Over the past two decades, governments and healthcare organizations in the developed countries of the world have invested heavily in the acquisition and deployment of health information technologies, particularly electronic health records (EHRs). In North America, nurses are typically the largest group of health professionals; hence they are also the predominant users of these systems and contributors of clinical data. To optimally leverage the investments both to date and going forward, nurses and others need to begin to utilize technology, informatics, and data science methods to mine evolving data repositories and extract practice-based evidence. In conjunction with classic approaches to research, practice-based evidence, generated and accessible to nurses in real time, has the potential to be a major game changer for the delivery of nursing care. The generation of evidence from data derived from practice will expand nursing’s knowledge base; demonstrate the impact of nursing care on outcomes, clinical and financial; inform the delivery of appropriate, safe, quality patient care; inform health policy directions; and support the appropriate allocation and mix of skilled nursing resources. However, the realization of these goals is largely dependent upon the adoption of clinical nursing data standards in all clinical settings (e.g., acute care, primary care, long-term care, home care). With few exceptions, most countries, including Canada, continue to strategize and strive for this reality. In this chapter, we discuss the opportunity to optimize quality outcomes with the adoption of clinical data standards that reflect nursing practice, particularly with the emergence of “big data” and “data science” methods. Further, examples from the Canadian context are presented as illustrations of the promise and possibilities to be realized from mining the data that richly depicts realms of nursing practice. In 1992, nurses in Canada reached consensus on the data elements required to understand the impact of nursing practice including client status, nursing interventions, and client outcomes. In addition to these clinical data, nurses in Canada identified the need for unique nurse identifiers and nursing resource intensity information to represent nursing practice in the healthcare system (Canadian Nurses Association, 1993). While there has been progress in identifying, defining, and standardizing nursing data, these data are still not consistently collected or widely integrated into EHRs. In addition, these data are not captured within administrative systems nor abstracted into key data repositories. However, national endorsement of data and documentation standards such as the interRAI assessment tools, Systematized Nomenclature of Medicine—Clinical Terms (SNOMED-CT), Logical Observation Identifiers Names and Codes (LOINC), and the International Classification of Nursing Practice (ICNP) have set the stage for the adoption of standards more broadly. In the Canadian context, nursing-specific initiatives such as the Canadian Health Outcomes for Better Information and Care (C-HOBIC) (Hannah, White, Nagle, & Pringle, 2009) and the National Nursing Quality Report for Canada (NNQR-C) (VanDeVelde, Doran, & Jeffs, 2015) have demonstrated the value of standardizing the collection of nursing data within specific jurisdictions and healthcare organizations. But currently, regardless of system vendor, the opportunity to adopt standardized models, tools, and measures is being lost with every healthcare organization that adopts its own approach. Ironically, the potential to design standardized data repositories and reporting tools is one of the greatest advantages of using EHRs, yet this has not been widely addressed by nursing or other health professions. With an aging demographic, increased incidence of chronic disease and demands for valueand outcomesdriven care (Australian Institute of Health and Welfare, 2018; Veillard, Fekri, Dhalla, & Klazinga, 2015), optimally leveraging technology and clinical data collection is an imperative. Collecting standardized information that supports continuity, care coordination, and the evaluation of outcomes (clinical and financial) as people transition across healthcare sectors has the potential to inform and transform health policy. In light of the recent global COVID-19 pandemic, there is no doubt that the capacity to consistently measure and track clinical outcomes within communities and institutions is critical to the effective management of such a crisis; if not globally, at the very least, nationally. Numerous efforts have been made to bring evidence to nurses in practice settings and support nurses to actually use the information they are gathering when making clinical decisions. The use of best practice guidelines/pathways, electronic order sets, smartphone apps (e.g., drug manuals, calculators), point-of-care documentation tools (e.g., barcode readers), plus access to Internet resources can facilitate and support evidence-informed practice. Nurses need to be held to account for taking appropriate clinical action based upon data gathered through the processes of care. Documentation standards should encompass the use of standardized nursing data and evidence-based tools to guide assessment, interventions, clinical decision-making, and outcomes evaluation. Healthcare delivery organizations need to consistently enable and support evidenceinformed practice and administration within and across the healthcare system. Moreover, with the adoption of standardized data and documentation methods, large volumes of comparable clinical data will become available for analysis and study, thereby facilitating the generation of new knowledge and evidence. Indeed, the future advancement of nursing practice will be underpinned by the emerging field of data science. As digital health technologies have permeated all aspects of healthcare over the course of the past several decades, they are now considered to be essential tools for contemporary healthcare and evidence-informed nursing practice. The implementation and adoption of technologies have progressed and matured; hence, attention is shifting from the tactical implementation of digital systems to the strategic use of patient and healthcare data that are captured and stored as a product of system use. Data sources have also proliferated, including EHRs, patient monitoring devices, smart technologies and mobile health applications, social media, diagnostic testing, and clinical assessments—all of which cumulatively generate enormous amounts of data about patients and the healthcare system. This vast, untapped accumulation of information offers unparalleled opportunities to understand more about disease prevention and management, intervention (e.g., symptom management) evaluation, and health system use. However, it demands that we approach this opportunity with new perspectives, new methods and tools, and new ways of conceptualizing health information management. As early as 2001, the challenges of data management arising from the dramatic shifts in e-commerce and social drivers to greater digitization were identified as volume, velocity, and variety (Laney, 2001). Although the term “big data” didn’t emerge until later, the “3Vs” were adopted and embedded in the definition of big data (Gu, Li, Li, & Liang, 2017). Big data is generally accepted as a term used to describe massive data sets that exceed the capability of traditional database management approaches and methodologies to derive meaning from analysis (Brennan & Bakken, 2015; Gu et al., 2017). Although debate persists about the exact definition of “big data,” two additional distinct characteristics were introduced, ultimately contributing the additional characteristics veracity and value (Westra et al., 2017). There is broad acceptance of the 5 Vs of big data and current definitions of big data routinely cite the 5 Vs (Westra et al., 2017). Volume refers to the sheer scale of data generated by a variety of sources—and many consider this to be the key hallmark of big data (Gu et al., 2017; Westra et al., 2017). Industry research into data proliferation was consolidated by IBM, who projected that 40 zetabytes (ZB) of data will be generated by 2020, reflecting a 300-fold increase over 2005 data volume (IBM, n.d.). Further, IBM reported that 2.5 quintillion bytes (QB) are created on a daily basis. Recently, researchers projected that healthcare data will grow faster than other sectors, at a compound annual rate of 36% through to 2025 (Kent, 2018). Velocity reflects the unparalleled speed of proliferation (Westra et al., 2017). IBM projected that 18.9 billion network connections would exist by 2016 and reported that the New York Stock Exchange captures 1 terabyte (TB) in data during daily data sources (Westra et al., 2017). IBM projected that by 2014, 420 million smart devices would be worn for health monitoring, and digital consultant David Sayce reported that by November 2018, approximately 6000 tweets per second were being sent, totalling 500 million tweets per day and 200 billion per year (Sayce, 2019). Veracity of the data reflects the degree of uncertainty of data elements and whether the data is fit for secondary analysis (Topaz & Pruinelli, 2017; Westra et al., 2017). IBM reported that poor data quality costs the United States in excess of 3 trillion dollars annually, while 30% of managers lack trust in the data used to make decisions and close to 30% survey participants are unable to confirm how much of their data is inaccurate (IBM, n.d.). Value reflects the perceived contribution the data are able to provide to support the organizational mission and objectives. Identification of the 5 Vs has led to a deeper appreciation of the vast, untapped potential of big data and a desire to examine the extent to which this data could be systematically analyzed and leveraged to improve outcomes and healthcare delivery. In their seminal review of nursing data science, Brennan and Bakken (2015) noted that a principled, scientific approach to big data emerged around 2015 to complement the popular big data narrative. “Data science” blends a multidisciplinary approach to data management, including math, computer science, statistics, modeling, predictive analytics, and others, offering greater philosophical and methodological rigor to all phases of the data management cycle (Fig. 41.1). However, diversity in perspectives about nursing data science exists. Broom (2016) defined big data science as a new field in which automated methods are applied to “collect, extract, and analyze” vast amounts of data to answer questions that were previously unanswered. Topaz and Pruinelli (2017) defined data science as the multidisciplinary scholarship approach to working with data and noted that researchers need to recognize how “messy” healthcare data is and be able to determine the optimal method for resolving this and applying appropriate analytic methods such as data mining, artificial intelligence, natural language processing, and visualization. Jeffrey (2019) suggests that data science lies at the convergence of “domain knowledge, computer science, statistics, and data visualization/presentation” and in his consultation of nurse leaders, a variety of perspectives were noted, including that data science is a tool and is naturally a subset of informatics, while others sought to discretely distinguish data science from the scope and knowledge of biomedical informaticists. Jeffrey concluded that data science is simply one of the numerous specialty areas open to informaticists. • FIGURE 41.1. Big Data Management Lifecycle. (Adapted from Brennan, P. F., & Bakken, S. (2015). Nursing needs big data and big data needs nursing. Journal of Nursing Scholarship, 47(5), 477–484. http://dx.doi.org/10.1111/jnu.12159.) Brennan and Bakken (2015) noted that data science investigations typically involve four characteristics including (1) disparate data sources that remain under the governance of the data owner, (2) the application of attribution and security to data, (3) expanded networks of research collaborators sharing approaches and methodologies, and (4) accelerating research insights through the use of secondary data and emphasizing the integration of the data rather than on the actual data itself. Westra et al. (2017) proposed a nursing data science research model (Fig. 41.2) that articulates the core components of big data and data science–driven nursing research. • FIGURE 41.2. (Reproduced, with permission, from Westra, B.L., Sylvia, M., Weinfurter, E.F., Pruinelli, L., Park, J.I., Dodd, D., … Delaney, C. (2017). Big data science: A literature review of nursing research exemplars. Nursing Outlook, 65, 549-561. Copyright © Elsevier.) As the concept of big data gained momentum across all sectors of society, the number of publications started to increase significantly. In their study of the evolution of big data research in health informatics, Gu et al. (2017) applied a bibliometric analysis to track the volume and scope of publications focused on big data. They reviewed almost 2400 studies indexed on the Web of Science (WOS) and published between 2003 and 2016. The analysis documented a staggering increase over time. In 2003, 53 articles on big data were indexed in WOS, which increased gradually until 2013. Between 2013 and 2015, publications more than doubled, rising from 240 to 517. Similarly, they recorded a significant increase in the same timeline in the number of authors exploring big data and health informatics. Analysis of the key words indicated that big data, epidemiology, personality, breast cancer, and data mining formed the top five most common key words. In addition, diabetes was also one of the most popular key words in the international research studies. Further, this research was able to identify the countries and research institutions leading the number of contributions to the big data discourse, ranking the top three contributors as the United States (662 articles), China (235), and the United Kingdom (191). Canada was ranked seventh among 17 countries, with 84 publications indexed on the WOS. The only Canadian institution identified among the top 10 institutions with 20 or more published articles was the University of Toronto, with 21 published articles (Gu et al., 2017). A 2013 vision report on health system use of data in Canada by the Canadian Institute for Health Information (CIHI) (CIHI, 2013) addressed many of the characteristics identified by Brennan and Bakken (2015) and Topaz and Pruinelli (2017), and noted that as health information is generated from a diverse array of sources and is largely unstructured, there is a high need to apply both structured and standardized data to enable codification, integration, and comparability. CIHI’s report also addressed the concepts of governance, privacy and security, technology, data collection, availability and use, and capacity and culture as necessary to fostering the progression of data science and health system use of data. Figure 41.3 highlights the core components of the CIHI framework to support data use for improved health outcomes. • FIGURE 41.3. (Reproduced, with permission, from Canadian Institute for Health Information (CIHI). (2013). Better information for improved health: A vision for health system use of data in Canada. Ottawa: CIHI.) With the broad emphasis on big data, organizations have established dedicated institutes to study this domain and stimulate both innovation and collaboration. For example, Dalhousie University established the Big Data Institute, which regularly hosts conference events that span all sectors. Other examples in Canada that are specifically health focused include the third IoT, Big Data Healthcare Summit Western Canada hosted by the Information Technology Association of Canada (ITAC), and the sixth Annual Big Data & Analytics Summit Canada hosted by Strategy Institute. Additionally, Canada Health Infoway (Infoway, 2019) is seeking funding to create an investment in digital health data platforms for Canada’s research hospitals and academic health sciences centers. HealthCareCAN (2019) will consult with Infoway given its expertise in the development, adoption, and effective use of digital health solutions across the country. Broom (2016) disputes the view that big data science or nursing data science will replace traditional research methodologies and suggests that the focus should be on ensuring that the profession is adequately and appropriately preparing future nurse researchers and leaders. This concern is shared by numerous authors and nursing leaders who address the competency needs for future nursing data scientists and leaders (Brennan & Bakken, 2015; Jeffrey, 2019; Topaz & Pruinelli, 2017; Westra et al., 2017). Competency in advanced statistics, data modeling, visualization, data mining, and other advanced data management techniques will be required to position nursing to continue to advance knowledge using emerging vast data sets. An example of this type of strategic competency development is occurring within the University of Victoria in Canada, where nursing students can complete a dual master’s degree in nursing and computer science, building skills to manage data and systems in addition to advanced nursing scholarship. Other programs include the Masters of Health Informatics at Dalhousie University and the University of Toronto, Western University’s Master of Data Analytics, and McGill’s Data Science. Nurses are investing considerable time documenting and capturing care data with the use of a variety of technologies (e.g., EHRs, smart devices, remote monitoring). Nonetheless they rarely receive any real-time evaluative feedback, reports, or outcome analysis outputs to further inform, revise, or refine their practice (Jeffrey, 2019; Westra et al., 2015). Westra et al. (2015) refer to this phenomenon as being “data rich and information poor” (DRIP). Increasingly, studies are using data science to explore practice outcomes to optimize clinical pathways and inform practice-based evidence. However, until technologies such as natural language processing become pervasive and refined for understanding complex practices like nursing, the promise of big data and the application of data science methods will be limited. Realizing the possibilities to be garnered from the application of data science largely rests with the ability to capture and share data that are comparable and shareable; that is, data and measures that are consistently used throughout the healthcare system—clinical data standards. While significant investments have been made within every jurisdiction in Canada for technology to create efficiency and improve health for Canadians, similar to other countries, healthcare organizations across Canada are in varying states of maturity related to EHRs and the integration of clinical data standards. In Canada, two national organizations are providing leadership in these areas. CIHI is a national organization with a mandate to deliver comparable and actionable information to accelerate improvements in healthcare, health system performance, and population health across the continuum of care (for more information see: https://www.cihi.ca/en/about-cihi/vision-and-mandate). CIHI collects clinical and administrative data from healthcare organizations and makes this information available to organizations, researchers, and decision-makers to examine and compare the delivery of health services and inform public health policy. A partnership exists between CIHI and interRAI, a research network committed to developing clinical standards across a variety of health and social services settings (For additional information see: http://www.interrai.org/organization/). CIHI serves as the custodian of the interRAI standards and as a repository of interRAI data submitted by healthcare organizations. Although much of the focus in Canada has been on organizations submitting interRAI data for home care, continuing care, and inpatient and community mental health, recently there has been recognition of the need for standardized clinical data from acute care. As part of the Discharge Abstract Database (DAD), CIHI currently collects information on all separations from acute care institutions, including demographics, diagnosis, comorbidities, discharges, deaths, and so on. The collection of clinical data from acute care to link with data from other sectors would facilitate local to national comparisons about clinical outcomes. Furthermore, the collection of a standardized suite of essential clinical information across all sectors of the healthcare system would allow for examining a person’s healthcare across settings and sectors, supporting continuity of care and improved health outcomes. Canada Health Infoway is a national organization with the goal of helping to improve the health of Canadians by working with partner organizations to accelerate the development, adoption, and effective use of digital health solutions across Canada (for more information see: https://infoway-inforoute.ca/en). Through national and provincial investments, Infoway plays a leadership role in helping to deliver better quality and access to care and more efficient delivery of health services for patients and clinicians. Infoway’s ACCESS 2022 is a new program focused on providing health information access to Canadians so that they are better informed to manage their health (for more information see: https://www.infoway-inforoute.ca/en/solutions/access-health). Infoway is also a source of interoperability standards (including data standards) and through investments plays a leadership role in helping to deliver better quality and access to care and more efficient delivery of health services for patients and clinicians. Infoway provides an online community (InfoCentral) for nursing and other disciplines to discuss and share experiences and learnings in the use of clinical data standards across Canada (for more information see: https://infocentral.infoway-inforoute.ca/en/). Harrington proposes that the ultimate benefit of the time, energy, and investments in EHRs is clinical intelligence whereby there is “aggregation of accurate, relevant and timely clinical data into meaningful information and actionable knowledge for clinicians and decision makers” (Harrington, 2011). Matney et al. (2017) argued that the standardization of healthcare data is essential for shareable and comparable data. It is only through standardization of clinical data and the ability to collect data once and use it for many purposes that we will be able to truly realize the value of investments made in EHRs (see Fig. 41.4) (Nagle & White, 2015). • FIGURE 41.4. The Vision: Data Collected Once for Many Purposes. (From Nagle, L. M., & White, P. A. (2015). Towards a pan-Canadian strategy for nursing data standards. https://infocentral.infoway-inforoute.ca/en/resources/docs/2188nursing-informatics-vision/view-document. Used with permission) O’Brien, Weaver, Settergren, Hook, and Ivory (2015) discuss the need to optimize nurses’ documentation efficiency while contributing to knowledge generation. Much of current nursing practice is evidence-based— nurses using the best available evidence to inform their practice; however, there can be gaps in existing evidence. Furthermore, nurses may have challenges in accessing current research studies. Electronic access to large amounts of data in a standardized format presents a great opportunity for creating new knowledge for the nursing profession. The use of clinical data standards in EHRs can facilitate practice-based evidence, whereby data about patient age, diagnoses, interventions, and outcomes are captured in the EHR and can be analyzed to support current individualized clinical practice (Miles & Loughlin, 2011). This will allow clinicians to learn from past patients with similar diagnoses about what interventions produced better clinical outcomes. Standardizing clinical data will benefit patients as they move through the healthcare system, making transitions and information sharing of health information seamless, and enhancing continuity of care and information. As an example, Canadians with chronic obstructive pulmonary disease are high users of healthcare (https://www.cihi.ca/en/copd-a-focus-on-high-users). Standardizing clinical data such as functional status, dyspnea, and fatigue and sharing this information across providers and settings will support better management of health care (White, 2016). The collection of standardized clinical data allows clinicians to visualize the flow sheet of essential data and identify trends, and compare current assessment in acute care to previous assessments such as previous acute care or home care admissions and trend assessment information over time and across settings to support practice decisions. If trends show that dyspnea consistently deteriorates on discharge then clinicians need to ask what can be changed? Is there an intervention that could be added in the home care sector to maintain or improve the patient’s dyspnea? Subsequently, the capacity to aggregate the data of hundreds of similar patients would provide insights to the effectiveness of care interventions, which profession is best suited to deliver the care (e.g., nurse or respiratory therapist), and in which setting. But access to shareable, comparable data is not a possibility without the adoption of clinical data standards—at the very least, an agreed-upon essential clinical data set identifiable in any and every setting. Canadian Health Outcomes for Better Information and Care (C-HOBIC) is a Canadian initiative to advance the uptake and use of a suite of standardized nursing-sensitive patient outcomes in acute care settings (Hannah et al., 2009) (for more information see: https://c-hobic.cna-aiic.ca/about/default_e.aspx). This suite of evidence-based clinical measures includes functional status, continence, symptoms (pain, fatigue, nausea, dyspnea), falls, pressure ulcers, and therapeutic self-care (TSC) (Doran, 2012). These concepts are assessed using the C-HOBIC and interRAI measures and they are harmonized across sectors to support sharing and comparing of clinical information across sectors of the healthcare system (see Table 41.1). TABLE 41.1. Use of C-HOBIC Tools/Measures in Different Care Sectors.
41
Nursing Data Science and Quality Clinical Outcomes
INTRODUCTION
BACKGROUND
THE EMERGENCE OF NURSING DATA SCIENCE
TRENDS IN BIG DATA AND NURSING DATA SCIENCE
NURSING DATA SCIENCE IN CANADA
ADOPTING CLINICAL DATA STANDARDS—THE BENEFITS TO BE REALIZED
FROM EVIDENCE-BASED PRACTICE TO PRACTICE-BASED EVIDENCE
THE EVIDENCE UNTIL NOW
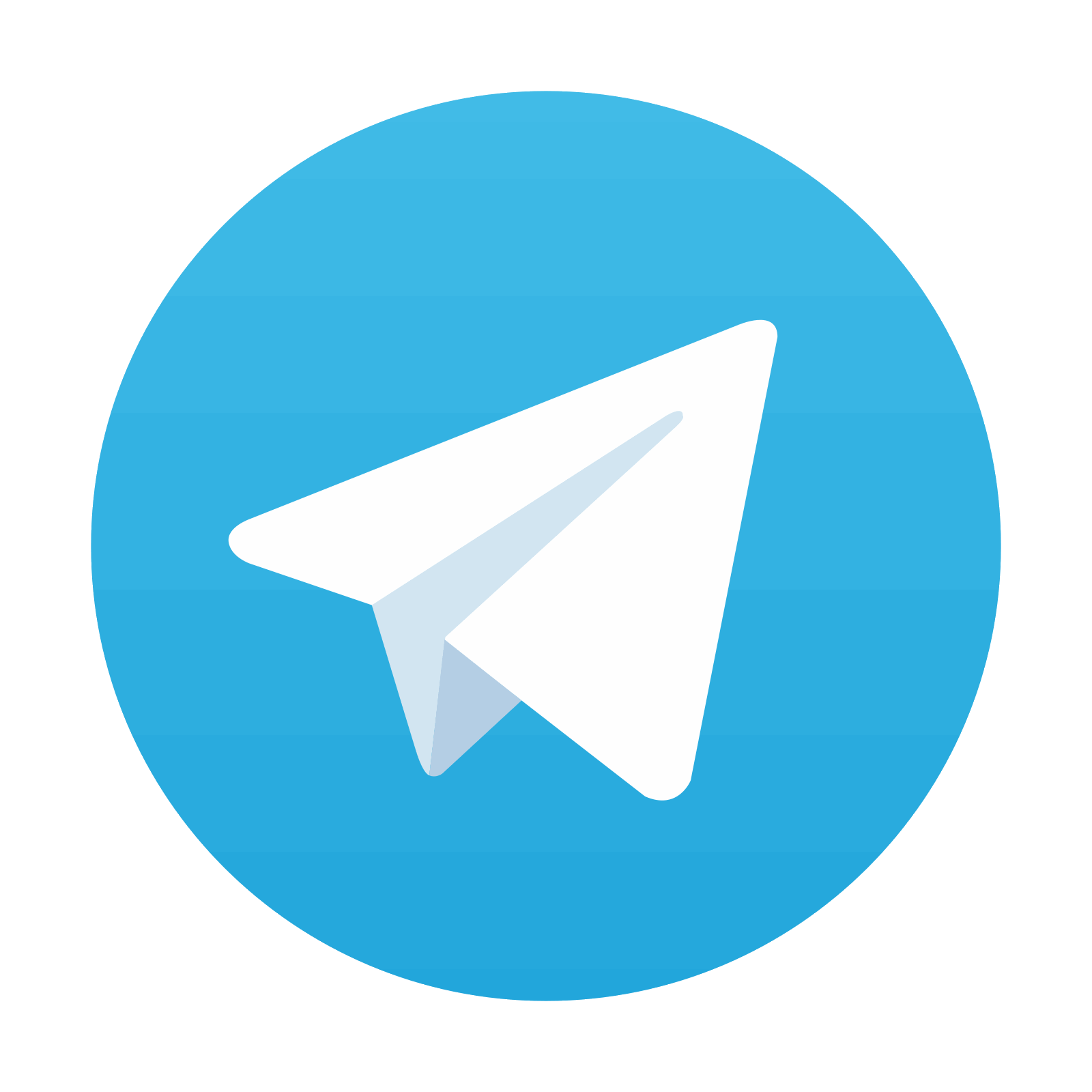
Stay updated, free articles. Join our Telegram channel

Full access? Get Clinical Tree
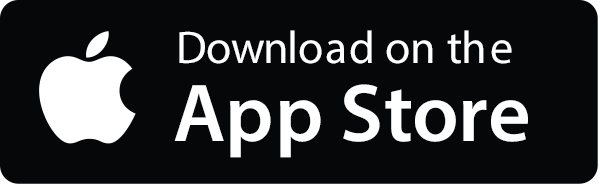
