html xmlns=”http://www.w3.org/1999/xhtml”>
13
Sampling, Reliability and Validity Issues in Data Collection and Analysis
Key points
- Random sampling decreases the likelihood that members of a sample are different from its population.
- Stratification and cluster sampling both ensure adequate representation of population subgroups in a sample.
- Quota sampling and systematic sampling approximate to random sampling.
- Convenience sampling is the simplest form of sampling but is least likely to conform to its population.
- External validity refers to the applicability of a study to the real world.
- Population validity refers to the similarity between a study sample and its population.
- Ecological validity refers to the similarity between study conditions and procedures and the real world.
- Internal validity determines the confidence we can have in cause-effect relationships in a study.
- Reliability consists of two concepts: consistency and repeatability.
- Consistency implies that, if a phenomenon is unchanged, it will be measured as the same by several observers or by several measuring methods.
- Repeatability means that, if a phenomenon is unchanged, it will be measured as the same on several occasions.
Introduction
In Chapter 6, we noted how, in sampling, quantitative researchers were concerned with the notion of representativeness and how this might be established by adequacy of sampling. This chapter explores some of the techniques used principally by quantitative researchers to increase the likelihood that their sampling approaches will lead to generalis- able results. Other major issues which contribute to generalisability of a study are the reliability and validity of the ways in which data have been collected. We will introduce these later in this chapter, and they will be returned to in later chapters in the contexts of the types of research design they relate to.
Representativeness, fairness and random sampling
One important issue in establishing representativeness is the notion that each potential participant has a fair, equal chance of participating in a study. This is achieved principally by random sampling, which we touched on in Chapter 6. The most efficient way of creating a random sample is by the use of a computer-generated list of random numbers. Other methods such as putting numbers into a hat or tossing a coin are generally subject to human error or depart from true randomness for other reasons such as the fact that a small number of trials is rarely random.
Coin-toss experiment
Try tossing a coin 10 times, now.
Did you get 5 heads and 5 tails?
If so (possible but unlikely), try again?
Another 5 heads and 5 tails? We do not think so.
If you did not get 5 heads and 5 tails (likely), you probably do not think there is anything wrong with the coin – it is not heavier on one side, for example. This is because we all know, from our ordinary lives, that probabilities of this type take a long time to even out. The same thing applies in research situations, too. Yet, randomisation by coin-toss was for many years to be thought to be adequate.
In surveys of a defined population, the researcher uses computergenerated numbers to choose which members of that population to enter into the study as the eventual sample, whilst in randomised controlled trials (see Chapter 17), a broadly similar process is used to assign participants to one treatment rather than another. Again, the notion of fairness is at the root of the procedure. The researcher is concerned that all participants have a fair chance of being allocated to each of the possible treatments. In the first instance, representativeness is increased because random selection reduces the likelihood that members of the sample are different from the whole population. In the second, it is increased because randomness decreases the likelihood that participants in one treatment are different from those in the other(s). In this second case, we are able to generalise from the sample to the supposed population because we assume that results in a population if they were similarly randomised would be equivalent to those in the sample. As we shall see in Chapters 15, 16 and 17, there are numerous other issues which affect our ability to make such a generalisation with confidence, but adequate randomisation is a basic prerequisite for drawing conclusions about populations from samples.
Stratification
In random sampling, stratification is one way of increasing fairness and, by extension, representativeness. At first glance, it might seem that, having sampled randomly, no further effort is required to ensure either of these things. For extremely large samples, this may, indeed, be true. However, most studies, even those with considerable samples, are not actually large enough to ensure fairness of inclusion. This is because, in many instances, populations are heterogeneous and practically sized samples are not large enough to capture that diversity. An extreme example will make this obvious. Breast cancer is extremely rare in males (roughly 1% of new UK cases annually). To be representative, we should ideally wish to sample 1 male patient for every 99 females. If we randomly sampled breast cancer patients, it is very unlikely that we would achieve this because the smaller the probability of something occurring in a given population, the larger the sample needed to capture it. In stratification, this problem is overcome by identifying sample numbers in proportion to the existence of different subgroups in the total population, then sampling at the same rate from each of the strata. In our example, we would identify say 100 men and 9900 women, and sample at a rate of 10% from each. We would then end up with an eventual sample of 10 men and 990 women, accurately reflecting the population of breast cancer patients. This is obviously a very large sample, but still much smaller that we would require to represent the genders fairly through randomisation alone. Characteristics such as gender, age and ethnicity are routinely stratified for in large studies, and stratification can also be applied to the allocation of patients to treatments in comparison studies such as RCTs.
Cluster sampling
This method of sampling is very close to stratification in that it is a way of ensuring fair representation of particular groups. It is done by randomly choosing particular clusters of participants. These clusters are typically geographical areas, but could, for example, also be hospital wards or diagnostic groups. It usually works most fairly if some form of comparability between the clusters can be reasonably asserted. Participants are then randomly chosen from within each cluster, typically, in accordance with the comparative size of the clusters. Cluster sampling is sometimes referred to as multistage sampling because of this second random sampling procedure. It would be possible to further break down each cluster into sub-clusters before eventually assigning participants.
The essential difference between stratified sampling and cluster sampling is that in cluster sampling, the clusters are chosen randomly from a larger population of clusters. In stratified sampling, all theoretically possible clusters are included and sampled from.
Cluster randomisation is a variant of cluster sampling used in treatment comparison studies (typically, RCTs), in which a treatment is randomly assigned to a setting. All patients who are treated in that setting who enter the study receive that treatment.
In high-quality quantitative research, the above methods of sampling are the most common, and are referred to as methods of probability sampling, because the probability of a participant appearing in a sample is equal to their rates in the population from which that sample is drawn. There are, however, numerous methods of non-probability sampling. Some of these are particularly associated with qualitative research, and are described in Chapter 7. Others are used in both quantitative and qualitative research. In quantitative research, they are regarded as less robust than probability samples, because their general- isability is poorer, and quantitative research typically has the aim of drawing inferences about populations from samples.
Quota samples
Quota sampling is perhaps closest to a probability sample, in that considerable efforts may be made to ensure representativeness. Researchers decide on a range of sampling parameters (quota controls) which are relevant to their study (e.g. age, gender, qualification, ethnic background) and sample on the basis of these. The difference between quota sampling and stratified sampling is that the numbers in each quota eventually recruited as participants via quota sampling do not necessarily reflect the rates of people in the study population who possess the quota control characteristics (although they may do). Even where the quotas do contain numbers of participants reflective of base levels, they are not selected randomly. Confusingly, some books refer to quota sampling as a type of stratified sampling (or the reverse). We prefer to use the term stratification only for random samples, as we believe this avoids confusion.
Systematic samples
Systematic sampling once again attempts to introduce fairness into the selection process. It involves the use of fixed intervals in assigning participants to a study. Thus, every fifth person from a list might be included in the sample. It is sometimes suggested that, if the first person to be drawn from the list is selected at random, before starting to pick every fifth person, then systematic sampling is a method of probability sampling. However, there is a possibility of biased sampling in systematic sampling. In the event that some bias has entered into compiling the list from which participants are to be drawn, then random assignment of the first participant will not help with this. It simply means that the biased list starts in a random place. This weakness is not present in random sampling, and therefore, whilst acknowledged to be a reasonably robust way of assigning participants to a study, systematic sampling is likely always to be less robust than random sampling, particularly where a list contains particular order characteristics the researcher is not aware of.
Convenience/accidental samples
This is probably the weakest form of sampling. It is also the easiest to obtain, and therefore the one most often seen in small projects and student assignments. The amount of generalisability possible from such a sample is low because of the inherent weaknesses in the sampling procedure. Convenience samples (sometimes called accidental samples) are exactly what they say, samples of people gained in the way most convenient to the researcher. This may be as simple as sampling patients by taking the next 100 who walk through the clinic door and asking them to complete a questionnaire, or taking the books at my bedside as a sample of my reading habits. However, supposing we take our sample of patients on a Monday, and the clinic always sees its sickest patients on that day. Similarly, supposing I like to read undemanding detective stories at night because they help me sleep, but have bookcases stacked with plays, non-fiction works etc., which I read extensively at other times. In both these extreme cases, the poor gener- alisability of convenience samples is evident. The same principle (that circumstances may introduce bias into convenience sampling) is just as problematic in all convenience sampling. Balanced against this shortcoming, convenience samples are the easiest to obtain, and this may be a critical factor in student projects or small pilot studies.
Synopsis of sampling approaches in quantitative research
Random sampling is most likely to ensure that a sample is generally representative of its population.
In practice, stratification and cluster sampling refine random sampling in small samples to ensure adequate representation of subgroups.
Non-random quota sampling and systematic sampling approximate to random sampling in their representativeness.
Convenience sampling is weak because there is less likelihood of similarity between sample and population.
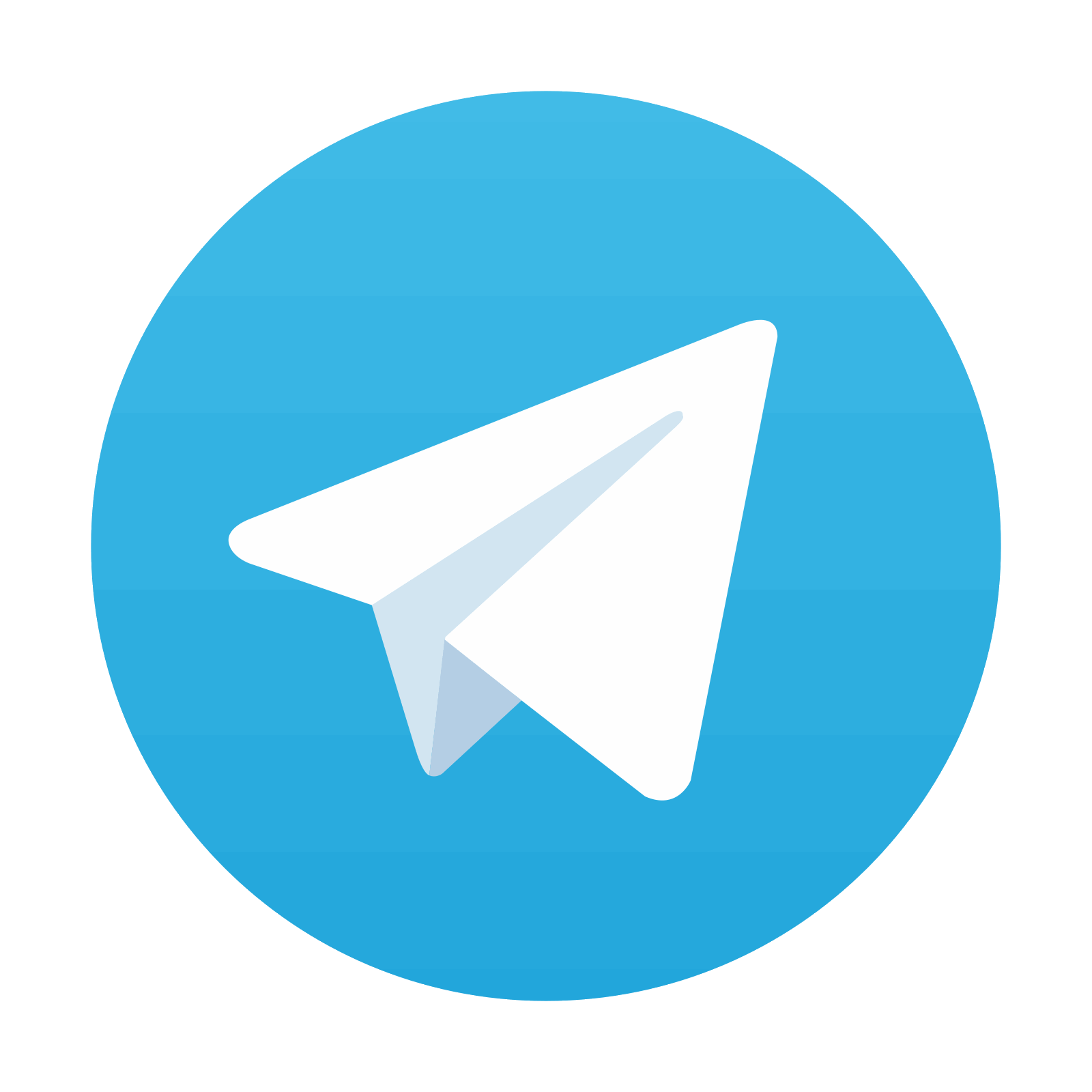
Stay updated, free articles. Join our Telegram channel

Full access? Get Clinical Tree
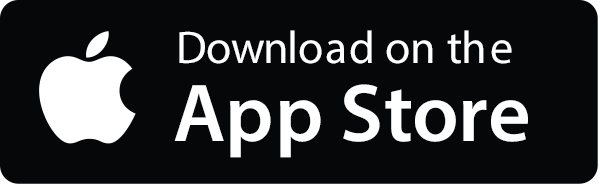
