Health systems and public health agencies increasingly use informatics and artificial intelligence tools to create and share digital information for public health purposes (public health informatics) and health management (clinical informatics) (United States Office of the National Coordinator [ONC], 2018). These parallel efforts to improve health at individual and population levels merge to form the discipline of population health informatics (PopHI) (ONC, 2018). The core functions of public health (assessment, policy development, and assurance) (United States Centers for Disease Control and Prevention [CDC], 2011) and the goals of population health management (better outcomes and care at reduced cost) (Institute for Healthcare Improvement [IHI], n.d.) both depend on an information technology (IT) infrastructure that enables data-based decision-making. (Overhage & Dixon, 2018; CDC, 2011; IHI, n.d.; ONC, 2018). Both public health and population health management likewise emphasize social determinants of health and are concerned with outcomes measurement (IHI, n.d.; Institute of Medicine [IOM], 2014a, 2014b). In 2008 the Institute for Healthcare Improvement (IHI) described the Triple Aim of improving population health and the patient experience of care, and reducing per capita cost. Methods for measuring population health improvement were subsequently described by the IHI and others, relying on EHR data as a primary source along with multiple other sources, such as healthcare systems, public health departments, social service agencies, school systems, and employers (Friedman & Parrish, 2010; Stoto, 2013). The notion of population health measurement for accountability in healthcare was inherent in the U.S. Accountable Care Act (ACA) that reshaped healthcare systems and payment nationally (Stoto, 2013). The ACA shifted the focus of healthcare from episodic care for individuals to health management of populations, emphasizing primary care and prevention. Increasingly researchers, informaticians, practitioners, and policymakers embraced the notion of health systems as important contributors to and consumers of population health data for healthcare delivery systems, public health agencies, and communitybased organizations (Stoto, 2013). Thus, despite differences in origin and perspective, the notion of PopHI as the science of information management for populations reflects both the tenets of public health and population health management. Defining Population Health Informatics. This chapter views PopHI as an evolving discipline supporting the goals of population health management that has overlapping and synergistic interests with public health informatics (PHI). Indeed, from an informatics perspective, many principles of PopHI and PHI are the same regardless of origins and stakeholders. Heuristically, PHI strives to meet the information needs of governmental organizations that work to protect, promote, and improve the public’s health for the residents of a particular geographic jurisdiction; while PopHI strives to meet the needs of health systems that manage their member groups at risk of poor outcomes (e.g., diabetes, heart failure, multimorbid conditions). Population health management requires new ways of obtaining more information to drive analytics, enabling risk stratification and outcomes tracking, while public health likewise turns to informatics solutions to improve the flow of data for surveillance and other important public health functions. The shared population-based approach of both disciplines builds on years of scholarship from diverse sources and that lead to the same conclusion in alignment with the goals of the learning health system: informatics solutions help stakeholders leverage data to support decision-making and improve the health of populations (Gamache, Kharrazi, & Weiner, 2018; IHI, n.d.; Overhage & Dixon, 2018; Kindig & Stoddart, 2003; Etches, Frank, Ruggiero, & Manuel, 2006; Kharrazi et al., 2017; World Health Organization [WHO], 2012). Definitions of key concepts in PopHI are provided below: • Health disparities/health inequities: The unfair and avoidable differences in health status seen within and between countries (WHO, 2012). • Population health informatics: It addresses the IT and analytic needs of groups and organizations responsible for the health management of defined populations (Gamache et al., 2018, p. 200). • Population health management: A set of actions, backed and dictated by the flow of information, that guide providers to the best way to providing optimal services to a group of patients with specific healthcare needs (Bresnick, 2015). • Public health informatics: The systematic application of information, computer science, and technology to public health practice, research, and learning including surveillance, reporting, and health promotion (Yasnoff, O’Carroll, Koo, Linkins, & Kilbourne, 2000; AMIA, n.d.). • Social determinants of health: The conditions influencing health outcomes, in which people are born, grow, live, work, and age (WHO, 2012). • Surveillance: An ongoing, systematic collection, analysis, and interpretation of health-related data essential to the planning, implementation, and evaluation of public health practice. Surveillance is undertaken to inform disease prevention and control measures (World Health Organization [WHO], n.d.-a). • Triple aim: Improving the patient experience of care (including quality and satisfaction); improving the health of populations; and reducing the per capita cost of health care (IHI, n.d.). Population of Interest. Defining the population of interest is key to discerning differences between the closely aligned perspectives of public health and population health management. When the population of interest relates to health system members, the perspective is that of population health management versus public health. Conversely, when the population of interest relates to geographically based jurisdictions such as states, counties, or nations, the perspective is that of public health, not population health management (Kharrazi et al., 2017; IHI, n.d.). Population Health Record. The notion of a health record for populations (PopHR) was advanced in the late 1990s (Friedman & Parrish, 2010; Shaban-Nejad, Lavigne, Okhmatovskaia, & Buckeridge, 2017). PopHR literature describes using heterogeneous data from a geographybased public health perspective to enable a broad view connecting health determinants and the health of populations. PopHRs are the ideal platform in which population health management and public health principles enable clinicians and researchers to understand whole-person health in the context of all available data for individuals and populations. Achieving this goal depends on collaboration across sectors and requires attention to security, standards and interoperability, and data governance. Pioneers advocating for a PopHR [AMIA in 1997 and National Committee on Vital and Health Statistics (NCVHS) inclusion of the PopHR as part of a national health information strategy in 1998] suggested models for achieving this goal (Friedman & Parrish, 2010; ShabanNejad et al., 2017). A working semantic Web PopHR application has been developed at McGill University that automates extraction, harmonization, linking, and integration of data from multiple sources to support population health measurement and monitoring to improve decision-making (Shaban-Nejad et al., 2017). This section describes in more detail how informatics tools and processes may be applied by informaticians to advance population health management and public health. Population health informatics leverages health IT to strengthen care management and advance public health data reporting (ONC, 2018; Hodach, Chase, Fortini, & Delaney, 2012). Informatics needs of population health management involve population identification, identification of care gaps, stratification by risk or other factors, patient engagement, care management, and outcomes measurement (Hodach et al., 2012). Population Identification. Populations of interest may be defined based on demographics or other characteristics such as diagnoses (e.g., individuals with diabetes or hearth disease), risks (e.g., pregnant women with a previous preterm birth), or pay source (e.g., individuals with public insurance). Thus, it is incumbent upon health systems to consistently employ national standards for encoding demographics, pay sources, and diagnoses in order to aid in defining populations of interest within and across health systems (ONC, 2018). Identification of Care Gaps. Gaps in care may be identified based on claims data from expected encounters compared to completed encounters, such as routine screenings or immunizations. Such analytics are important for internal quality improvement, incentive payments, and for comparisons across systems (ONC, 2018). Stratification. Stratification of populations based on various criteria to inform decision-making is critical to ensure that clinicians may address risk appropriately, and avoid harm due to lack of important information. Stratification also enables reporting of outcomes specific to populations of interest. Evidence-based guidelines for stratification and appropriate interventions should be incorporated within algorithms used in clinical decision support systems and dashboards (Lin, Evans, Grossman, Tseng, & Krist, 2018). Patient Engagement. Patient engagement is key to improved population health outcomes. It is defined as “the desire and capability to actively choose to participate in care in a way uniquely appropriate to the individual in cooperation with a healthcare provider or institution for the purposes of maximizing outcomes or experiences of care” (Higgins, Larson, & Schnall, 2017, p. 33). Patient engagement is associated with increased patient safety, reduced costs, better care coordination, and more informed data consumers and citizen scientists. Secure informatics solutions are needed to support patient engagement in healthcare that meets the unique needs of individuals (Higgins et al., 2017). Care Management. Ideally, populations of interest may be “managed” or assisted with targeted interventions to improve health, reduce risk, and ensure adequate and appropriate care. The Centers for Medicare and Medicaid Services (CMS) provides details regarding updated care management codes (i.e., CPT codes) and payment information (CMS, 2019a). Documentation of care management programs may include a number of required measures as well as patient assessments and intervention details. Nursing terminologies provide granular details regarding care management interventions (CMS, 2019b). Outcomes Measurement. Health outcomes measurement is defined as measurement of change in the health of an individual, group of people, or population that is attributable to an intervention or series of interventions (Smith, Tang, & Nutbeam, 2006). Such measurement depends on defined outcomes measures (Etches et al., 2006). Outcomes may be retrieved from EHR data as in predefined quality measures, morbidity/mortality statistics, and recorded outcomes as in standardized nursing outcome measures (American Nurses Association [ANA], 2018). Public Health Data Reporting. Population health informatics solutions are needed to support public health in their efforts to prevent epidemics and the spread of disease, protect against environmental hazards, prevent injuries, promote healthy behaviors, respond to and assist in recovery from disasters (Overhage & Dixon, 2018; ONC, 2018). Public health departments depend upon EHR data from clinicians routinely captured in clinical care. The data are shared via interoperable systems that enable secure transfer of the required information. These data are used by state health departments and the national Centers for Disease Control (CDC) to calculate the prevalence and incidence of diseases. All clinicians are required to electronically report diagnosis, laboratory results, radiographic results, medications, and vital signs. Clinicians also report to databases that contain patient health information about a specific disease or program (called registries). As interprofessional team members, nurses are essential to the success of PopHI (RWJF, 2017; Ariosto et al., 2018). Nurses are key communicators in leadership teams, and are skilled at facilitating coordination of care across practices and programs. Nurses regularly collaborate with other professionals to address informatics needs. Nurses have long been concerned about social and behavioral determinants of health; indeed nurses bring a holistic perspective considering the physical, mental, social, and spiritual aspects in the context of an environment. Nurses advocate for the individual and the community as central to the PopHI mission to use information to improve health (RWJF, 2017; Ariosto et al., 2018). Nurse informaticians actively support capacity building for nurses to lead population health, care coordination across settings, and interprofessional and community stakeholder collaboration; and influence practice, education, research, and healthcare policy (Ariosto et al., 2018). Nursing informatics leadership will drive the necessary culture change to improve health and reduce costs using health data. The infrastructure for PopHI is emerging and multifaceted. Building on work initiated in 2012, PopHI experts convened in 2017, and noted an unclear vision and lack of a shared model for a PopHI infrastructure (Kharrazi et al., 2017; Hripcsak et al., 2014). They proposed a national research and development agenda for PopHI that included developing a standardized collaborative framework and infrastructure, advancing technical tools and methods, developing a scientific evidence and knowledge base, and developing a framework for policy, privacy, and sustainability (Kharrazi et al., 2017). Several recommendations detailed the necessary next steps for PopHI infrastructure development (Kharrazi et al., 2017; Hripcsak et al., 2014). Funding for Infrastructure Development and Maintenance. Population health informatics is uniquely synergistic across public and private sectors with alignment of goals and methods to achieve related outcomes. Given this synergy, in order to collectively develop a shared national vision for PopHI and answer questions regarding funding for PopHI infrastructure development and maintenance, a major public–private sector effort should be undertaken to develop and implement a national health data use strategy (Hripcsak et al., 2014; Kharrazi et al., 2017). The governmental public health roles that include assessment, assurance, and policy development do not necessarily include amassing and curating population health data. The extent to which tax dollars should support data warehousing to track population health nationally is under debate. Further, the linking of existing data sources for use in PopHI initiatives is at best piecemeal and experimental. For example, private health systems and organizations such as Optum Labs have a vested interest in funding and maintaining large data sets that may be used to predict health outcomes. Optum Labs data warehouse has numerous partners, is used for research, and is self-sustaining through fees for use in research (Optum Labs Data Warehouse [OLDW], (n.d.). Despite extensive coverage of the US population within the Optum Labs data warehouse, not all populations are represented in the data, and underrepresented communities could be left behind. Research is needed to examine the best ways of linking diverse data sources for PopHI, and advocacy is needed to ensure funding and support for this work across public and private sectors. To achieve public safety and optimize population health, patients, clinicians, and societies are obligated, morally and/or legally, to report health data such as diseases, injuries, or adverse events (Goodman, 2010; Mann, Savulescu, & Sahakian, 2016). The ethical management of data sources used to protect the public good is imperative, at minimum, to ensure privacy and security for sensitive patient data. Fundamental rights of individuals and society have been explicated, including privacy rights and the right to benefit from research; as well as the moral and ethical duties of society. Privacy Rights. Privacy rights are protected by the Health Insurance Portability and Accountability Act (HIPAA), with exceptions for purposes of serving a compelling public health, safety, or welfare need (United States Health and Human Services [HHS], 2019). Right to Benefit from Research. Furthermore, the notion of a human right to benefit from research is key to understanding the moral landscape of PopHI. Adding such a human rights perspective to discourse on preventing harm offers a positive framework for policy development needed in this area around consent, privacy, security, accountability, and interoperability (Salerno, Knoppers, Lee, Hlaing, & Goodman, 2017). In addition, a human rights stance toward the use of IT for public health will address potential barriers to reporting such as the withholding of anonymized health data from public health analysis (Goodman, 2010; Overhage & Dixon, 2018). Moral and Ethical Duties. The law describes several moral and ethical duties that may apply to use of data to ensure and promote population health. These include but are not limited to duty to warn (responsibility to inform authorities, i.e., mandated reporting); public health surveillance (governmental responsibility to monitor populations in order to protect the public from crime, disease, or disaster); and duty of easy rescue (failing to come to the rescue of another party who could face potential injury or death) (Goodman, 2010; Overhage & Dixon, 2018; Giubilini, Douglas, Maslen, & Savulescu, 2018). The disproportionate burden of disease and death for underserved populations in the case of the COVID-19 pandemic exemplifies the critical need to examine ethical applications of PopHI in health care (Yancy, 2020). These are embedded within ethical frameworks that have been developed for the science of epidemiology. The goals of epidemiology, defined as the study of the causes and distributions of diseases in human populations so that we may identify ways to prevent and control disease (Galea, 2013), align closely with the goals of PopHI. The ethical underpinnings of epidemiology lend insight into the ethics of PopHI. Frameworks. In 2000, the American College of Epidemiology published Ethics Guidelines around the use of data in research to protect the welfare and rights of research participants and to help ensure optimization and equitable distribution of potential benefits of epidemiologic research (Salerno et al., 2017). In 2013, Galea argued for a consequentialist epidemiology, concerned with using data to maximize desired outcomes for populations, versus simply understanding causation (Galea, 2013). In 2014, the Framework for Responsible Sharing of Genomic and Health-Related Data was published by the Global Alliance for Genomics and Health. It embraced the human right to benefit from population health data, and was founded on four principles: respect for individuals, families and communities; advancement of research and scientific knowledge; promotion of health, wellbeing, and the fair distribution of benefits; and fostering trust, integrity, and reciprocity (Salerno et al., 2017). These frameworks aid in understanding ethical principles that underlie population health management and PopHI. Policy Development. The ethical infrastructure for PopHI encompasses policy development to ensure protections for individuals as well as to advance a human rights perspective (Hripcsak et al., 2014; HHS, 2019; Goodman, 2010). These notions reflect the values of fair information practice and emphasized data stewardship as a trustworthy approach to data management. Data governance is the process by which responsibilities of stewardship are conceptualized and carried out. Data stewardship and governance principles and policies based on these principles must meet the challenges posed by technology-enabled health data (Hripcsak et al., 2014). To achieve these goals, consent policy should ensure that individuals understand the need to reuse data for population health management and research purposes. Such a policy should ensure transparency in health data use and promote understanding and acceptance of the practices (Hripcsak et al., 2014). Accountability policy should ensure that health systems, governmental bodies, and researchers follow best practices when reusing data of individuals. Privacy and security policy should protect individuals from identity theft and breach of data privacy that compromises privacy and ability to access and use care. Finally, data de-identification techniques should be employed to protect data and reduce the potential for re-identification (Hripcsak et al., 2014). Informatics tools and techniques enable the development and use of interoperable information systems for public health and population health management functions as described in Part I (United States Office of the National Coordinator [ONC], 2015). The consistent, universal use of terminology standards (e.g., SNOMED-CT, LOINC) and process standards (e.g., HL7, FHIR) is the basis of data interoperability and exchange (Overhage & Dixon, 2018). Use of Standards in Clinical Data for PopHI and PHI. Use of standards across levels from clinical to population enables aggregation and population-level analysis for various units and jurisdictions (Overhage & Dixon, 2018). In other words, we can use standardized data from individual clinical care to describe the health of populations. These required standards are discussed at length in other chapters. In addition, other chapters describe the decadeslong efforts of nurse informaticians who developed the infrastructure for standardized nursing data including minimum data sets and terminologies (ANA, 2012). These important tools apply to PopHI as well, and provide a voice in clinical data for nursing and the individuals they serve (Monsen, Bekemeier, Newhouse, & Scutchfield, 2012). Some standards that are specific to interoperability and exchange in PHI have been developed, mapped, and managed by the US CDC. The CDC Public Health Information Network (PHIN) Vocabulary Access and Distribution System (VADS) is a Web-based enterprise vocabulary system for accessing, searching, and distributing vocabularies used in public health and clinical care practice, promoting consistent use of vocabularies for exchange (CDC, 2018a). It consists of 206 code systems, 1506 value sets, 77 views, and more than 5 million concepts. Inclusion in PHIN VADS is based on recommendations from the Health Information Technology Standards Panel (HITSP) C80 specifications (Healthcare Information Technology Standards Panel [HITSP], 2008). The main purpose of PHIN VADS is to distribute the value sets associated with HL7 message implementation guides (CDC, 2018a). First published in 2011, the CDC/CSTE Electronic Laboratory Reporting (ELR) Task Force Reportable Conditions Mapping Table (RCMT) provides mappings between reportable conditions and their associated LOINC laboratory tests and SNOMED results. RCMT enables identification of ELR messages related to reportable conditions and helps route ELR messages from clinics and laboratories to appropriate destinations (e.g., diseasespecific programs of the CDC) (CDC, 2015a). Specific to vaccine surveillance, the U.S. CDC National Center of Immunization and Respiratory Diseases Immunization Information System Support Branch (IISSB) developed and maintains the CVX (vaccine administered) code set. CVX codes indicate the vaccination product (e.g., MMR=CVX 03). MVX codes indicate the vaccine manufacturer. These codes are intended for use in HL7 data transmission, and when aggregated can be used to understand vaccine coverage for populations (CDC, 2019a). Policy to Support Use of Standards.
32
Population Health Informatics
PART I: INTRODUCTION TO POPULATION HEALTH INFORMATICS
History and Origins of Population Health Informatics
Informatics Applications to Improve Population Health
Interprofessionality
Population Health Informatics Infrastructure
PART II: ETHICAL FOUNDATIONS OF POPULATION HEALTH INFORMATICS
History and Origins: Moral, Ethical, and Legal Perspectives
PART III: INFORMATICS TOOLS AND TECHNIQUES FOR POPULATION HEALTH
Stay updated, free articles. Join our Telegram channel

Full access? Get Clinical Tree
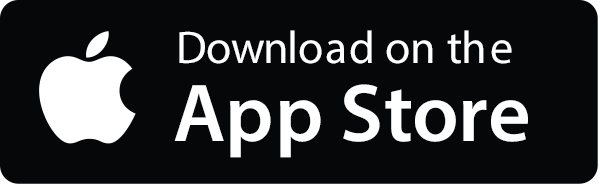
