Epidemiology
Holly B. Cassells
Objectives
Upon completion of this chapter, the reader will be able to do the following:
1. Identify epidemiological models used to explain disease and health patterns in populations.
2. Use epidemiological methods to describe the state of health in a community or aggregate.
3. Calculate epidemiological rates in order to characterize population health.
4. Understand the use of epidemiological methods in primary, secondary, and tertiary prevention.
5. Evaluate epidemiological study designs for researching health problems.
Key terms
age-adjustment of rates
age-specific rates
analytic epidemiology
attack rates
cause-and-effect relationship
crude rates
descriptive epidemiology
ecosocial epidemiology
epidemiological triangle
epidemiology
incidence rates
morbidity rates
mortality rates
natural history of disease
person-place-time model
prevalence rate
proportionate mortality ratio
rates
risk
risk factor
screening programs
standardization of rates
surveillance
web of causation
Additional Material for Study, Review, and Further Exploration
Epidemiology is the study of the distribution and determinants of health and disease in human populations (Harkness, 1995) and is the principal science of community health practice. It entails a body of knowledge derived from epidemiological research and specialized epidemiological methods and approaches to scientific research. Community health nurses use epidemiological concepts to improve the health of population groups by identifying risk factors and optimal approaches that reduce disease risk. Epidemiological methods are important for accurate community assessment and diagnosis and in planning and evaluating effective community interventions. This chapter discusses the uses of epidemiology and its specialized methodologies.
Use of epidemiology in disease control and prevention
Although epidemiology originated in ancient times, formal epidemiological techniques developed in the nineteenth century. Early applications focused on identifying factors associated with infectious diseases and the epidemic spread of disease in the community. Public health practitioners hoped to improve preventive strategies by identifying critical factors in disease development.
Specifically, investigators attempted to identify characteristics of people who had a disease such as cholera or plague and compared them with characteristics of those who remained healthy. These differences might include a broad range of personal factors such as age, gender, socioeconomic status, and health status. Investigators also questioned whether there were differences in the location or living environment of ill people, compared with healthy individuals, and whether these factors influenced disease development. Finally, researchers examined whether common time factors existed (i.e., when people acquired disease). Use of this person-place-time model organized epidemiologists’ investigations of the disease pattern in the community (Box 5-1). This study of the amount and distribution of disease constitutes descriptive epidemiology. Identified patterns frequently indicate possible causes of disease that epidemiologists can examine with more advanced epidemiological methods.
In addition to investigating the person, place, and time factors related to disease, epidemiologists examine complex relationships among the many determinants of disease. This investigation of the causes of disease, or etiology, is called analytic epidemiology.
Even before the identification of bacterial agents, public health practitioners recognized that single factors were insufficient to cause disease. For example, while exploring the cholera epidemics in London in 1855, Dr. John Snow collected data about social and physical environmental conditions that might favor disease development. He specifically examined the contamination of local water systems. Snow also gathered information about people who became ill; this included their living patterns, their water sources, their socioeconomic characteristics, and their health status. A comprehensive database helped him develop a theory about the possible cause of the epidemic. Snow suspected that a single biological agent was responsible for the cholera infection, although the organism, Vibrio cholerae, was undiscovered. He compared the death rates among individuals using one source of water with those among people using a different water source. This suggested an association between cholera and water quality.
The epidemiologist examines the interrelationships between host and environmental characteristics and uses an organized method of inquiry to derive an explanation of disease. This model of investigation is called the epidemiological triangle because the epidemiologist must analyze the following three elements: agent, host, and environment (Figure 5-1). The development of disease is dependent on the extent of the host’s exposure to an agent, the strength or virulence of the agent, and the host’s genetic or immunological susceptibility. Disease is also dependent on the environmental conditions existing at the time of exposure, which include the biological, social, political, and physical environment (Table 5-1). The model implies that the rate of disease will change when the balance among these three factors is altered. By examining each of the three elements, a community health nurse can methodically assess a health problem, determine protective factors, and evaluate the factors that make the host vulnerable to disease.
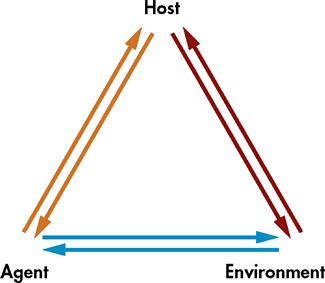
TABLE 5-1
Modified from Lilienfeld DE, Stoley PD: Foundations of epidemiology, New York, 1994, Oxford University Press.
Conditions linked to clearly identifiable agents such as bacteria, chemicals, toxins, and other exposure factors are readily explained by the epidemiological triangle. However, other models that stress the multiplicity of host and environmental interactions have developed, and understanding of disease has progressed. The “wheel model” is an example of such a model (Figure 5-2). The wheel consists of a hub that represents the host and its human characteristics such as genetic makeup, personality, and immunity. The surrounding wheel represents the environment and comprises biological, social, and physical dimensions. The relative size of each component in the wheel depends on the health problem. A relatively large genetic core represents health conditions associated with heredity. Origins of other health conditions may be more dependent on environmental factors (Mausner and Kramer, 1985). This model subscribes to multiple-causation rather than single-causation disease theory; therefore it is more useful for analyzing complex chronic conditions and identifying factors that are amenable to intervention.
Following the discovery of the causative agents of many infectious diseases, public health interventions eventually resulted in a decline in widespread epidemic mortality, particularly in developed countries. This caused the focus of public health to shift to chronic diseases such as cancer, coronary heart disease, and diabetes during the past few decades. These chronic diseases tend to have multiple interrelated factors associated with their development rather than a single causative agent.
In studying chronic diseases, epidemiologists use methods that are similar to those used in infectious disease investigation, thereby developing theories about chronic disease control. Risk factor identification is of particular importance to chronic disease reduction. Risk factors are variables that increase the rate of disease in people who have them (e.g., a genetic predisposition) or in people exposed to them (e.g., an infectious agent or a diet high in saturated fat). Therefore their identification is critical to identifying specific prevention and intervention approaches that effectively and efficiently reduce chronic disease morbidity and mortality. For example, the identification of cardiovascular disease risk factors has suggested a number of lifestyle modifications that could reduce the morbidity risk before disease onset. Primary prevention strategies, such as dietary saturated fat reduction, smoking cessation, and hypertension control, were developed in response to previous epidemiological studies that identified these risk factors (Box 5-2). The web of causation model illustrates the complexity of relationships among causal variables for heart disease (Figure 5-3).
A newer paradigm, ecosocial epidemiology, challenges both the more individually focused risk factor approach to understanding disease origins and the growing interest in molecular epidemiology, exemplified by the sequencing of genes to determine individual susceptibility to various diseases (MacDonald, 2004). This ecological approach emphasizes the role of evolving macro-level socioenvironmental factors, including complex political and economic forces along with microbiological processes, in understanding health and illness. Investigating the context of health will necessitate alternative research approaches, such as qualitative and ecological studies and studies of social institutions and processes. In turn, the examination of social and contextual origins will enlighten the interventions of public health practitioners.
For example, Buffardi and colleagues (2008) analyzed the ecosocial and psychosocial correlates of diagnosis of sexually transmitted infections (STIs) among young adults. Specifically, they examined STI diagnosis within “contextual conditions” including low income, “housing insecurity,” childhood physical or sexual abuse, intimate partner abuse, gang participation, personal history of having been arrested, and drug/alcohol use. It was determined that STIs were statistically associated with housing insecurity, exposure to crime, and having been arrested. Additionally, nearly all of the contextual conditions predicted more lifetime sexual partners and earlier initiation into sexual behavior. The researchers concluded that ecosocial or contextual conditions strongly enhance STI risk by increasing sexual risk behaviors and likelihood of exposure to infection.
In another study, Campbell and others (2009) developed a “comprehensive model” for late-stage diagnosis of breast cancer using data from the national cancer registry. The researchers considered variables of socioeconomic status and poverty indicators, race/ethnicity, and geographical residence (address). They determined that minority and poor women experience more frequent advanced breast cancer at diagnosis. Indeed, both black and Hispanic women are at greater risk for regional and distant-stage diagnosis of breast cancer than white women, and poverty, regardless of race/ethnicity, was strongly associated with the probability of the diagnosis being made at later stages. A final note from the study was that it was important for all researchers to examine health disparities within a “well-defined medical care catchment area.”
Calculation of rates
The community health nurse must analyze data about the health of the community to determine the pattern of disease in a community. The nurse may collect data by conducting surveys or compiling data from existing records (e.g., data from clinic facilities or vital statistics records). Assessment data often are in the form of counts or simple frequencies of events (e.g., the number of people with a specific health condition). Community health practitioners interpret these raw counts by transforming them into rates.
Rates are arithmetic expressions that help practitioners consider a count of an event relative to the size of the population from which it is extracted (e.g., the population at risk). Rates are population proportions or fractions in which the numerator is the number of events occurring in a specified period of time. The denominator consists of those in the population at the specified time period (e.g., per day, per week, or per year). This proportion is multiplied by a constant (k) that is a multiple of 10, such as 1000, 10,000, or 100,000. The constant usually converts the resultant number to a whole number, which is larger and easier to interpret. Thus, a rate can be the number of cases of a disease occurring for every 1000, 10,000, or 100,000 people in the population.
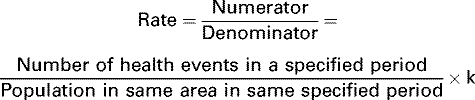
When raw counts are converted to rates, the community health nurse can make meaningful comparisons with rates from other cities, counties, districts, or states; from the nation; and from previous time periods. These analyses assist the nurse in determining the magnitude of a public health problem in a given area and allow more reliable tracking of trends in the community over time (Box 5-3).
Sometimes a ratio is used to express a relationship between two variables. A ratio is obtained by dividing one quantity by another, and the numerator is not necessarily part of the denominator. For example, a ratio could contrast the number of male births to that of female births. Proportions can describe characteristics of a population. A proportion is often a percentage, and it represents the numerator as part of the denominator.
Morbidity: Incidence and Prevalence Rates
The two principal types of morbidity rates, or rates of illness, in public health are incidence rates and prevalence rates. Incidence rates describe the occurrence of new cases of a disease (e.g., tuberculosis, influenza) or condition (e.g., teen pregnancy) in a community over a period of time relative to the size of the population at risk for that disease or condition during that same time period. The denominator consists of only those at risk for the disease or condition; therefore, known cases or those not susceptible (e.g., those immunized against a disease) are subtracted from the total population (Table 5-2).

TABLE 5-2
Morbidity Rates | Crude Death Rate | Specific Rates |
Incidence Rate![]() | Crude Rates![]() | Infant Mortality Rate![]() |
Prevalence Rate![]() | Crude Birth Rate![]() | Fertility Rate![]() |
The incidence rate may be the most sensitive indicator of the changing health of a community because it captures the fluctuations of disease in a population. Although incidence rates are valuable for monitoring trends in chronic disease, they are particularly useful for detecting short-term acute disease changes—such as those that occur with infectious hepatitis or measles—when the duration of the disease is typically short.
If a population is exposed to an infectious disease at a given time and place, the nurse may calculate the attack rate, a specialized form of the incidence rate. Attack rates document the number of new cases of a disease in those exposed to the disease. A common example of the application of the attack rate is food poisoning; the denominator is the number of people exposed to a suspect food, and the numerator is the number of people who were exposed and became ill. The nurse can calculate and compare the attack rates of illness among those exposed to specific foods to identify the critical food sources or exposure variables.
A prevalence rate is the number of all cases of a specific disease or condition (e.g., deafness) in a population at a given point in time relative to the population at the same point in time.

When prevalence rates describe the number of people with the disease at a specific point in time, they are sometimes called point prevalences. For this reason, cross-sectional studies frequently use them. Period prevalences represent the number of existing cases during a specified period or interval of time and include old cases and new cases that develop within the same period of time.
The following factors influence prevalence rates: the number of people who experience a particular condition (i.e., incidence) and the duration of the condition. A nurse can derive the prevalence rate by multiplying incidence by duration (P = I × D). An increase in the incidence rate or the duration of a disease increases the prevalence rate of a disease. With the advent of life-prolonging therapies (e.g., insulin for treatment of type 1 diabetes mellitus), the prevalence of a disease may increase without a change in the incidence rate. Those who survive a chronic disease without cure remain in the “prevalence pot” (Figure 5-4). For conditions such as cataracts, surgical removal permits many people to recover and thereby move out of the prevalence pot. Although the incidence has not necessarily changed, the reduced duration of the disease lowers the prevalence rate of cataracts in the population.
Morbidity rates are not available for many conditions because surveillance of many chronic diseases is not widely conducted. Furthermore, morbidity rates may be subject to underreporting when they are available. Routinely collected birth and death rates, or mortality rates, are more widely available. Table 5-2 provides examples of calculating selected rates.
Other Rates
Numerous other rates are useful in characterizing the health of a population. For example, crude rates summarize the occurrence of births (i.e., crude birth rate), mortality (i.e., crude death rates), or diseases (i.e., crude disease rates) in the general population. The numerator is the number of events, and the denominator is the average population size or the population size at midyear (i.e., usually July 1) multiplied by a constant.
The denominators of crude rates represent the total population and not the population at risk for a given event; therefore, these rates are subject to certain biases in interpretation. Crude death rates are sensitive to the number of people at the highest risk for dying. A relatively older population will probably produce a higher crude death rate than a population with a more evenly distributed age range. Conversely, a young population will have a somewhat lower crude death rate. Similar biases can occur for crude birth rates (e.g., higher birth rates in young populations). This distortion occurs because the denominator reflects the entire population and not exclusively the population at risk for giving birth. Age is one of the most common confounding factors that can mask the true distribution of variables. However, many variables, such as race and socioeconomic status, can also bias the interpretation of biostatistical data. Therefore, the nurse may use several approaches for removing the confounding effect of these variables on rates.
Age-specific rates characterize a particular age-group in the population and usually consider deaths and births. Determining the rate for specific subgroups of a population and using a denominator that reflects only that subgroup remove age bias.

To characterize a total population using age-specific rates, rates for each category must be computed separately. This is because a single summary rate, such as a mean, cannot adequately characterize a total population. Specific rates for other variables can be determined in a similar fashion (e.g., race-specific or gender-specific rates) (Table 5-3).
TABLE 5-3
Comparison of U.S. Mortality rates—2006 (Preliminary)
From Centers for Disease Control and Prevention, National Center for Health Statistics: Deaths: preliminary data for 2006, Natl Vital Stat Rep 56(16):2008: www.cdc.gov/nchs/data/nvsr/nvsr56/nvsr56_16.pdf.
Age-adjustment or standardization of rates is another method of reducing bias when there is a difference between the age distributions of two populations. The nurse uses either the direct method or the indirect standardization method. The direct method selects a standard population, which is often the population distribution of the United States. This method essentially converts age-specific rates for age categories of the two populations to those of the standard population, and it calculates a summary age-adjusted rate for each of the two populations of interest. This enables the nurse to compare the two rates as if both had the standard population’s age structure (i.e., without the prior problem of age distortion). Tables 5-4, 5-5, and 5-6 give examples of the computations.
TABLE 5-4
Direct Standardizations of Mortality Rates by Age for “South Community” and “North Community”
Age (yr) | Size of Population (A) | Percentage of Population | Number of Deaths (B) | Age-Specific Death Rate Per 1000 (B/A) |
South Community* | ||||
North Community† | ||||
< div class='tao-gold-member'> Only gold members can continue reading. Log In or Register a > to continue
![]() Stay updated, free articles. Join our Telegram channel![]() Full access? Get Clinical Tree![]() ![]() ![]() |