The use of clinical quality measures for improving healthcare and informing performance-based payment methods is becoming increasingly important as new value-based payment models evolve. Various forms of health information technology allow for the collection of data that can be analyzed and provided to clinicians and healthcare systems for measurement of care coordination; federal, state, and local governments for oversight and payment; and most importantly, patients, families, and caregivers for healthcare decision-making. The collection and exchange of healthcare information allows doctors, nurses, pharmacists, other healthcare providers, and patients to appropriately access and securely share a patient’s vital medical information electronically—improving the speed and quality of clinical quality measurement. Electronic clinical quality measures (eCQMs) use data electronically extracted from electronic health records (EHRs) and health information technology systems to measure the quality of healthcare provided. While it is important to gather clinical data to make decisions regarding the course of care, it is just as important to collect information to measure the patient’s experience of care, about what they want to get from the treatment, and to engage them in the decision-making process. Patient-reported outcomes measures provide information to patients on how other patients have achieved their intended goals from clinicians and provide information to clinicians to improve the quality of care that they provide. There is a continuing need to evolve the field of healthcare informatics, promote interoperability among health records, reduce collection burden, and improve the value of CQMs. We often think of quality measures as a complicated and time-consuming collection of data that has questionable use or value. Quality measurement is sometimes considered to be an administrative time drain. However, as we think about taking a journey, we often establish our destination and then plot out how we want to get there, the mode of travel, the stops along the way, how long it will take us, what we want to do when we get there, and the budget we want to stay within. We may encounter difficulties along the way, then we must recalculate and take detours, but we always keep in mind the destination we are trying to reach. Quality measurement serves that same purpose in the healthcare journey. We establish the outcome we want to achieve with an intervention or prevention approach, and then we plot out our path and use quality measures and standards to determine if we are meeting the goals. Quality measurement helps us to decide whether we are achieving the desired goals and outcomes and how we compare to our peers or best in class. Measurement reveals areas in which we can improve and points to areas of best practices. Measurement provides information for consumers which they can use in the decision-making related to the type of providers they want to care for them or their loved ones. Quality measurement has been used for centuries although frequently referenced as a new activity. Florence Nightingale highlighted the importance of quality measurement as she traveled with 38 other nurses to a military camp during the Crimean War. When she arrived, she noted the deplorable conditions in which treatment was being provided. Combining her passion for helping with available scientific evidence, Ms. Nightingale began to measure the quality of care through monitoring the rate of mortality which was nearly 60%. She worked to decrease the rate by employing sanitation and nutrition intervention and measuring success to achieve a decrease in the rate to 42% and then to 2.2%. Dr. Ernest Codman, MD was a surgeon in Boston that brought improvements in care by measuring the outcomes of patients over time. He is well known for his work in 1911 in which he followed the outcomes of patients that were discharged from the hospital. He believed that the hospital and the surgeon should follow patients long enough to determine whether the treatment was successful and to learn from those with complications (Brand, 2009). During the same period, Dr. Codman was asked to form and chair the Committee on Standardization of Hospitals for the American College of Surgeons. That committee later evolved into the Joint Commission on Accreditation of Healthcare Organizations, now known as The Joint Commission (Warshaw, 2014). Avedis Donabedian was instrumental in the 1950s and 1960s in developing a method to measure quality in healthcare. He advanced the field by analyzing healthcare systems and resources to determine the impact on overall quality of care. In 2000 and 2001 the Institute of Medicine (IOM) released two landmark publications. The first was To Err Is Human and the second Crossing the Quality Chasm, continue to guide quality measurement and improvement even today. The IOM defined quality as “the degree to which health services for individuals and populations increase the likelihood of desired health outcomes and are consistent with current professional knowledge” (Institute of Medicine, 1990). In the first report, To Err Is Human: Building a Safer Health System, the IOM concluded that tens of thousands of Americans die each year from errors in their care, and hundreds of thousands suffer or barely escape from nonfatal injuries that a truly highquality care system would largely prevent. The report establishes a quality measurement goal of a 50% reduction (at a minimum) in errors over five years (Institute of Medicine, 2000). Crossing the Quality Chasm magnified the need for urgent change in the United States to close the gap between the current state of healthcare and the desired state. The report highlighted the fact that Americans were not receiving care that meets their needs and based on the best scientific knowledge (Institute of Medicine, 2001). The publication outlined an organizing framework to better align the incentives inherent in payment and accountability with improvements in quality, emphasizing the need for evidence-based practice, quality measurement, and better clinical information systems. One of the recommendations called for Congress to authorize and appropriate funds for the Department of Health and Human Services to establish monitoring and tracking processes for use in evaluating the progress of the health system to achieve the aims of safety, effectiveness, patient-centeredness, timeliness, efficiency, and equity. In 1966 Avedis Donabedian introduced a classification of quality measures including three components: structure, process, and outcome. Donabedian notes that structure includes such things as the administrative structure and operations of programs and institutions providing care and fiscal organization. Many of the early electronic incentive goals for health information exchange (HIE) focused on developing the structure that would allow for the exchange of information. The Health Information Technology for Economic and Clinical Health Act (HITECH) of the American Recovery and Reinvestment Act of 2009 (ARA) set national meaningful use goals to spur EHR adoption (Blumenthal, 2010). The Recovery Act specifies the following three components of Meaningful Use: (1) Use of certified EHR in a meaningful manner (e.g., e-prescribing); (2) Use of certified EHR technology for electronic exchange of health information to improve quality of healthcare; and (3) Use of certified EHR technology to submit CQMs and other such measures selected by the Secretary (Centers for Medicare & Medicaid Services, 2011a, 2011b). The second type of measure described by Donabedian is called process measures. Process measures are used to assess the application of what is known to be good practices in care. Process measures include, for example, assessing the performance of recommended diagnostic and screening tests. The process of performing the tests will presumably lead to the desired outcome of prevention or early detection of disease processes. He described outcome measures as the components of care delivered that focuses on recovery from the intervention, restoration of function, and survival (Donabedian, 2005). Today outcome measures often focus on mortality, hospital readmissions, improved function, and the absence of complications. The Meaningful Measures Framework is the Centers for Medicare & Medicaid Services’ (CMS’) new initiative which identifies the highest priorities for quality measurement and improvement (Centers for Medicare & Medicaid Services, 2018a, 2018b). It provides a lens to determine core issues that are the most critical to providing high-quality care and improving individual outcomes. The Meaningful Measures Initiative was developed collaboratively with input from a wide variety of stakeholders. It also draws on prior work performed by the Health Care Payment Learning and Action Network (HCPLAN), other federal agencies, the National Quality Forum, and the National Academies of Medicine. It includes perspectives from patient representatives, clinicians and providers, measure developers, and other experts such as the Core Quality Measures Collaborative. The Meaningful Measures Areas serve as the connectors between CMS strategic goals and individual measures/initiatives that demonstrate how to achieve high-quality outcomes for Medicare, Medicaid, and Child Health Insurance Program (CHIP) beneficiaries. They include quality topics which reflect core issues that are vital to high-quality care and better patient outcomes. Examples of Meaningful Measure Areas include Healthcare-Associated Infections and Prevention and Treatment of Opioid and Substance Use Disorders. Each of these Meaningful Measure Areas helps to make the connection to specific CMS strategic goals such as “Empower patients and doctors to make decisions about their healthcare” and “Support innovative approaches to improve quality, safety, accessibility, and affordability.” Meaningful Measures are intended to move payment toward value by focusing everyone’s efforts on the same quality areas. CMS identified the following important principles for determining whether a measure is meaningful: 1. Addresses high-impact measure areas that safeguard public health; 2. Patient-centered and meaningful to patients; 3. Outcome-based where possible; 4. Fulfills requirements in programs’ statutes; 5. Minimizes level of burden for providers; 6. Significant opportunity for improvement; 7. Addresses measure needs for population-based payment through alternative payment models (APMs); and, 8. Aligns across programs and with other payers (Medicaid, commercial payers). The Meaningful Measures framework contains 19 Meaningful Measure areas organized into specific, overarching healthcare quality priorities. Meaningful Measure area number 9 focuses on the need for interoperability among healthcare information systems (Table 19.1). TABLE 19.1. Meaningful Measure Area 9 The Health Care Payment Learning & Action Network (HCPLAN) is a public–private partnership whose mission is to accelerate the healthcare system’s transition to APMs by aligning the innovation, power, and reach of the private and public sectors. Launched in 2015, the HCPLAN has membership from a wide variety of stakeholders including federal and state governments, academic centers, associations and professional societies, commercial payers, healthcare providers, patient and consumer advocates, private employers, health plans, and many others. The HCPLAN’s stated purpose is to facilitate the shift from the fee-for-service (FFS) payment model to a model that pays providers for quality care, improved health, and lower costs. The HCPLAN provides resources and learning opportunities to catalyze movement toward the adoption of APMs with an established goal of tying 50% of U.S. healthcare payments to APMs by the end of 2018 (Health Care Payment Learning and Action Network, 2018). The drive toward alignment in payment approaches across and within the public and private sectors of the U.S. healthcare system is not likely to happen on its own. Many are needed to advance the goals, and a number of topics must be explored to support clinicians and providers in their efforts. The HCPLAN has developed several resources and white papers to support the movement. The papers were developed with multidisciplinary stakeholder involvement and released for public comment before finalizing. One of the white papers explores quality performance measures in a population-based performance payment approach. The HCPLAN concludes that performance measurement, which evaluates provider organizations on the basis of quality and outcomes, is foundational to the success of population-based performance models because it helps ensure that patients receive high-quality care and achieve their health goal. The White Paper provides principles and recommendations on approaches to performance measurement in performance-based payment models. One of the principles highlights the notion that the national infrastructure must be sufficient to systematically collect, use, and report clinically rich and patient-reported data. The HCPLAN also developed a white paper on the importance of sharing data in population-based payment models (Health Care Payment Learning and Action Network, 2016). The Data Sharing White Paper focuses on the importance of sharing important patient data to inform clinical decision-making, allow payers to assess provider performance, and support increased alignment across public and private payers. While everyone realizes improvement in information exchange is a necessity for coordination of care, reaching these goals remains challenging. The field of information exchange continues to develop at a pace that is slower than many would hope. The HCPLAN provides recommendations on the key characteristics of data sharing that can help sustain population-based payment models. The principles for data sharing include: • Data sharing in population-based payment will need to be different than data sharing in FFS models; • Personal data should follow the patient; • Population-level data should be treated as a public good; and • Widespread data sharing may necessitate thirdparty intermediaries. One area of complaint regarding quality measurement is the lack of alignment between payers, oversight and accreditation entities, clinical registries, health systems, and other entities. Each entity may require the reporting of quality measures in a slightly different manner or completely different quality measures. There are several efforts underway to align measures across many stakeholders. The Core Quality Measure Collaborative (CQMC) is a broad-based coalition of healthcare leaders convened by America’s Health Insurance Plans (AHIP) starting in 2015. The Collaborative convenes a wide array of stakeholders including CMS, health insurance providers, medical associations, consumer groups, and purchasers. The CQMC is working on (1) ongoing maintenance of the existing core measure sets to reflect the changing measurement landscape, including, but not limited to, changes in clinical practice guidelines, data sources, or risk adjustment; (2) expanding into new clinical areas not yet addressed; and (3) identifying gaps in measurement and challenges in implementation in order to advance adoption of the core sets (Core Quality Measures Collaborative, 2019). Quality measurement is only as good as the data from which it is derived. The collection of data for quality measures is accomplished through several methods. In the early inception of quality measurement, most measures were either calculated using data from administrative claims or manual abstraction of a medical record. Claims Data Administrative claims data are used to calculate many types of process and outcomes measures. Common measures calculated from claims may include assessing the completion of screening exams, readmitting patients to the hospital in a certain time frame, and checking mortality rates for multiple conditions. Claims-derived measures have many benefits, in that the data is readily available from billing data, thus requiring no additional work by clinicians. They also allow for comparing a large number of patients for population-based measures. Data analytics capabilities have also improved, allowing for more rapid analysis and predictive models using artificial intelligence. Administrative claims also have its drawbacks, in that the claims may contain errors or have important fields necessary for calculating the measures missing. Many of the clinical findings necessary to develop the measures may not be included with the administrative data since claims have their origin in billing information versus clinical information. Chart Abstraction
19
Quality Measurement and the Importance of Nursing Informatics
ABSTRACT
IMPORTANCE OF QUALITY MEASUREMENT
EVOLUTION OF QUALITY MEASUREMENT
CLASSIFICATION OF QUALITY MEASURES (STRUCTURE, PROCESS, OUTCOMES, AND EXPERIENCE)
The Meaningful Measurement Framework
Healthcare Payment Learning and Action Network
The Core Quality Measures Collaborative
Methods of Collecting Data for Quality Measurement
Stay updated, free articles. Join our Telegram channel

Full access? Get Clinical Tree
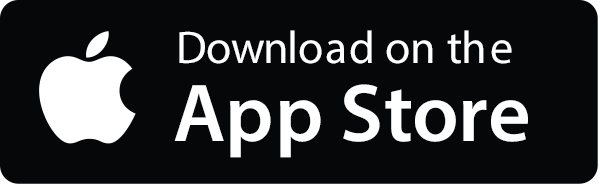
