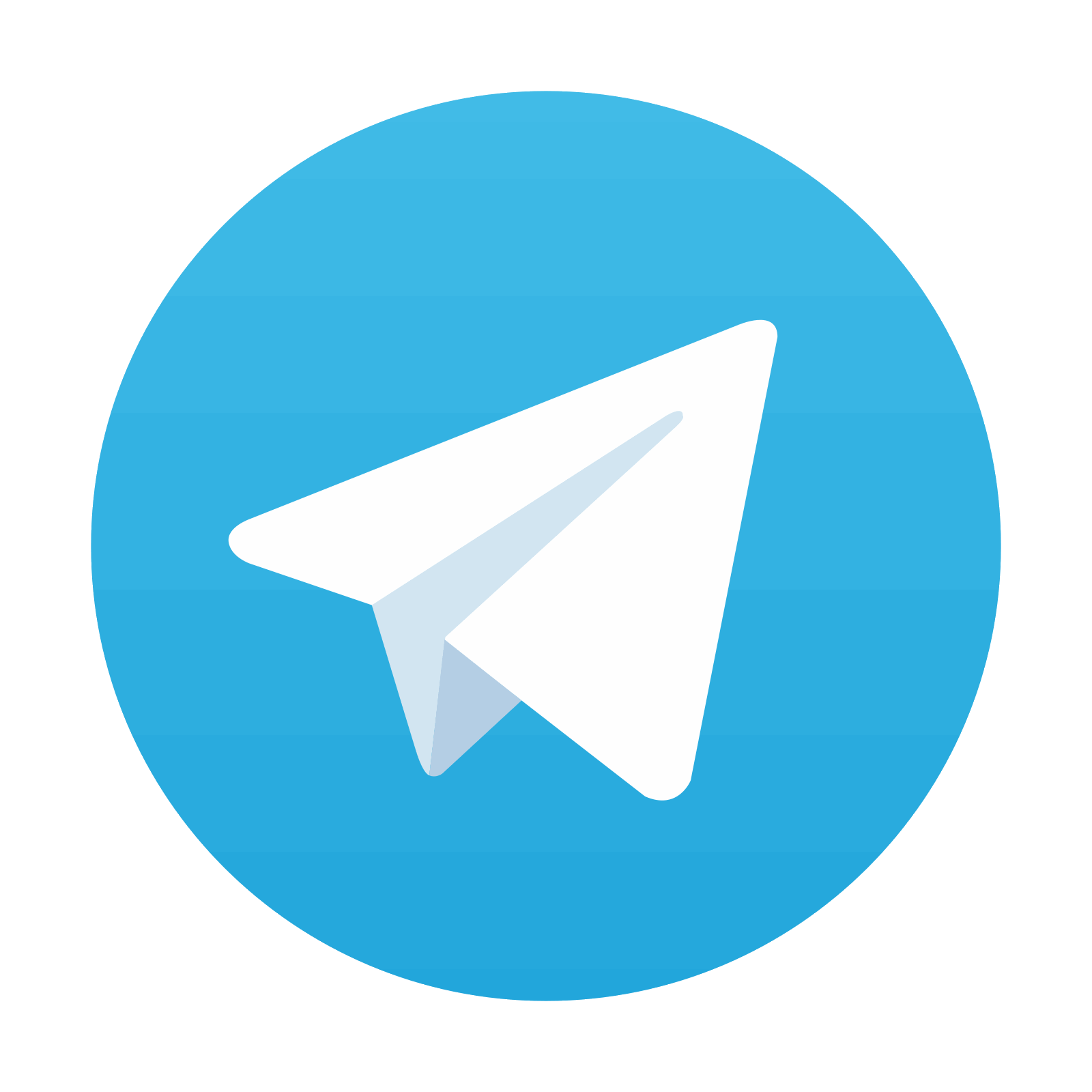
Stay updated, free articles. Join our Telegram channel

Full access? Get Clinical Tree
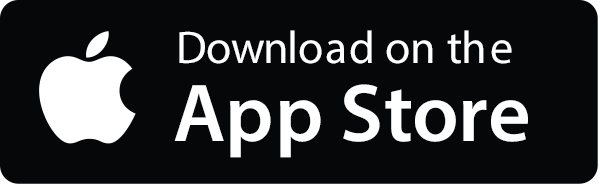

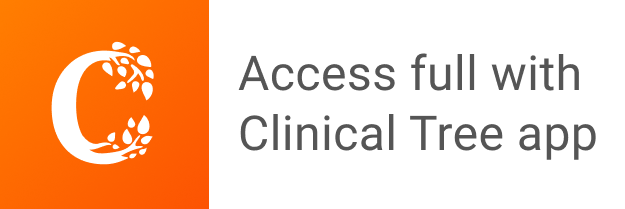