Discuss key research design decisions for a quantitative study
Discuss the concepts of causality and identify criteria for causal relationships
Describe and identify experimental, quasi-experimental, and nonexperimental designs
Distinguish between cross-sectional and longitudinal designs
Identify and evaluate alternative methods of controlling confounding variables
Understand various threats to the validity of quantitative studies
Evaluate a quantitative study in terms of its research design and methods of controlling confounding variables
Define new terms in the chapter
Key Terms
Attrition
Baseline data
Blinding
Case-control design
Cause
Cohort design
Comparison group
Construct validity
Control group
Correlation
Correlational research
Crossover design
Cross-sectional design
Descriptive research
Effect
Experiment
Experimental group
External validity
History threat
Homogeneity
Internal validity
Intervention
Longitudinal design
Matching
Maturation threat
Mortality threat
Nonequivalent control group design
Nonexperimental study
Placebo
Posttest data
Pretest–posttest design
Prospective design
Quasi-experiment
Randomization (random assignment)
Randomized controlled trial (RCT)
Research design
Retrospective design
Selection threat (self-selection)
Statistical conclusion validity
Statistical power
Threats to validity
Time-series design
Validity
For quantitative studies, no aspect of a study’s methods has a bigger impact on the validity of the results than the research design—particularly if the inquiry is cause-probing. This chapter has information about how you can draw conclusions about key aspects of evidence quality in a quantitative study.
OVERVIEW OF RESEARCH DESIGN ISSUES
The research design of a study spells out the strategies that researchers adopt to answer their questions and test their hypotheses. This section describes some basic design issues.
Key Research Design Features
Table 9.1 describes seven key features that are typically addressed in the design of a quantitative study. Design decisions that researchers must make include the following:
Will there be an intervention? A basic design issue is whether or not researchers will introduce an intervention and test its effects—the distinction between experimental and nonexperimental research.
What types of comparisons will be made? Quantitative researchers often make comparisons to provide an interpretive context. Sometimes, the same people are compared at different points in time (e.g., preoperatively vs. postoperatively), but often, different people are compared (e.g., those getting vs. not getting an intervention).
How will confounding variables be controlled? In quantitative research, efforts are often made to control factors extraneous to the research question. This chapter discusses techniques for controlling confounding variables.
Will blinding be used? Researchers must decide if information about the study (e.g., who is getting an intervention) will be withheld from data collectors, study participants, or others to minimize the risk of expectation bias—i.e., the risk that such knowledge could influence study outcomes.
How often will data be collected? Data sometimes are collected from participants at a single point in time (cross-sectionally), but other studies involve multiple points of data collection (longitudinally).
When will “effects” be measured, relative to potential causes? Some studies collect information about outcomes and then look back retrospectively for potential causes. Other studies begin with a potential cause and then see what outcomes ensue, in a prospective fashion.
Where will the study take place? Data for quantitative studies are collected in various settings, such as in hospitals or people’s homes. Another decision concerns how many different sites will be involved in the study—a decision that could affect the generalizability of the results.
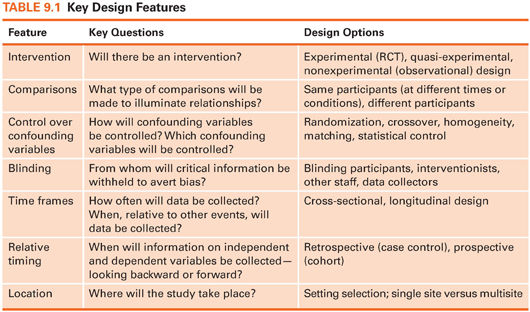
Many design decisions are independent of the others. For example, both experimental and nonexperimental studies can compare different people or the same people at different times. This chapter describes the implications of design decisions on the study’s rigor.
![]() | TIP Information about the research design usually appears early in the method section of a research article. |
Causality
Many research questions are about causes and effects. For example, does turning patients cause reductions in pressure ulcers? Does exercise cause improvements in heart function? Causality is a hotly debated issue, but we all understand the general concept of a cause. For example, we understand that failure to sleep causes fatigue and that high caloric intake causes weight gain. Most phenomena are multiply determined. Weight gain, for example, can reflect high caloric intake or other factors. Causes are seldom deterministic; they only increase the likelihood that an effect will occur. For example, smoking is a cause of lung cancer, but not everyone who smokes develops lung cancer, and not everyone with lung cancer smoked.
While it might be easy to grasp what researchers mean when they talk about a cause, what exactly is an effect? One way to understand an effect is by conceptualizing a counterfactual (Shadish et al., 2002). A counterfactual is what would happen to people if they were exposed to a causal influence and were simultaneously not exposed to it. An effect represents the difference between what actually did happen with the exposure and what would have happened without it. A counterfactual clearly can never be realized, but it is a good model to keep in mind in thinking about research design.
Three criteria for establishing causal relationships are attributed to John Stuart Mill.
1. Temporal: A cause must precede an effect in time. If we test the hypothesis that smoking causes lung cancer, we need to show that cancer occurred after smoking began.
2. Relationship: There must be an association between the presumed cause and the effect. In our example, we have to demonstrate an association between smoking and cancer—that is, that a higher percentage of smokers than nonsmokers get lung cancer.
3. Confounders: The relationship cannot be explained as being caused by a third variable. Suppose that smokers tended to live predominantly in urban environments. There would then be a possibility that the relationship between smoking and lung cancer reflects an underlying causal connection between the environment and lung cancer.
Other criteria for causality have been proposed. One important criterion in health research is biologic plausibility—evidence from basic physiologic studies that a causal pathway is credible. Researchers investigating causal relationships must provide persuasive evidence regarding these criteria through their research design.
Research Questions and Research Design
Quantitative research is used to address different types of research questions, and different designs are appropriate for different questions. In this chapter, we focus primarily on designs for Therapy, Prognosis, Etiology/Harm, and Description questions (Meaning questions require a qualitative approach and are discussed in Chapter 11).
Except for Description, questions that call for a quantitative approach usually concern causal relationships:
Does a telephone counseling intervention for patients with prostate cancer cause improvements in their psychological distress? (Therapy question)
Do birth weights under 1,500 g cause developmental delays in children? (Prognosis question)
Does salt cause high blood pressure? (Etiology/Harm question)
Some designs are better at revealing cause-and-effect relationships than others. In particular, experimental designs (randomized controlled trials or RCTs) are the best possible designs for illuminating causal relationships—but it is not always possible to use such designs. Table 9.2 summarizes a “hierarchy” of designs for answering different types of causal questions and augments the evidence hierarchy presented in Figure 2.1 (see Chapter 2).
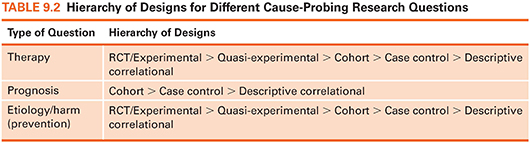
EXPERIMENTAL, QUASI-EXPERIMENTAL, AND NONEXPERIMENTAL DESIGNS
This section describes designs that differ with regard to whether or not there is an intervention.
Experimental Design: Randomized Controlled Trials
Early scientists learned that complexities occurring in nature can make it difficult to understand relationships through pure observation. This problem was addressed by isolating phenomena and controlling the conditions under which they occurred. These experimental procedures have been adopted by researchers interested in human physiology and behavior.
Characteristics of True Experiments
A true experiment or RCT is characterized by the following properties:
Intervention—The experimenter does something to some participants by manipulating the independent variable.
Control—The experimenter introduces controls into the study, including devising an approximation of a counterfactual—usually a control group that does not receive the intervention.
Randomization—The experimenter assigns participants to a control or experimental condition on a random basis.
By introducing an intervention, experimenters consciously vary the independent variable and then observe its effect on the outcome. To illustrate, suppose we were investigating the effect of gentle massage (I), compared to no massage (C), on pain (O) in nursing home residents (P). One experimental design for this question is a pretest–posttest design, which involves observing the outcome (pain levels) before and after the intervention. Participants in the experimental group receive a gentle massage, whereas those in the control group do not. This design permits us to see if changes in pain were caused by the massage because only some people received it, providing an important comparison. In this example, we met the first criterion of a true experiment by varying massage receipt, the independent variable.
This example also meets the second requirement for experiments, use of a control group. Inferences about causality require a comparison, but not all comparisons yield equally persuasive evidence. For example, if we were to supplement the diet of premature babies (P) with special nutrients (I) for 2 weeks, their weight (O) at the end of 2 weeks would tell us nothing about the intervention’s effectiveness. At a minimum, we would need to compare posttreatment weight with pretreatment weight to see if weight had increased. But suppose we find an average weight gain of 1 pound. Does this finding support an inference of a causal connection between the nutritional intervention (the independent variable) and weight gain (the outcome)? No, because infants normally gain weight as they mature. Without a control group—a group that does not receive the supplements (C)—it is impossible to separate the effects of maturation from those of the treatment. The term control group refers to a group of participants whose performance on an outcome is used to evaluate the performance of the experimental group (the group getting the intervention) on the same outcome.
Experimental designs also involve placing participants in groups at random. Through randomization (also called random assignment), every participant has an equal chance of being included in any group. If people are randomly assigned, there is no systematic bias in the groups with regard to attributes that may affect the dependent variable. Randomly assigned groups are expected to be comparable, on average, with respect to an infinite number of biologic, psychological, and social traits at the outset of the study. Group differences on outcomes observed after randomization can therefore be inferred as being caused by the intervention.
Random assignment can be accomplished by flipping a coin or pulling names from a hat. Researchers typically either use computers to perform the randomization.
![]() | TIP There is a lot of confusion about random assignment versus random sampling. Random assignment is a signature of an experimental design (RCT). If subjects are not randomly assigned to intervention groups, then the design is not a true experiment. Random sampling, by contrast, refers to a method of selecting people for a study, as we discuss in Chapter 10. Random sampling is not a signature of an experimental design. In fact, most RCTs do not involve random sampling. |
Experimental Designs
The most basic experimental design involves randomizing people to different groups and then measuring outcomes. This design is sometimes called a posttest-only design. A more widely used design, discussed earlier, is the pretest–posttest design, which involves collecting pretest data (often called baseline data) on the outcome before the intervention and posttest (outcome) data after it.
Example of a pretest–posttest design
Berry and coresearchers (2015) tested the effectiveness of a postpartum weight management intervention for low-income women. The women were randomly assigned to be in the intervention group or in a control group. Data on weight, adiposity, and health behaviors were gathered at baseline and at the end of the intervention.
![]() |
The people who are randomly assigned to different conditions usually are different people. For example, if we were testing the effect of music on agitation (O) in patients with dementia (P), we could give some patients music (I) and others no music (C). A crossover design, by contrast, involves exposing people to more than one treatment. Such studies are true experiments only if people are randomly assigned to different orderings of treatment. For example, if a crossover design were used to compare the effects of music on patients with dementia, some would be randomly assigned to receive music first followed by a period of no music, and others would receive no music first. In such a study, the three conditions for an experiment have been met: There is intervention, randomization, and control—with subjects serving as their own control group.
A crossover design has the advantage of ensuring the highest possible equivalence among the people exposed to different conditions. Such designs are sometimes inappropriate, however, because of possible carryover effects. When subjects are exposed to two different treatments, they may be influenced in the second condition by their experience in the first. However, when carryover effects are implausible, as when intervention effects are immediate and short-lived, a crossover design is powerful.
Example of a crossover design
DiLibero and colleagues (2015) used a crossover design to test whether withholding or continuing enteral feedings during repositioning of patients affected the incidence of aspiration. Patients were randomly assigned to different orderings of enteral feeding treatment.
Experimental and Control Conditions
To give an intervention a fair test, researchers need to design one that is of sufficient intensity and duration that effects on the outcome might reasonably be expected. Researchers describe the intervention in formal protocols that stipulate exactly what the treatment is.
Researchers have choices about what to use as the control condition, and the decision affects the interpretation of the findings. Among the possibilities for the control condition are the following:
“Usual care”—standard or normal procedures are used to treat patients
An alternative treatment (e.g., music vs. massage)
A placebo or pseudointervention presumed to have no therapeutic value
An attention control condition (the control group gets attention but not the intervention’s active ingredients)
Delayed treatment, i.e., control group members are wait-listed and exposed to the intervention at a later point
Example of a wait-listed control group
Song and Lindquist (2015) tested the effectiveness of a mindfulness-based stress reduction intervention for reducing stress, anxiety, and depression in Korean nursing students. Students were randomly assigned to the intervention group or a wait-listed control group.
Ethically, the delayed treatment design is attractive but is not always feasible. Testing two alternative interventions is also appealing ethically, but the risk is that the results will be inconclusive because it may be difficult to detect differential effects of two good treatments.
Researchers must also consider possibilities for blinding. Many nursing interventions do not lend themselves easily to blinding. For example, if the intervention were a smoking cessation program, participants would know whether they were receiving the intervention, and the intervener would know who was in the program. It is usually possible and desirable, however, to blind the participants’ group status from the people collecting outcome data.
Example of an experiment with blinding
Kundu and colleagues (2014) studied the effect of Reiki therapy on postoperative pain in children undergoing dental procedures. Study participants were blinded—those in the control group received a sham Reiki treatment. Those who recorded the children’s pain scores, the nurses caring for the children, and the children’s parents were also blinded to group assignments.
![]() | TIP The term double blind is widely used when more than one group is blinded (e.g., participants and interventionists). However, this term is falling into disfavor because of its ambiguity, in favor of clear specifications about exactly who was blinded and who was not. |
Advantages and Disadvantages of Experiments
RCTs are the “gold standard” for intervention studies (Therapy questions) because they yield the most persuasive evidence about the effects of an intervention. Through randomization to groups, researchers come as close as possible to attaining an “ideal” counterfactual.
The great strength of experiments lies in the confidence with which causal relationships can be inferred. Through the controls imposed by intervening, comparing, and—especially—randomizing, alternative explanations can often be ruled out. For this reason, meta-analyses of RCTs, which integrate evidence from multiple experimental studies, are at the pinnacle of evidence hierarchies for questions relating to causes (Fig. 2.1 of Chapter 2).
Despite the advantages of experiments, they have limitations. First, many interesting variables simply are not amenable to intervention. A large number of human traits, such as disease or health habits, cannot be randomly conferred. That is why RCTs are not at the top of the hierarchy for Prognosis questions (Table 9.2), which concern the consequences of health problems. For example, infants could not be randomly assigned to having cystic fibrosis to see if this disease causes poor psychosocial adjustment.
Second, many variables could technically—but not ethically—be experimentally varied. For example, there have been no RCTs to study the effect of cigarette smoking on lung cancer. Such a study would require people to be assigned randomly to a smoking group (people forced to smoke) or a nonsmoking group (people prohibited from smoking). Thus, although RCTs are technically at the top of the evidence hierarchy for Etiology/Harm questions (Table 9.2), many etiology questions cannot be answered using an experimental design.
Sometimes, RCTs are not feasible because of practical issues. It may, for instance, be impossible to secure the administrative approval to randomize people to groups. In summary, experimental designs have some limitations that restrict their use for some real-world problems; nevertheless, RCTs have a clear superiority to other designs for testing causal hypotheses.
Quasi-Experiments
Quasi-experiments (called trials without randomization in the medical literature) also involve an intervention; however, quasi-experimental designs lack randomization, the signature of a true experiment. Some quasi-experiments even lack a control group. The signature of a quasi-experimental design is the implementation and testing of an intervention in the absence of randomization.
Quasi-Experimental Designs
A frequently used quasi-experimental design is the nonequivalent control group pretest–posttest design, which involves comparing two or more groups of people before and after implementing an intervention. For example, suppose we wished to study the effect of a chair yoga intervention (I) for older people (P) on quality of life (O). The intervention is being offered to everyone at a community senior center, and randomization is not possible. For comparative purposes, we collect outcome data at a different senior center that is not instituting the intervention (C). Data on quality of life (QOL) are collected from both groups at baseline and 10 weeks later.
This quasi-experimental design is identical to a pretest–posttest experimental design except people were not randomized to groups. The quasi-experimental design is weaker because, without randomization, it cannot be assumed that the experimental and comparison groups are equivalent at the outset. The design is, nevertheless, strong because the baseline data allow us to see whether elders in the two senior centers had similar QOL scores, on average, before the intervention. If the groups are comparable at baseline, we could be relatively confident inferring that posttest differences in QOL were the result of the yoga intervention. If QOL scores are different initially, however, postintervention differences are hard to interpret. Note that in quasi-experiments, the term comparison group is sometimes used in lieu of control group to refer to the group against which outcomes in the treatment group are evaluated.
Now suppose we had been unable to collect baseline data. Such a design (nonequivalent control group posttest-only) has a flaw that is hard to remedy. We no longer have information about initial equivalence. If QOL in the experimental group is higher than that in the control group at the posttest, can we conclude that the intervention caused improved QOL? There could be other explanations for the differences. In particular, QOL in the two centers might have differed initially. The hallmark of strong quasi-experiments is the effort to introduce some controls, such as baseline measurements.
Example of a nonequivalent control group design
Hsu and colleagues (2015) used a nonequivalent control group pretest–posttest design to test the effect of an online caring curriculum to enhance nurses’ caring behavior. Nurses in one hospital received the intervention, whereas those in another hospital did not. Data on the nurses’ caring behaviors were gathered from both groups before and after the intervention.
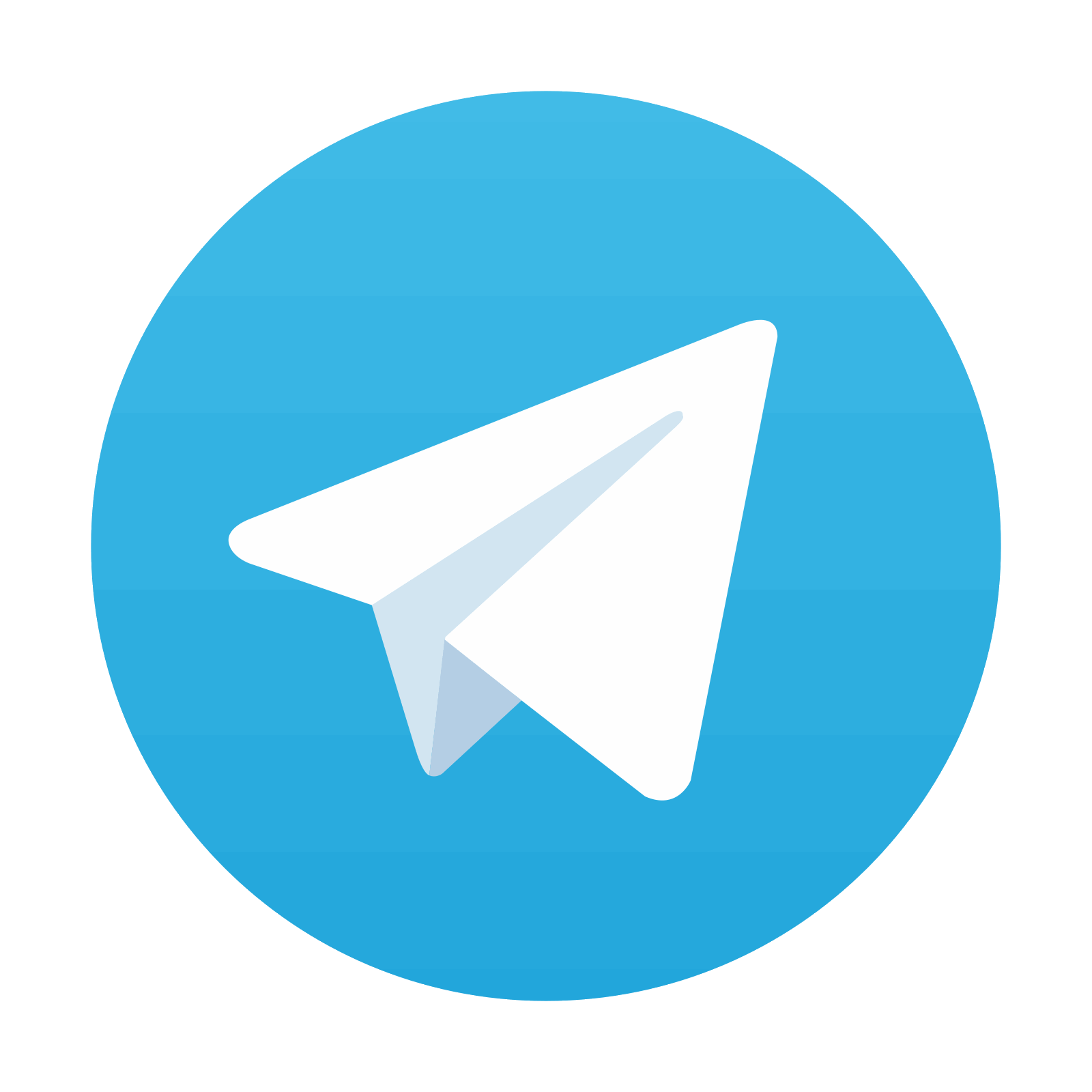
Stay updated, free articles. Join our Telegram channel

Full access? Get Clinical Tree
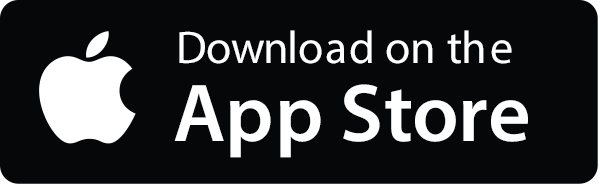
