html xmlns=”http://www.w3.org/1999/xhtml”>
14
Cause and Effect, Hypothesis Testing and Estimation
Key points
- Assertions about cause and effect are probabilistic, not definitive.
- We make cause-effect predictions in daily life.
- Cause-effect predictions in healthcare research are the same as those in daily life.
- Quantitative researchers are concerned with independent variables, dependent variables and intervening variables.
- Researchers manipulate independent variables, observe any changes to dependent variables and attempt to account for intervening variables.
- Hypotheses are explicit statements of the predicted relationship between independent and dependent variables.
- Directional hypotheses are made only when there is reason to think a relationship operates only in one direction.
- Adequate statistical power is essential to the safe acceptance of the null hypothesis.
- Hypothesis testing is an all-or-nothing statement of related- ness, but estimation emphasises the extent of a relationship.
Introduction
The examination of cause and effect is one of the most misunderstood concepts in healthcare research. It has been characterised, variously as mechanistic, unrealistic and over-simple. However, all these assertions about examining cause and effect are themselves based on a version of cause and effect testing and the methods employed to do it (most notably, the randomised controlled trial) which are quite different from those actually practiced by quantitative researchers. Specifically, those who reject methods which seek to establish cause and effect usually do so because they assume that quantitative researchers are claiming the right to a single, definitive answer.
However, this is not so. In fact, quantitative researchers are at pains, in their writing, their research methods and even their statistical procedures (which are all about probability not fact) to recognise that their answers to cause and effect questions are always simply the most likely answer given the current state of our knowledge. It is explicitly acknowledged that many different factors influence cause and effect relationships, and that not all of them can be controlled or even identified. The best that can be achieved is to be as careful as possible in taking account of as many factors that we can control or perceive. As a consequence, it is never claimed that a certain intervention (say, a certain wound care procedure) is always the approach of choice for a particular patient problem (a pressure ulcer), only that it is most likely to be effective. The habit, amongst many researchers and clinicians, of describing a particular intervention as effective is merely a shorthand for this expression of a likely relationship between a given cause (e.g. treatment) and effect (e.g. patient improvement). This habit has probably given rise to considerable misunderstanding of the nature of scientific ‘fact’ in quantitative research.
Issues of cause and effect are never definitively answered
Describing a treatment as effective is a shorthand for saying it is most likely to be effective, given the current state of our knowledge. It is always acknowledged that there are many influencing factors that cannot be known.
Cause and effect relationships in daily life
Nevertheless, quantitative researchers do say that we live in a cause-effect world. With all the qualifications noted above, it really is contended that, by and large, doing certain things has consequences for patient care and that these can be reliably demonstrated time and time again. However, this is not a stance which is peculiar to quantitative researchers in particular, or even to researchers in general. In Chapter 6, we showed how sampling is a normal everyday activity carried out by all of us. Exactly the same is true for the assessment of cause and effect relationships. Awareness of cause and effect is basic to human life, and, arguably, to the survival of all life. For example, at the level of the other animal species, it is well demonstrated that organisms recognise and avoid aversive stimuli (e.g. pain, loud noises). This is a simple recognition of cause and effect relationships – performing such and such an activity leads to (causes) pain or discomfort and is therefore best avoided.
In our own lives, we make such decisions all the time. Every morning I open my window and look at the sky. If it is cloudy, I predict that it will rain and take an umbrella with me to the station. In doing so, I am making a cause-effect prediction: ‘The presence of dark clouds results in rain’. Now, strictly speaking, clouds do not cause rain, but I am unaware of the actual processes which do and would, in any case, be unable to measure them without sophisticated equipment. This does not matter, because it is merely an issue of measurement. From my point of view, I have repeatedly tested the assertion that certain kinds of cloudy skies are followed by rain, and this had led to my making the cause-effect link. Nor does it matter if, on a particular occasion, I am proved wrong. What matters is that, most of the time, this cause-effect relationship holds true. Indeed, if it did not, I would soon stop making this prediction and stop carrying my umbrella. I would then be responding to a criterion I had set for myself according to which I would regard my prediction as having been verified or falsified. This brings us again to the notion that cause and effect relationships are almost always probabilistic. I also will try to take into account other aspects of the weather (did it rain yesterday, what kind of shape are the clouds, how dark are they, what is the temperature) which, according to my level of knowledge, I will include in my assessment of the likelihood of rain today. This leads us to the assertion that cause and effect predictions are complex. This is recognised just as much in research as it is in this everyday example, and we certainly do not expect healthcare research to be less complicated than our everyday decision making.
Cause-effect is part of daily life
In everyday life, we make cause-effect predictions all the time. In doing this, we recognise that we are making probability predictions. Often, we take many complex issues into consideration in making such predictions in important as well as mundane situations. Strangely, we have no problem in doing so – only when this kind of decision is applied to our research or clinical work do people see this as problematic.
Examining cause and effect in healthcare
Therefore, the kinds of cause and effect predictions we make in healthcare can be just as rich, probabilistic and complex as those we make intuitively in everyday life. The methods used to tease out these complexities are often painstaking and complex because, in research, we want to ensure that the processes we have used to arrive at our decisions are transparent to all, in a way which we do not have to do with our own everyday decisions. We want to do this so that others can scrutinise and criticise our work with a clear understanding of what we have done and why we have done it. If necessary, we want to attempt to replicate our work (repeat it using exactly the same methods in a different context). This has given rise to a formal language, which is perhaps unfortunate, but is no different than the verbal shorthand health professionals use to communicate rapidly with each other. The actual processes are little different from the way in which we, as a society, have arrived at our shared knowledge that dark clouds are usually followed by rain.
Variables
In asserting and testing cause-effect relationships, we are basically describing and predicting relationships between variables. Variables are any entities in the world which are not fixed, but are subject to change. There are three types of variables to concern us: independent variables, dependent variables and intervening variables. The independent variable is the thing which we, as researchers, vary or manipulate in some way. Usually, this is a treatment of some sort. So, if we give one group of patients a relaxation exercise prior to surgery and give another group a premedication sedative, this difference in treatment is the independent variable. It is said to vary over two levels (relaxation and pre- medication). If we introduce a further group (treatment as normal), the independent variable will then vary over three levels. The use of the word ‘level’ does not, however, imply that one treatment is more valuable than another, and the term experimental condition is sometimes used interchangeably with level. Thus, relaxation, premedication and treatment as normal are three different experimental conditions in the above example.
Levels and conditions – the same, but different
Strictly speaking, levels and conditions are not the same. For example, if we tested two interacting independent variables (exercise regime and intensity of exercise), we could have several levels of each variable: regime (running versus circuit training); intensity (half an hour daily versus an hour every 2 days). So, each variable here consists of two levels. There are four conditions (half an hour daily running, hour bi daily running, half an hour daily circuit, hour bi daily circuit). So, levels refer only to different conditions within a single variable, whilst conditions can be used to describe levels across conditions! Remember, all this is just jargon. It is useful to know the language, so that you can better understand written reports, but it does not materially affect the actual conducting of research.
By contrast with the independent variable, the dependent variable is not manipulated by the researcher. Instead, changes in this variable are regarded as being dependent on the action of the independent variable. In the example above, one possible dependent variable might be patient anxiety, and we would expect the amount of anxiety to differ between the three levels of the independent variable. If this turned out to be the case, we would conclude that these differences were the result of or, dependent upon, the actions of the different levels of the independent variable. This is the essence of the investigation of cause-effect relationships in quantitative research.
The relationship between independent and dependent variables
Changes which we measure in the dependent variable are regarded as the result of (dependent on) our manipulations of the independent variable. In clinical practice, this is like concluding that changes in patient well-being are the result of treatment.
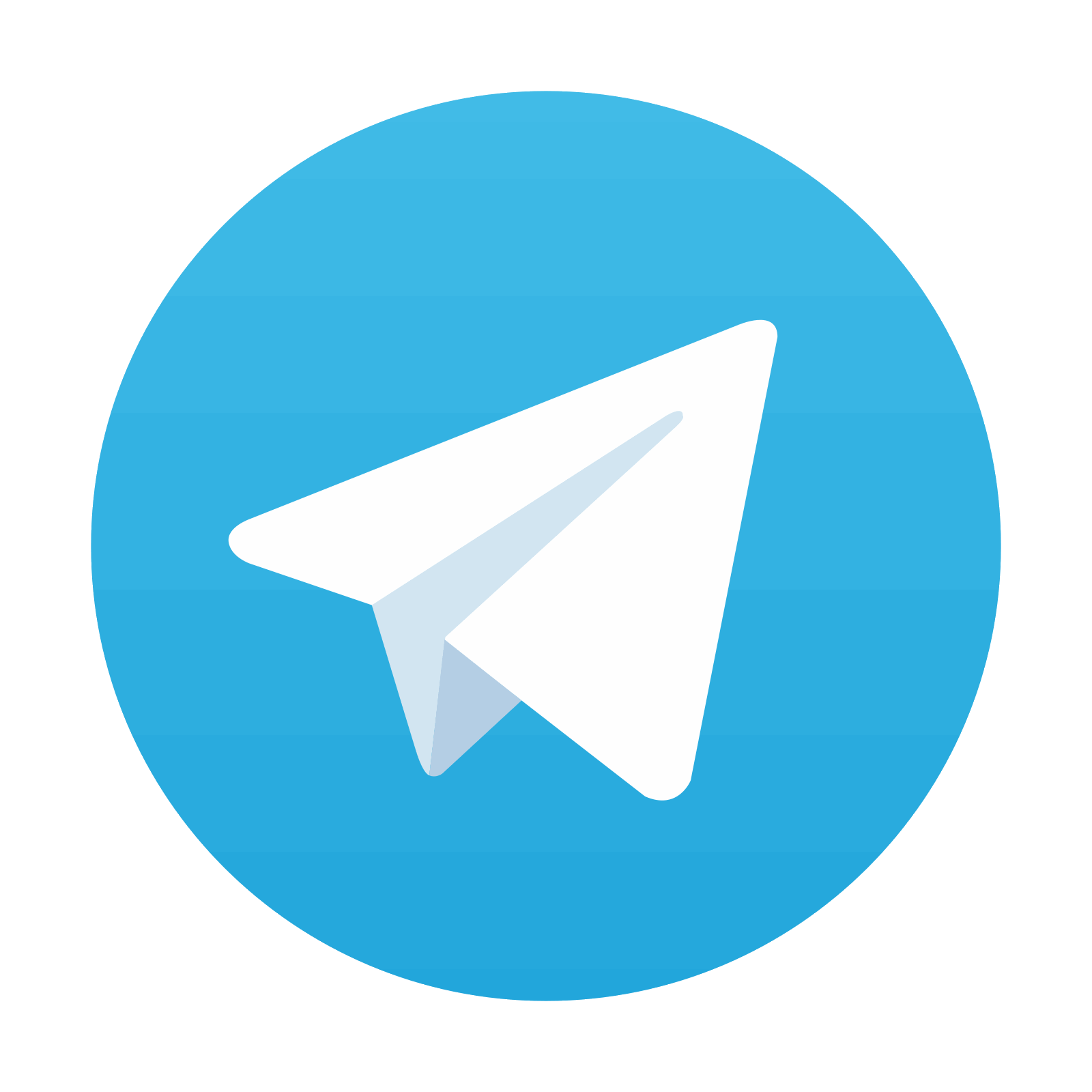
Stay updated, free articles. Join our Telegram channel

Full access? Get Clinical Tree
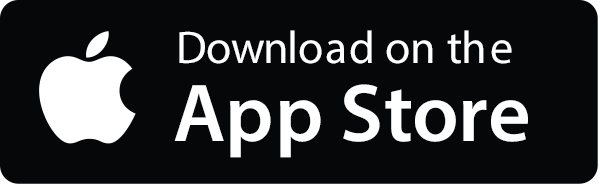
