Outcome evaluation focuses on determining the causal effects of health interventions on the hypothesized ultimate outcomes. The goal is to demonstrate the benefits of interventions in addressing the health problem and in improving general health and well‐being in the target client population. As explained in Chapter 10, causal effects imply that the interventions’ active ingredients are solely and uniquely responsible for producing the improvement in the ultimate outcomes observed after implementation of the interventions. Thus, changes in the outcomes are attributable, with confidence, to the intervention and not to any other factor that may be operating in the context of intervention delivery such as the characteristics of clients and of their life environment. Logic, intervention theory, experience, and evidence indicate that a range of factors may influence the effects of health interventions on the ultimate outcomes (Cao et al., 2014); therefore, the factors present plausible alternative explanations of the interventions’ effects. There are two general approaches for handling these factors. The first involves an experimental control. The control consists of eliminating or minimizing the potential influence of the factors; it is accomplished by incorporating some features into the design of the evaluation study, as is advocated for examining the efficacy of an intervention. The experimental or the randomized controlled or clinical trial (RCT) design has been considered the most reliable design for determining the efficacy of health interventions (Anglemyer et al., 2014). The second approach entails an account of the factors and investigation of their influence on the intervention’s effects. This is usually done by testing the intervention’s effects under real‐world conditions that represent variability in the factors and in clients’ perceptions and responses to the intervention. Relevant data are collected to determine what factors, in addition to or in combination with the intervention, contribute to the ultimate outcomes, as is advocated for examining the effectiveness of interventions. Although the RCT is still considered appropriate, other experimental and non‐experimental designs are suggested (Medical Research Council, 2019), and have been found suitable for examining effectiveness. In this chapter, the features of the conventional RCT design are described; the pathways through which its features control for factors and improve the validity of inferences regarding the causal effects of an intervention are explained. Yet, these same features and the assumptions underlying them are being questioned in light of cumulating evidence indicating the vulnerability of the RCT design to biases that weaken the validity of inferences regarding the causal effects of interventions. The limitations of the RCT design are delineated, supported by relevant empirical evidence. Alternative experimental and nonexperimental designs are presented for examining the effectiveness of health interventions under real‐world conditions. The features, advantages or strengths, and disadvantages or limitations of these designs are discussed relative to how they overcome the limitations of the RCT. The traditional experimental or RCT design is considered the most reliable, even the “gold standard” for determining the causal effects (efficacy and effectiveness) of a health intervention on the ultimate outcomes (Winter & Colditz, 2014). The ascribed “high status” emanates from the features of the RCT that are believed to eliminate or minimize the influence of factors (other than the intervention) on the outcomes. The factors reflect the characteristics of clients, interventionists, and contexts; the delivery of the intervention; and the assessment of outcomes. The experimental control of these factors is posited to reduce their likelihood as plausible alternative explanations of the changes in the ultimate outcomes observed post treatment. Ruling out alternative plausible explanations is the most important criterion for inferring causality (see Chapter 10). Therefore, the control increases the confidence in attributing the changes in outcomes to the intervention. The main features of the RCT are careful selection of clients, random assignment to treatment conditions, blinding and concealment of treatment allocation, manipulation of treatment delivery, and outcome assessment and analysis. Clients are carefully selected to ensure enrollment of participants that are representative of the target population; yet, the participants should not have characteristics that are known or hypothesized (in the intervention theory) to affect engagement and enactment of treatment, and to confound the intervention effects (i.e. have unique and direct associations with the outcomes). Careful selection is achieved by prespecifying a set of inclusion and exclusion criteria, assessing the criteria with appropriate measures, and ascertaining that participants meet all eligibility criteria prior to exposure to treatment. The inclusion criteria ensure that participants in the RCT belong to the target client population and experience the health problem in a way that is amenable to treatment by the intervention under evaluation. Therefore, the inclusion criteria are delineated to represent the characteristics of the target client population in terms of: The exclusion criteria are used to control for clients’ characteristics that are hypothesized in the intervention theory (see Chapter 5) to interfere with participants’ engagement and enactment of the intervention, and/or to be directly associated with the ultimate outcomes. The direct associations between the characteristics and the outcomes can be present irrespective of exposure to treatment and are illustrated by the well‐established gender differences in the experience of depressive symptoms and the relationship between anxiety and knowledge gain. The client characteristics include personal, clinical, or health conditions (1) that may limit participants’ engagement and enactment of treatment, such as limited physical functioning that prevents attendance at treatment sessions or optimal adherence to treatment recommendations; (2) for which the intervention is contraindicated, such as pregnancy during which taking a medication may not be safe; and (3) for which the intervention was found ineffective or potentially harmful; for instance, cognitive therapy alone may not be appropriate for managing insomnia associated with dysfunction in circadian rhythm. Two categories of additional exclusion criteria are preset. The first is related to concurrent treatment; the treatment is prescribed for the same health problem targeted by the intervention or for comorbid conditions. Participants are excluded if they are receiving a treatment or if their healthcare providers are unable or unwilling to stop or keep constant the type and dose of concurrent treatment during the trial period. The concurrent treatment can confound or moderate (i.e. strengthen or weaken) the effects of the experimental intervention on the ultimate outcomes, in particular outcomes reflecting resolution of the health problem. The second category is related to clients’ tendency for noncompliance. It is assessed during a run‐in‐period, prior to exposure to treatment. Participants who do not comply with a task (e.g. completing a diary) are excluded in order to minimize variability in their adherence to treatment and consequently, in their level of improvement in the ultimate outcomes. The selection of participants on the basis of strict eligibility criteria is believed to “guarantee” the exclusion of participants with potentially confounding characteristics. It results in the inclusion of participants who are homogeneous in terms of their experience of the health problem and their personal and health or clinical profiles. The homogeneity of the sample is expected to contribute to the comparability of participants in the experimental intervention group and the comparison treatment group on all characteristics and outcomes measured at baseline or pretest. Participants assigned to each treatment group are also expected to exhibit comparable responses to the allocated treatment. Participants assigned to the experimental group and having similar baseline profile are all expected to show a similar pattern of improvement in the ultimate outcomes; that is, they report change in the outcome of the same direction and amount or level, following delivery of the intervention. Participants assigned to the comparison group and having a similar baseline profile are all expected to exhibit no change in the outcomes. The comparability in the baseline profile is a means for controlling the potential influence of clients’ characteristics on their responses to treatment; the influence is minimized because these characteristics are constant. Furthermore, the comparability of participants’ pattern of change in the ultimate outcomes, within each of the experimental group and the comparison group, reduces the individual variability in the level of outcomes achieved post treatment. When the posttest outcomes are compared between the two groups, the difference in the groups’ means is larger (which is the numerator in the formula for the independent sample t‐test or F‐test) than the within‐group variability (that reflects differences across individuals within groups and is the denominator in the formula for the t‐test and F‐test). Thus, the large between‐to‐within group ratio (i.e. t‐test or F‐test) increases the power to detect significant intervention effects. The effects can be confidently attributed to the intervention because the groups are similar in their baseline profile, which controls for the potential confounding influence of client characteristics on the ultimate outcomes. Random assignment or randomization is the hallmark of the experimental or RCT design. It is the feature believed to “ensure or guarantee” the comparability of the baseline profile of participants allocated to the experimental intervention and the comparison treatment groups. Therefore, randomization controls for selection bias that induces confounding and weakens internal validity, that is, the claim that the observed improvement in the ultimate outcomes is solely attributed to the intervention (Borglin & Richards, 2010; Sidani, 2015). Randomization involves the application of chance‐based procedures (see Chapter 15 for details) for allocating participants to the experimental intervention and the comparison treatment groups. The chance‐based procedures eliminate human influence, whether unconscious or deliberate, on assignment to group. Humans (e.g. researchers or health professionals) may interfere with the assignment. For instance, researchers favoring the experimental intervention have a tendency to assign to the experimental intervention, participants who are fit and have great potentials to benefit from the intervention. This pattern of assignment compromises the comparability of the study groups on baseline profiles. Randomization is believed to enhance the comparability of participants in the experimental and the comparison groups on all measured and unmeasured characteristics, before treatment delivery (Donovan et al., 2018). It leads to a situation in which participants with given characteristics assigned to one group will, on the average, be counterbalanced by participants with similar or the same characteristics assigned to the other group (Cook & Campbell, 1979). This translates into an even or balanced distribution of participants in the experimental intervention and the comparison treatment groups, with similar characteristics that may influence engagement and enactment of treatment or that may be associated with the ultimate outcomes. This comparability at baseline is believed to yield two situations. First, it holds client characteristics constant between groups. Therefore, the characteristics cannot contribute to participants’ responses to treatment, which increases the confidence in attributing the observed effects solely to the intervention. Second, the comparability reduces the individual variability in the outcomes assessed post treatment within each group. The low variability in posttest outcomes increases the power to detect significant intervention effects. Blinding (also called masking) entails concealing or not disclosing the nature (whether experimental or comparison) of the treatments included in the RCT to those involved in the trial. These include research staff (i.e. data collectors and interventionists if possible), participants, and health professionals assisting in referring their clients. Blinding requires that the treatments are comparable; they should have the same structure, mode, and dose of delivery, and should be not labeled as experimental or control (Sil et al., 2019; Wartolowska et al., 2018). Rather, the treatments are referred to by their respective label such as sleep education and stimulus control therapy for insomnia; they are described as different strategies that put emphasis on slightly different ways of addressing the health problem (e.g. McCurry et al., 2014) in all study documents and in particular, the consent information form. Blinding minimizes biases associated with perceptions and reactions to treatments that have the potential to affect the validity of inferences regarding the causal effects of the intervention. Blinding is believed to reduce the following biases: performance bias which is associated with the attention provided to participants in the intervention group, ascertainment or detection bias which is related to knowledge of the treatment that influences judgment in outcome assessment, co‐treatment bias where participants in the comparison treatment group seek additional treatment outside the trial, crossover from one treatment group to another, and attrition from treatment or study (Probst et al., 2019; Renjith, 2017; Wartolowska et al., 2018). Several pathways explain the impact of nonblinding. Participants may perceive the experimental intervention favorably that is, desirable and helpful. If not assigned to this intervention, they may react negatively resulting in attrition, nonengagement and nonenactment of the allocated treatment, resulting in less‐than‐optimal improvement in the ultimate outcomes. Health professionals’ awareness of the treatments under evaluation could affect their decision to refer their clients to the RCT, potentially influencing the size of the sampling pool. If clients enroll in the trial, health professionals’ knowledge of the treatment to which their clients (participating in the RCT) are allocated may hold favorable or unfavorable views of that treatment; they may discuss the trial treatment with participants and nudge them to continue or withdraw from treatment, respectively. In addition, health professionals may alter their assessment of participants’ condition (e.g. heightened sensitivity toward side effects) and their interactions with participants (e.g. prompting participants for experiences of side effects). This in turn, influences participants’ behaviors in the RCT (e.g. withdrawal, adherence to treatment) and responses to the allocated treatment. Research staff who are aware of which treatment is the experimental may be biased in outcome data collection and analysis. They may be tempted to observe and report improvement in the outcomes in the experimental group more so than the comparison group, and to modify the analysis plan to show that the intervention is effective. All these perceptions and reactions could confound the intervention effects and result in their biased (under or over) estimates (Sidani, 2015). Blinding is believed to mitigate these biases. Concealment of treatment allocation means nondisclosing or hiding the randomization scheme from research staff involved in participant assignment and health professionals referring clients to the RCT. Concealment is done to eliminate research staff’s and professionals’ potential influence on the assignment of participants to the experimental intervention and the comparison treatment groups. Research staff and health professionals may engage in what is depicted as “gaming the system” in order to allocate participants to a particular treatment they view as most appropriate in addressing the individual participant’s needs. For instance, research staff may delay assignment of a participant in need of an active treatment that she or he cannot afford elsewhere (Streiner & Sidani, 2015). This pattern of assignment could compromise the comparability on baseline characteristics, of participants allocated to the experimental intervention and the comparison treatment groups. Between‐group differences in characteristics introduce confounding and biased estimates of the intervention effects. Concealment of treatment allocation is best maintained by entrusting the randomization scheme to a central office. Manipulation of treatment delivery involves controlling the context and the actual provision of the experimental intervention and the comparison treatment. Controlling the context of delivery is achieved through: The selection of the setting in which the experimental intervention and the comparison treatment are delivered. The setting is selected on the basis of physical, psychosocial, and/or political features, hypothesized in the intervention theory to facilitate the delivery of the intervention. Factors that may interfere with the provision of the intervention are eliminated or maintained constant across participants over the treatment delivery period. For instance, the room temperature is kept at the same level when having participants listen to relaxing music in order to minimize potential physical discomfort that affects the achievement of the intended outcome of decreased anxiety. It is believed that providing the experimental intervention and the comparison treatment in the same setting eliminates or minimizes the influence of setting on the delivery and the effects of the intervention. The selection and training of interventionists for delivering the treatments. Interventionists are selected on the basis of clearly specified personal qualities and professional qualifications and intensively trained in the theoretical underpinning and the practical skills required for delivering the treatments. Interventionists are also instructed to give the treatments in a standard and consistent way across participants in order to enhance fidelity of treatment delivery. They are encouraged to maintain the same style of interpersonal interactions and the same demeanor with all participants in order to standardize the nature and level of therapeutic relationship or working alliance. The standardization of interactions is expected to minimize their influence on outcomes. The selection and training of research staff in the study protocol and the interaction with participants. The purpose of training is to maintain high‐quality performance of research activities, in particular data collection. Staff are encouraged to be consistent in the manner in which they behave and communicate with participants. Staff are not informed of the RCT hypotheses, the nature of the experimental and comparison treatments, and participants’ assignment to treatment, in order to ensure blinding. This is considered essential so that research staff do not develop expectancies or prejudice about treatments, that may affect their perception, observation, or judgment when collecting outcome data, particularly following treatment delivery. Controlling the actual delivery of the experimental intervention and the comparison treatment is exerted in three ways. The provision of the experimental intervention to one group and withholding it from another group of participants. This is essential to generate differences in exposure to the experimental intervention and to demonstrate differences in outcome achievement post treatment between the groups. These differences present the evidence required for demonstrating the covariation criterion of causality (Chapter 10). The specification and the provision of the comparison treatment. Ideally, participants assigned to the comparison treatment group should not be exposed to any treatment—called no‐treatment control condition. The no‐treatment condition is required to demonstrate the covariation criterion of causality. Further, participants who may be taking a prescribed treatment to manage the health problem targeted by the experimental intervention are requested to stop taking it or to keep its dose constant throughout the RCT treatment period. Withholding or keeping constant a prescribed treatment minimizes the potential threat of co‐treatment because the prescribed treatment may confound or moderate the effects of the experimental intervention on the outcomes. However, the no‐treatment control condition presents an ethical dilemma when a much needed treatment is withheld. Other comparison treatments can be and have been used in RCTs (see Chapter 15) such as the placebo treatment and usual care. It is essential that the selected comparison treatment should not incorporate any of the active ingredients that characterize the experimental intervention, in order to minimize potential overlap between the experimental intervention and the comparison treatment. The overlap affects the magnitude of the between‐group differences in the posttest outcomes and, hence, the power to detect significant intervention effects. That is, if participants in the comparison group are exposed to some active ingredients of the intervention, then they may experience, to some degree, improvement in the outcomes assessed at posttest. This improvement reduces the magnitude of the between‐group differences and increases the variability within the comparison group resulting in nonsignificant intervention effects. The standardized and consistent delivery of the intervention. The same treatment components, content, and activities comprising the intervention are given, with fidelity, in the same way and at the same dose to all participants assigned to this experimental group. Standardized and consistent delivery is expected to reduce variability in levels of exposure to the intervention, and in enactment of the treatment recommendations and subsequently, in responses to the intervention. Thus, participants are expected to demonstrate similar or same levels of improvement in the outcomes measured at posttest. With the decreased variability in posttest outcomes within the experimental group, the power to detect significant intervention effects is increased. Standardized and consistent delivery is also applicable to the comparison treatment if different from no‐treatment conditions. Standardized and consistent delivery minimizes variability in levels of exposure to the comparison treatment and the potential for contamination, both of which could yield nonsignificant intervention effects. In an RCT, the timing for outcome assessment is well specified relative to the delivery of the experimental intervention. The timing is the same in both the experimental intervention and the comparison treatment groups, which is critical for meeting the temporal order criterion of causality (Chapter 10). Therefore, all outcomes are measured in both groups, at the same occasions: at least once before and once after treatment is given. The outcomes assessed before treatment (baseline or pretest) serve as a reference point for comparison with the outcomes measured after treatment (posttest). The comparison over time delineates the pattern of change, that is, direction (increase, no change, decrease) and magnitude (how much) of change. The pattern of change is important to determine the extent to which participants in the experimental intervention group experience the hypothesized improvement in the outcomes, and to compare this group’s changes in outcomes to the comparison treatment group’s anticipated report of no changes in the outcomes. Differences in the pattern of change in the outcomes between groups represent the evidence supporting the efficacy or effectiveness of the intervention. In an RCT, analysis of the outcome data is based on the intention or intent‐to‐treat principle. In intent‐to‐treat analysis, all participants randomized to the experimental intervention and the comparison treatment groups are included, whether or not they completed the allocated treatment or the study. As such, the comparability of the two groups (achieved through randomization) on baseline profile is maintained. The baseline comparability controls for selection bias and the influence of any potential confounding variable. To be able to conduct the analysis, outcome data are imputed for participants who withdraw, using the mean of the participants’ respective group at posttest or the last observation carried forward. It is believed that the intent‐to‐treat analysis is the most appropriate, providing valid estimates of the causal effects of the intervention on the outcomes, because it controls for selection and confounding bias. Traditionally, the features of the experimental or RCT design were considered the strengths of this research design, making it the most appropriate or the gold standard for outcome (efficacy and effectiveness) evaluation. The features are congruent with the conventional notion of causality that focuses on the direct association between the intervention and the outcomes. The RCT features operationalize the criteria for determining conventional causality and are expected to yield unbiased estimates of the intervention effects. However, the RCT features may not align well with the recently acknowledged notion of multicausality (Chapter 10). They are limiting in examining the effectiveness of health interventions delivered in real‐world practice and in providing answers to practice‐relevant questions: Who most benefit, from what intervention, given in what mode and at what dose? How does the intervention work to produce beneficial outcomes? Furthermore, cumulating experience (in designing and conducting RCTs) and empirical evidence (mainly generated through systematic reviews of RCT findings) have identified limitations of the experimental or RCT design in evaluating outcomes of health interventions. Despite its advantages in controlling for potential confounding factors and hence, in establishing a causal relationship between an intervention and the ultimate outcomes (Deaton & Cartwright, 2018; Frieden, 2017; Holm et al., 2017), the experimental or RCT design has several limitations. The limitations stem from its features that ignore the complexity of the real world, which is characterized by multicausality, heterogeneity, and flexibility. As discussed in Chapter 10, multicausality recognizes that the intervention is not the only determinant of health outcomes (Chavez‐MacGregor & Giordano, 2016); rather, a range of factors, inherent in a particular context in which the intervention is delivered and related to the characteristics of participants, interventionists, setting or environment, the nature and method of delivering the intervention, influence directly the ultimate outcomes or interact with the intervention in producing the outcomes (Amaechi & Counsell, 2013; Daniel et al., 2016; Diez‐Roux, 2011; Fernandez et al., 2015; Hansen & Tjørnhøj‐Thomsen, 2016; Mazzuca et al., 2018; Robins & Weissman, 2016; Tarquinio et al., 2015; VanderWeele, 2016; WanderWeele et al., 2016). Furthermore, multicausality recognizes the complexity of the mechanism of action for health interventions, whereby several specific and nonspecific processes mediate the effects of the intervention on the ultimate outcomes (Bonell et al., 2012; Fletcher et al., 2016; Midgley et al., 2014). Yet, understanding these processes is the essence of causal explanation or inference (Blackwood et al., 2010; Johnson & Schoonenboom, 2016; Villeval et al., 2016). By focusing on the direct association between the intervention and the ultimate outcomes, and by experimentally controlling for contextual factors, the traditional RCT may not be well suited to capture the complex processes contributing to the intervention’s effects in the real‐world context. Heterogeneity and flexibility are other characteristics of the real world that are ignored in the RCT. The broad assumption underlying the RCT is that participants have comparable sociodemographic and health or clinical characteristics, and experience the health problem in a similar way (e.g. same level of severity). Therefore, they can all benefit from the same intervention, given in a fixed mode and dose (Mohr et al., 2015). Participants are also expected to react in a similar manner to the health intervention and to respond to it in the same or similar way. Accordingly, the focus in the RCT is on the “average” effects (Krauss, 2018). Individual variability in responses to treatment is ignored (Nahum‐Shani et al., 2012) and is usually represented as “error” variance in outcome data analysis (i.e. denominator in the t‐test or the F‐test). The RCT findings are of limited relevance to real‐world practice, where the emphasis is on client centeredness. Client centeredness involves accounting for clients’ individuality, tailoring treatment to meet their individual experiences of the health problem, concerns and life circumstances, and adapting treatment on the basis of clients’ observed responses to treatment (Fernandez et al., 2015; Ling, 2012; Marchal et al., 2013; Mohr et al., 2015; Reynolds et al., 2014). Ironically, the features of the traditional RCT design reflect both its strengths and limitations. The limitations are serious enough to weaken internal validity and external validity including the meaningfulness of the RCT findings in informing practice. Conceptual arguments and relevant empirical evidence (where available) pointing to the limitations of the traditional RCT are presented next. A set of strict, stringent, or restrictive eligibility criteria is specified in the traditional RCT to control for client characteristics that could potentially influence engagement and enactment of treatment, and/or confound the intervention effects on the ultimate outcomes. The application of such criteria contributes to: Results of individual RCTs (e.g. Cha et al., 2016) and systematic reviews indicate that less than 50% of clients recruited for an RCT meet the preset eligibility criteria (e.g. Grapow et al., 2006) and the accrued sample size is, on average, small (Golfam et al., 2015). Small sample sizes reduce the power to detect significant intervention effects and yield unreliable or unstable estimates of the intervention effects (Greenhalgh et al., 2014; Golfam et al., 2015). Furthermore, the sample (small or large) accrued in an RCT is not representative of all subgroups comprising the target client population, leading to sample selection bias (Berger, 2018; Yang et al., 2017). Differences in the personal and health or clinical characteristics of participants and nonparticipants in an RCT have been reported. In general, compared with nonparticipants, participants are depicted as younger; less deprived that is, have higher education and income, and are of the dominant ethnicity (Cha et al., 2016); having fewer comorbid conditions (Chavez‐MacGregor & Giordano, 2016) or being high risk (Frieden, 2017); being more motivated to get treatment for the health problem; and open to new treatment (Tarquinio et al., 2015; Troxel et al., 2016). The unrepresentativeness of the RCT samples of the target client population is additionally supported by estimates consistently indicating that less than 40% of clients seen in real‐world practice meet the eligibility criteria (Hershkop et al., 2017; Shean, 2014). Accordingly, the RCT findings, based on a selective subgroup of the target client population, are of limited applicability in real‐world practice, as the findings are relevant or applicable to a rather small percentage of the client population (Frieden, 2017; Horwitz et al., 2017; Leviton, 2017; Tomlison et al., 2015; Troxel et al., 2016; Woodman, 2014). Non‐applicability of findings may account for the slow uptake of RCT‐derived evidence in practice (Donovan et al., 2018). The limited generalizability and applicability of RCT findings is further compounded by nonconsent bias, as discussed in the next section. Although random assignment has been considered the main strength of the RCT in mitigating selection bias and controlling for potential confounding, it has been recently questioned on scientific and practical grounds. There is also evidence linking randomization to some biases, including nonconsent or nonenrollment bias and unfavorable reactions to the allocated treatment (see Chapter 11 for detail) that threaten the validity of inferences. Scientifically, randomization does not secure valid inferences about the causal effects of the intervention on the ultimate outcomes (Hernán, 2018). Randomization increases the likelihood of the comparability between participants assigned to the experimental intervention group and the comparison treatment group, thereby minimizing selection bias. This is all it does, nothing else! Randomization does not prevent, address, or rule out other, equally important, biases that are introduced following randomization and that weaken the confidence in attributing the improvement in the ultimate outcomes, solely, to the intervention (Cook et al., 2010; Hernán, 2018; Krauss, 2018; West & Thoemmes, 2010). These biases include: differential attrition, contamination or crossover, non‐adherence to treatment recommendations, co‐treatment, interventionists’ interactional style, and participants’ perceptions and engagement in treatment. Contrary to common belief, randomization does not guarantee or ensure baseline comparability on all measured and unmeasured or known and unknown characteristics of participants assigned to the experimental intervention and the comparison treatment groups, within a particular RCT, especially when the sample size is small that is, less than 1000 (Berger, 2018; Frieden, 2017; Henry et al., 2017). Accordingly, some known and many unknown factors may not be equally distributed between groups with randomization (Krauss, 2018). Some argue that any between‐group differences in participants’ baseline characteristics observed with randomization are due to “chance” (and not human influence or interference). The counterargument is that the characteristics showing these differences may affect participants’ engagement and enactment of treatment and be directly correlated with the ultimate outcomes. Thus, even if due to chance, differences in baseline characteristics between groups result in selection bias and confound the effects of the intervention on the ultimate outcomes (Rickles, 2009). In addition, the comparability on baseline profile is anticipated, maintained, and examined at the group level, and not at the individual level. Group level comparability is determined by nonsignificant between‐group differences in the personal and health or clinical characteristics measured at baseline. However, interindividual differences, within each group, in these characteristics are not controlled with randomization. The interindividual differences are represented in large within‐group variance. They can still operate by affecting participants’ engagement and enactment of treatment, and experience of improvement in the ultimate outcomes at posttest. The latter point is supported by evidence from two reviews (Heinsman & Shadish, 1996; Sidani, 2006). The reviews’ results showed a significant, low‐moderate, positive correlation between the effect sizes for outcomes measured at pretest and the effect sizes for the same outcomes measured at posttest. This correlation suggests that baseline variables continue to exert their influence on the outcomes, despite randomization. This point resurged in the literature; Liu and Maxwell (2020) explained that the amount of change in the outcomes is proportional to the baseline values. At the practical level, randomization is not acceptable to clients. It does not reflect how treatment is provided in practice: treatment is given to meet individual experiences of the health problem and after careful consideration of alternative treatments. As mentioned in Chapter 11, clients participating in an RCT may have preferences for the treatments they view as acceptable. Clients resent randomization as it deprives them their right to actively engage in treatment decision‐making and from getting the treatment they desire. This unfavorable perception of randomization contributes to participants’ decision to: Evidence from several recent meta‐analyses that compared the effect sizes for the same intervention, given to the same target client population, obtained in RCT and nonrandomized (e.g. observational) studies, consistently indicates convergence in findings across research designs. The estimated effects reported in RCTs were not significantly different from those found in nonrandomized studies (Anglemyer et al., 2014; Finch et al., 2016; Golfam et al., 2015; Mallard et al., 2016; Nelms & Castel, 2016; Rigato et al., 2017; Smeeing et al., 2017; Soni et al., 2019; Tang et al., 2016). This evidence suggests that well‐designed nonrandomized studies that minimize or appropriately account for biases produce results that approximate those of RCTs. Therefore, the important role traditionally ascribed to randomization in ensuring high internal validity and unbiased estimates of the intervention effects, is questionable (Golfam et al., 2015), which led Krauss (2018) to warn again “blind faith” in RCT as they are fallible. Blinding and concealment of allocation may not be feasible in RCTs evaluating health interventions, especially when the comparison treatment is not comparable to the experimental intervention. The noncomparability is obvious when the comparison treatment is a no‐treatment control (Younge et al., 2015). The latter treatment condition may not be structurally equivalent to the experimental intervention (see Chapter 15). Even when blinding is possible, it is difficult to maintain once the allocated treatment (whether pharmacological or nonpharmacological) is delivered (Tarquinio et al., 2015). Participants are active agents, capable of interpreting their experiences, making decisions and taking actions. They are able to monitor their experience of the health problem and to correctly guess the treatment they receive, either independently or in collaboration with their healthcare providers (Berger, 2018; Kowalski & Mrdjenovich, 2013). Participants who receive the experimental intervention experience improvement in the health problem and other health outcomes. They may also experience possible side effects associated with the intervention. Participants exposed to the comparison treatment do not experience changes in the health problem. Three systematic reviews (Baethge et al., 2013; Broadbent et al., 2011; Hróbjartsson et al., 2007) examined trials that reported on the success of blinding by asking participants to guess the treatment received. Overall, the findings showed that blinding was unsuccessful in less than 45% of the trials included in the reviews. Baethge et al. (2013) and Broadbent et al. (2011) reported that up to two‐thirds of participants assigned to the experimental intervention or the placebo treatment correctly guessed the treatment they received. Further, the evidence on the effects of blinding and concealment is inconsistent. Whereas the findings of systematic reviews (Hróbjartsson et al., 2014; Probst et al., 2019; Saltaji et al., 2018) show that lack of client and healthcare provider blinding was associated with large estimates of the interventions’ effects, the results of a review of meta‐epidemiologic studies (i.e. review of systematic reviews and meta‐analyses) indicated that blinding (or lack of) affected, to a little extent, the estimates of the interventions’ effects (Page et al., 2016). The evidence questions the utility of blinding in evaluating a range of health interventions. The nature of the experimental intervention, the type of comparison treatment, and the control exerted in delivering both treatments in an RCT are different from what is offered and what happens in real‐world practice. The differences may be grave, rendering the trial findings of no clinical relevance (Johnson & Schoonenboom, 2016). The nature of the experimental intervention: Health interventions evaluated in a traditional RCT are usually discrete, composed of specific active ingredients. They are provided either in isolation of (i.e. participants are asked to stop other prescribed treatments) or on top of other prescribed treatments, to all participants (i.e. regardless of their individual experience of the health problem, concerns and life circumstances), in a standardized way and in fixed mode and dose. Such interventions are described as “unrealistic” (Hernán et al., 2013) and hence, challenging, if not impossible, to integrate in real‐world practice. Real‐world context is characterized by flexibility: the norm is the provision, by multiple health professionals, of different yet complementary treatments that are selected and tailored to the individual clients’ characteristics, and further adapted (in terms of type, mode, and dose) in light of individual responses to treatment. The traditional RCT is ill‐suited to evaluate the outcomes of adaptive interventions (Nahum‐Shani et al., 2020). The type of comparison treatment: In an RCT, a comparison treatment is included. The capacity of the intervention in inducing the hypothesized changes in the ultimate outcomes is inferred from comparing the posttest outcomes between the experimental intervention and the comparison treatment groups, a situation that is not afforded in real‐world practice. In practice, the effectiveness of treatment is examined through within‐person comparison over time. Thus, participants serve as their own control as the responses to treatment are individual and dependent on personal baseline profile (Frieden, 2017; Johnson & Schoonenboom, 2016; Liu & Maxwell, 2020). In addition, the comparison treatment in a traditional RCT is typically a no‐treatment or placebo treatment. These two types of comparison treatments may introduce biases associated with participants’ reactions to treatment. Those allocated to no‐treatment or placebo treatment may be disappointed and dissatisfied, and therefore, may withdraw from the trial and seek treatment outside the RCT. Alternatively, clients allocated to the no‐treatment or placebo treatment react negatively, expressed as worsening outcomes at posttest. All reactions contribute to biased (under or over) estimates of the intervention effects (Chemla & Hennessy, 2016; Horwitz et al., 2017). In addition, results indicating that health interventions are more effective than no‐treatment or placebo treatment are of limited relevance to practice, because these comparison treatments are not viable options in practice, except where no‐treatment watchful waiting or monitoring is an appropriate option (as in early stage prostate cancer). Health professionals and policy makers want information on the comparative effectiveness of different active treatments, that is, how does the new intervention fare in comparison to available treatments in current use, before integrating it in practice. The delivery of intervention: In a traditional RCT, the intervention is provided by carefully selected and intensively trained interventionists. They strictly adhere to the manual in order to deliver treatment in a standardized manner and with fidelity, in a context that has all required material and human resources. Thus, the intervention is delivered in a rigid way and the focus is on isolating its causal effects on the ultimate outcomes, disregarding the potential influence of the context of delivery (Bradley et al., 2009; Leviton, 2017; Shean, 2014; Tomlison et al., 2015). This is in contrast with the delivery of interventions in practice. In real‐world practice, interventions are delivered by health professionals with varying personal qualities and professional qualifications, who often receive brief, mainly didactic training (due to time constraints). Health professionals differ in their acceptability of the intervention, competency in delivering the intervention, ability to initiate and maintain a good rapport with clients, and interpersonal style. They also vary in their success in clearly relaying treatment‐related information to clients and in motivating clients to engage in treatment and enact the treatment recommendations. The influence of interventionists or health professionals on the outcomes is well documented, accounting for 69% of the variance in the outcomes of psychotherapy (Mulder et al., 2018). Ignoring interventionists’ influence in an RCT yields biased results and estimates of the intervention effects (see Chapter 8). In practice, interventions must be tailored to fit the individual clients’ characteristics and life circumstances. Further, interventions are provided in a flexible manner that is responsive to the clients’ changing condition. Standardized interventions are not compatible with the demands of real‐world practice. Thus, the experimental control over intervention delivery restricts the type of health interventions to evaluate in a traditional RCT; the interventions in an RCT are usually standardized (Shean, 2014; Tarquinio et al., 2015). In practice, interventions have to be adapted to fit the physical, sociocultural and political features, as well as the human and material resources available in a particular setting. Nondisclosure of these features and resources, and not accounting for their direct and indirect impact on the outcomes limit efforts to integrate the intervention in practice, appropriate adaptation of the intervention (without affecting its active ingredients), and understanding how the intervention works. Ignoring the influence of context contributes to nonreplication of the intervention effects across research or practice settings (Van Belle et al., 2016). Lastly, interventions are given in conjunction with other treatments that clients need. The latter treatments may interact with the intervention in that they may strengthen or weaken the intervention’s effects. Eliminating or ignoring treatment interactions is not useful in fully informing treatment decision‐making in practice. In a traditional RCT, the ultimate outcomes are assessed before and after intervention delivery; follow‐up assessments are scheduled at regular intervals, usually over a rather short time period (one to two years on average). The short term follow‐up precludes the assessment of the durability of the intervention effects (Frieden, 2017) and of the safety of the long term use of the intervention. The outcome analysis focuses on the direct association between the intervention and each of the hypothesized ultimate outcomes; it is concerned with group‐level comparison; and it follows the intent‐to‐treat principle. The results of such analysis may be biased and of limited relevance to practice. The direct association between the intervention and the ultimate outcomes disregards the mechanism of action. This limits the understanding of how the intervention works and what exactly contributed to the ultimate outcomes. Thus, the results fail to address questions of scientific and clinical importance: How does the intervention work and what causal mechanisms operate, where and when (Byrne, 2013). Answers to these questions are essential to inform the refinement, delivery and evaluation of the intervention in research and practice, and to guide decision‐making in practice (Van Belle et al., 2016). Furthermore, the direct effects of the intervention on the ultimate outcomes should be carefully interpreted. Consistent with the intervention theory and the hypothesized indirect relationship, the direct effects of the intervention on the ultimate outcomes are expected to be small or nonsignificant, being mediated by the immediate and intermediate outcomes (Wiedermann & von Eye, 2015). In this case, the interpretation of findings related to the direct impact of the intervention is erroneous. Conclusions that the intervention is not effective in improving the ultimate outcomes may be incorrect as the intervention has the capacity to induce the mechanism of action that mediates its impact on the ultimate outcomes. The focus on group‐level comparison ignores the heterogeneity in individual participants’ responses to the intervention. Heterogeneity, represented in individual differences, implies that some participants experience improvement in the outcomes (called responders); others show no change in the outcomes; and still others report worsening in the outcomes. Individual differences are reflected in the error term of statistical tests. When large, individual differences reduce the power to detect differences in the outcome between the experimental intervention and the comparison treatment groups, leading to type I error and the inference that the intervention is not effective. Therefore, a potentially useful intervention is abandoned. To minimize error, it is important to acknowledge that interventions do not work universally. It is equally useful to determine who benefit, to what extent, from the intervention to yield results that inform treatment decision‐making in practice (Beutler et al., 2016; Boyer et al., 2016). The intent‐to‐treat principle translates into analyzing the ultimate outcomes for participants randomized to the experimental intervention and the comparison treatment groups, regardless of their withdrawal, crossover, and adherence to the allocated treatment. The goal is to maintain the comparability of the groups in baseline characteristics and consequently, to control for potential confounding at the data analysis stage (Ranganathan et al., 2016). The logic of intent‐to‐treat principle has been questioned. Intent‐to‐treat analysis is believed to estimate the effects of “treatment assignment” (Hernán & Hernádez‐Diaz, 2012; West & Thoemmes, 2010), rather than the causal effects of the intervention: an intervention cannot produce changes in the outcomes if the participants are not exposed to it and do not engage and enact it. In addition, evidence consistently demonstrates that results based on the intent‐to‐treat analysis are biased, often underestimating the intervention effects on the outcomes. Underestimated effects potentially lead to type II error and the incorrect conclusion that the intervention is ineffective (Candlish et al., 2017; Hernán & Hernádez‐Diaz, 2012; TenHave et al., 2008). The analysis should be supplemented with per‐protocol analysis. Advances in research designs and methods have been made to address the limitations of the traditional RCT. These are summarized in Table 14.1. Advances in research designs are presented in the next section, and those pertaining to methods for conducting an intervention evaluation study are described in Chapter 15. TABLE 14.1 Overview of designs and methods addressing the limitations of the traditional RCT. A pragmatic approach to intervention evaluation underpins the advances in research designs. A pragmatic approach calls for examining the effects of health interventions under the real‐world conditions, which enables the generation of evidence that is relevant to practice (Methodological Committee of the Patient‐Centered Outcomes Research Institute [PCORI], 2012). A pragmatic approach acknowledges the complexity of the real‐world and embraces the notions of multicausality, flexibility, and heterogeneity that are inherent in the real world. Consequently, it advocates against the high experimental control that characterizes the traditional RCT design in order to reflect the natural diversity of clients, interventionists, contexts, health interventions and outcomes, and to examine the intricate relationships among multiple factors, occurring at multiple levels and contributing to the effectiveness of interventions. The goal is to provide answers to questions guiding real‐world treatment decisions: Who benefit from which health intervention, given by whom, in what mode, format and dose? How does the intervention work, in what context? What are risks or discomforts associated with the intervention? Intervention evaluation studies that are informed by a pragmatic approach have any or a combination of these features: (1) selection of participants that represent the diversity (personal and health profiles) of the target client population; (2) selection of sites or settings that represent the diversity (physical, sociopolitical, availability of resources) of practice setting; (3) comparison of the health intervention of interest to other active treatments considered as standards of care; (4) involvement of health professionals in the implementation of the intervention in a standard or tailored format; (5) assessment of a range of outcomes, including patient‐oriented or ‐centered health outcomes, over time; and (6) use of random, nonrandom, or a mix of both methods for treatment allocation. Accordingly, different designs are being developed and are considered appropriate for evaluating health interventions under real‐world conditions. It is beyond the scope of this book to review all research designs mentioned in the literature. However, the experimental or randomized, quasi‐experimental or nonrandomized, and mixed designs that are commonly and recently used in intervention research are discussed next. Where available, different terms used to refer to the same design are presented. The experimental or randomized category represents designs that extend the traditional RCT. The extensions consist of some modification in the trial’s features aimed to address specific limitations or challenges encountered in the conduct of the traditional RCT (Krauss, 2018). The waiting‐list control (WLC) group design is also called delayed start design (D’Agostino, 2009) or deferred‐treatment design (Campbell et al., 2005). It has been recommended when withholding treatment and randomization of individual participants to treatment groups are unethical or unacceptable. This may be the case when: (1) there is empirical evidence (synthesized from previous research) indicating that the health intervention under evaluation is more beneficial (i.e. demonstrates larger effects) than standard care; (2) the target client population is in pressing need for treatment that is not affordable outside the trial; or (3) clients, health professionals, and decision‐makers involved in the trial find it ethically unacceptable to withhold treatment and to provide treatment on the basis of chance (Sidani, 2015). The WLC group design is a viable alternative because it mimics the pattern of treatment delivery followed in practice, where some clients are given the needed treatment at different points in time (i.e. immediately or delayed) as a result of limited resources in a practice setting. The WLC group design has features comparable to those characterizing the traditional RCT, except that participants in the comparison or control group are provided the intervention at a delayed time. Thus, at the end of the trial, all participants are exposed to the experimental intervention. The conduct of the WLC group design involves these steps: The outcome data analysis involves: The WLC group design has some advantages. It addresses the ethical issues related to: (1) withholding any type of treatment in a no‐treatment control group (done in some traditional RCT) and (2) denying a potentially beneficial intervention for participants in need of treatment (Cunningham et al., 2013). In the WLC group design, all participants are aware that they will ultimately receive the experimental health intervention, whether immediately or at a later time, as is typically done in practice. This awareness entices them to participate in the trial, thereby increasing trial enrollment rates (Sidani, 2015) and accrual of the required sample size within the study time line. Similarly, health professionals are aware that all participants will receive the experimental intervention. Therefore, they are not compelled to disseminate the experimental intervention (if they are responsible for delivering it) to the WLC group, thereby minimizing the risk of contamination. Also, they are not compelled to enhance usual treatment provided to participants in the control group, thereby reducing the risk of treatment compensation (Campbell et al., 2005). The WLC group design enables between‐ and within‐group outcome analyses. The between‐group comparison (Time 2) reflects the covariation criterion for causality; thus, it is useful in determining the causal effects of the experimental intervention on the outcomes. In the within‐group outcome analysis, in particular within the delayed group, individual participants serve as their own control because they are exposed first to no treatment and second to the experimental intervention, a situation reflective of the counterfactual. The comparison on outcomes within the delayed treatment group is important for determining the causal effects of the intervention, unbiased by the potential confounding influence of participants’ baseline characteristics (Berry et al., 2006) (further discussed in Section 14.3.2.4). In addition, the analysis examining changes in the outcomes from pretest to posttest, done in the pooled sample, yields reliable estimates of the magnitude of improvement in the outcomes, immediately following completion of the experimental intervention. The limitations of the WLC group design stem from the nature of the health problem and its recovery, participants’ expectations, and logistical issues. Participants experiencing acute, time‐limited health problems (e.g. common cold, acute fatigue or insomnia, skin abrasion) and assigned to the delayed group may spontaneously and naturally recover, resulting in improvement in the outcomes at Time 2. This improvement reduces the size of the between‐group differences at Time 2, leading to an underestimation of the intervention effects. Participants randomized to the delayed group may have different expectations. Some may have desired immediate attention and treatment to manage what they consider as a pressing, severe health problem; those disappointed may withdraw early in the trial (i.e. before Time 2) to seek treatment outside the trial. High attrition rates, in particular in the delayed group, have been reported (Foster, 2012). High attrition rates contribute to reduced sample size and statistical power, and to post‐randomization confounding, and subsequently to biased estimates of the intervention effects. Other participants in the delayed group anticipate that their health problem or its severity will improve over time. This expectancy is reflected in reported (even if small) improvement in the outcomes at Time 2, leading to underestimated intervention effects (Sidani, 2015). Still, other participants in the delayed group expressing readiness to change or taking steps to make the change, may halt their change initiative because they perceive that they have to wait to make the change until they receive the experimental intervention, as reported by Cunningham et al. (2013). The logistical problems associated with repeated measurement of outcomes may be viewed as burdensome by participants, contributing to their withdrawal (Berry et al., 2006). The crossover design is similar to the WLC group design in that participants are crossed over from one treatment to another during the study period. However, the treatments consist of either different components of a complex, multicomponent intervention or different interventions addressing the same health problem. The design can be used in two situations: (1) to compare the effects of the selected components or interventions in a situation where it is ethically unacceptable to withhold treatment, and (2) to determine the most appropriate, effective, safe and efficient sequence for providing the components or the interventions. In the latter situation, the findings inform the implementation of a stepped approach to care, which is desirable to reduce the burden of care for participants (e.g. providing intensive treatments to those who may not need it) and for health professionals in practices with limited resources. The features of the crossover design are comparable to those of the WLC group design, except for incorporating washout periods. The washout period is of higher importance for trials aimed to compare the components or the interventions than trials focusing on determining the appropriate sequence of treatments’ delivery. The washout period is scheduled after providing a component or intervention but before exposure to another. During this washout period, the first component or intervention is withheld to allow its effects to dissipate prior to providing the second component or intervention. This is important to prevent the carryover effects of the first component or intervention and to minimize the cumulative influence or the interaction (strengthening or weakening) effects of the first with the second component or intervention. The length of the washout period is informed by available theoretical or clinical knowledge of the duration of each component’s or intervention’s effects; otherwise, it is logical to specify the duration of the washout period to be equal to the duration for giving the components or interventions (Sidani, 2015). For instance, if it takes four weeks to provide an intervention, then the washout period is four weeks. The sequence for providing the components or interventions is planned in advance. This is usually done by randomizing the order for exposure to the components or interventions: participants are randomly assigned to different sequences. For example, some participants receive intervention 1 followed by intervention 2, whereas others are exposed to intervention 2 followed by intervention 1, separated by a washout period. When the concern is the development of a stepped approach to intervention delivery, the delineation of the sequences for providing components or interventions can be informed by the intervention theory or relevant clinical knowledge. For instance, foundational, non‐intensive components (e.g. sleep education and hygiene) could be offered first, followed by more intensive ones (e.g. stimulus control therapy). Alternatively, the order for giving the intensive components could be randomized (e.g. stimulus control therapy then relaxation therapy then sleep restriction therapy, versus relaxation therapy then stimulus control therapy then sleep restriction therapy). Conducting a crossover design involves: The outcome analysis is complex as it should account for the possible carryover and ordering effects when comparing the effects of the components or interventions, as explained by Wellek and Blettner (2012). The analysis includes comparisons between groups after receiving each component or intervention, shedding light on their relative (to each other) effectiveness. The analysis also includes comparisons within group over time. These comparisons generate evidence on the capacity of each component or intervention to induce the beneficial outcomes while controlling for possible confounding associated with participants’ characteristics (since participants serve as their own control). The crossover design enables the concurrent evaluation of two or more components or interventions, or the sequence for providing them, thereby generating evidence of relevance to practice. All participants receive treatment, thereby mitigating the ethical dilemma of withholding treatment to those who need it. Since participants receive all components or interventions under evaluation, they serve as their own control, allowing comparison of the same participants under different components or interventions. This comparison controls for or minimizes the potential influence of client characteristics on treatment engagement, enactment, and outcomes (Berry et al., 2006). It yields two advantages: (1) reduced error variance, which increases the statistical power to detect significant intervention effects; and (2) need for a small sample size, estimated to be about one half of that required for traditional RCTs (Hui et al., 2015).
CHAPTER 14
Outcome Evaluation: Designs
14.1 TRADITIONAL RCT DESIGN
14.1.1 Careful Selection of Clients
14.1.2 Random Assignment
14.1.3 Blinding and Concealment of Treatment Allocation
14.1.4 Manipulation of Treatment Delivery
14.1.5 Outcome Assessment and Analysis
14.2 LIMITATIONS OF THE TRADITIONAL RCT DESIGN
14.2.1 Careful Selection of Clients
14.2.2 Random Assignment
14.2.3 Blinding and Concealment of Allocation
14.2.4 Manipulation of Treatment Delivery
14.2.5 Outcome Assessment and Analysis
RCT limitation
Design
Method
Reduced pool of potentially eligible participants
Multisite RCT and/or cluster RCT
Multiprong recruitment strategy
Small percentage of clients meeting eligibility criteria resulting in small, unrepresentative sample
Pragmatic approach informing experimental (e.g. practical clinical trial) or quasi‐experimental (e.g. cohort) trials
Specification of broad, less restrictive eligibility criteria.
Power analysis to estimate required sample size
Participants’ declining randomization and preferences for treatment
Preference trials
Assessment of preferences (Chapter 11)
Assignment to treatment of choice
High attrition
Integration of strategies to minimize attrition
Crossover and contamination within site
Cluster RCT
Conduct of process evaluation (Chapter 13) and of per protocol analysis
Delivery of fixed interventions in a standardized manner
Adaptive interventions and designs
Development of protocol or manual to adapt intervention (Chapters 6, 7, and 9)
Conduct of process evaluation (Chapter 13)
Limited relevance of comparison (no‐treatment, placebo) treatment
Comparative effectiveness trials
Within‐subject designs
Conduct of process evaluation (Chapter 13)
Ignoring influence of interventionists
Pragmatic approach guiding experimental and quasi‐experimental studies
Assessment of interventionists’ characteristics (Chapter 8)
Accounting of nesting of participants within interventionists
Examining influence of interventionists in outcome analysis
Ignoring influence of context and/or concurrent treatment
Cluster RCT
Pragmatic approach guiding experimental and quasi‐experimental studies
Mixed (quantitative and qualitative) method designs
Assessment of contextual factors and concurrent treatment
Accounting for nesting of participants within sites
Examining influence of sites and of contextual factors in outcome analysis
Conduct of process evaluation
Subgroup analysis to examine impact of concurrent treatment
Ignoring participants’ needs and response to treatment
Adaptive interventions and designs
Regression‐discontinuity design
Assessment of characteristics at baseline
Delivery of treatment as specified in tailoring protocol
Assessment of outcome (response to treatment) at regular intervals
Subgroup or multilevel outcome analysis
Ignoring intervention’s mechanism of action
Mixed (quantitative and qualitative) method designs
Assessment of mediators (representing the mechanism of action)
Integration of process and outcome evaluation
Conduct of meditational analysis
Ignoring level of exposure to treatment
All types of designs
Assessment of exposure, engagement and enactment (Chapter 9)
Conduct of per‐protocol analysis and account for level of exposure in outcome analysis
Limited capacity to examine long‐term effects of intervention
Quasi‐experimental design and within‐subject design with long‐term follow‐up
14.3 ALTERNATIVE DESIGNS
14.3.1 Experimental or Randomized Designs
14.3.1.1 Waiting‐List Control Group Design
Features
Advantages/Strengths
Disadvantages/Limitations
14.3.1.2 Crossover Design
Features
Advantages/Strengths
Disadvantages/Limitations
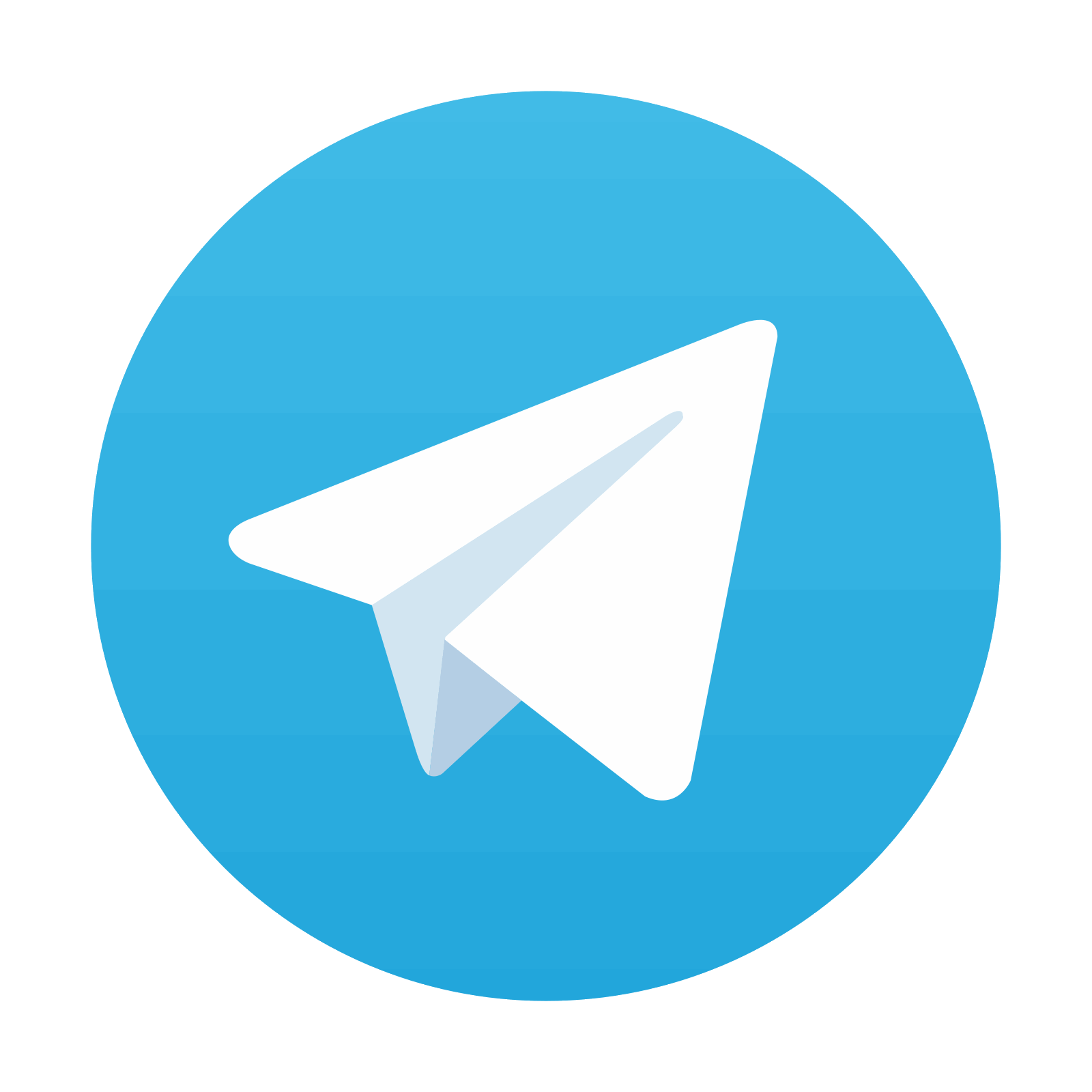
Stay updated, free articles. Join our Telegram channel

Full access? Get Clinical Tree
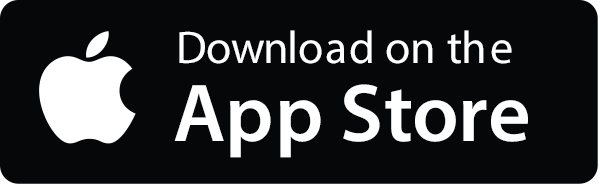
