Evaluation of newly designed health interventions is a necessary step preceding their implementation in practice. Evaluation consists of a systematic process for determining the merit, worth, or value of health interventions. The value of interventions is indicated by their appropriateness, effectiveness, safety, and efficiency in addressing clients’ experience of the health problem and in promoting clients’ health (Chapter 1). Traditionally, evaluation research has been concerned with demonstrating the effectiveness of interventions on the ultimate outcomes. To this end, several studies are conducted, with the expectation that convergence of the studies’ findings provides the evidence supporting the effectiveness of an intervention. Cumulating evidence, however, shows limited replicability of the results of studies that evaluate the same health intervention (Woodman, 2014). Limited replicability is indicated by mixed findings, with some supporting and others not supporting the effectiveness of the intervention. The literature is replete with examples of studies revealing mixed results and of systematic reviews and meta‐analyses reporting heterogeneity in the primary studies’ findings; heterogeneity precludes the synthesis of empirical evidence on the effectiveness of a health intervention. Similarly, the results of implementation studies indicate failure of evidence‐based interventions to show benefits in practice (Amrhein et al., 2017; Heneghan et al., 2017). For example, Crawford et al. (2016) reviewed the results of trials that evaluated the efficacy (under controlled research conditions) and effectiveness (under real world practice conditions) of the same simple or complex interventions. They found that, although the interventions were initially (in early efficacy studies) reported to be efficacious, 58% of simple interventions and 71% of complex ones returned negative results in subsequent effectiveness trials, implying that the interventions were no longer effective in later studies. Several factors have been suggested as contributing to the limited replicability of findings on the intervention’s effectiveness. The factors are related to weaknesses in the conceptualization of evaluation studies and in the research methodology used in these studies (Crawford et al., 2016). Conceptually, the evaluation studies were informed by a notion of causality that focuses on the direct impact of the intervention on the ultimate outcomes, and does not attend to other factors with the potential to contribute to the outcomes. The factors are inherent in the context in which the intervention is delivered and are associated with: the characteristics of the setting or environment, the interventionist, and the clients; the fidelity with which the intervention is implemented; the clients’ perceptions of the treatments included in the evaluation study; and the capacity of the intervention to initiate the mechanism of action. The focus on the direct causal effects of the intervention on the ultimate outcomes results in an emphasis on internal validity, at the expense of other types of validity, and consequently on valuing the experimental design or randomized controlled trial as the most robust in generating evidence of effectiveness. With the limited attendance to context, the findings of intervention evaluation studies provide answers to the question: Does the intervention work? They fall short of addressing questions of relevance to practice and of importance in guiding treatment decisions: What clients, presenting with which characteristics, benefit from which intervention, given in what mode, at what dose, and in what context? And how does the intervention produce the beneficial effects. This state of the science has generated some shifts in perspectives underlying intervention evaluation research, accompanied by the acceptance of various designs and methods as appropriate and useful for determining the effectiveness of health interventions in research and practice. The shifts in perspectives are represented in the adoption of the notion of multi‐causality, the emphasis on enhancing all types of validity (discussed in this chapter), and the delineation of what to evaluate and in what sequence. The shifts translated into recommendations for evaluating clients’ perceptions of health interventions (Chapter 11); the feasibility of interventions (Chapter 12); the contextual factors and the processes contributing to the implementation and effectiveness of interventions (Chapter 13); and a range of research designs (Chapter 14) and methods (Chapter 15) for examining the effects of interventions on a range of outcomes. In this chapter, the conventional perspectives on causality, validity, and the sequential phases for evaluating health interventions are briefly reviewed. Advances in the field of intervention evaluation are discussed. Underlying the systematic process for determining the effectiveness of health interventions is the notion of causality. Causality implies that the changes in the outcomes, observed following delivery of an intervention, are attributable to, or represent the impact of, the intervention. The notion of causality is evolving from the traditional perspective of single causality to the more recent view of multiple or multi‐causality. Demonstrating the effectiveness of health interventions involves the generation of evidence indicating that the intervention causes the ultimate outcomes. A cause is something that creates an effect or produces a change in a state or condition that would not happen without it (Powell, 2019). Causality refers to a structural relationship that underlies the dependence among phenomena or events (Stanford Encyclopedia of Philosophy, 2008), whereby the occurrence of one phenomenon or event is contingent on the occurrence of another. As applied to intervention evaluation, causality implies an association between the intervention (i.e. cause) and the outcome (i.e. effect). The association is characterized by the dependence of the changes in the outcome on the receipt of the intervention. In other words, the changes in the outcome take place in the presence (or exposure, receipt) of the intervention and do not occur in the absence of the intervention. This association enables the attribution of the outcomes solely and uniquely to the intervention. This notion of causality focuses on the single, deterministic, and direct association between the intervention and the ultimate outcome. It rests on the counterfactual claim that if an intervention occurs, then the effect would occur or take place and conversely, if an intervention does not occur, then the effect would not occur (Cook et al., 2010). This notion of causality and the way in which it is represented in an evaluation study have been criticized on theoretical and empirical grounds. The traditional perspective on causality is considered simplistic, ignoring the potential direct and indirect influence of a range of factors on the delivery, mechanism of action, and outcomes of health interventions (e.g. Greenhalgh et al., 2015; Wong et al., 2012). The recent perspective has extended the notion of causality to encompass chains of structural relationships among phenomena or events. The shift was engendered by the widening recognition that multiple factors, in combination with the intervention, contribute to changes in the outcomes (Chapter 5). The factors are experienced in various domains of health (e.g. physical, psychological, social) and at different levels (e.g. client, community, society). The factors, independently and collectively, predict the health problem or other outcomes; they may also interact with the intervention in shaping clients’ perceptions of, responses to, the health intervention, as well as improvement in the immediate and intermediate outcomes that mediate the effects of the intervention on the ultimate outcomes. The recent notion is that of multi‐causality. It acknowledges the interdependence among phenomena or events in that they are posited to influence each other, forming a complex system of causal relationships. The application of the notion of multi‐causality to intervention evaluation research translates into three propositions. The first is that a set of contextual factors influence directly the delivery of the intervention by interventionists, the implementation of treatment recommendations by clients, the initiation of the intervention’s mechanism of action, and the outcomes. The second suggests that contextual factors moderate the causal effects of the intervention on the outcomes. The third proposition indicates that the effects of the intervention on the ultimate outcomes are indirect, mediated by the immediate and intermediate outcomes that operationalize the hypothesized mechanism of action. The direct and indirect relationships are tested empirically to determine what exactly causes the beneficial effects of health interventions on the ultimate outcomes. The resulting evidence provides answers to the practice or clinically relevant questions of who benefits from the intervention and how does the intervention work, in what context. The intervention theory (see Chapter 5) plays an important role in delineating the complex system of causal relationships. The criteria for inferring causality commonly mentioned across fields of study (e.g. epidemiology, psychology, program evaluation) are comparable for the traditional and recent perspectives on causality. They include temporality, covariation, contiguity, congruity, and ruling out other plausible alternative causes of the intervention effects (Larzele et al., 2004). The evidence required to support each of these criteria differs slightly for the traditional and the recent perspectives. Temporality (or temporal sequence). This criterion reflects the temporal order of the cause and the effect. It is applicable to both, traditional and recent, perspectives on causality. It is typical and logical to think that the changes in the mediators (representing the mechanism of action) and in the ultimate outcomes should occur with or after the delivery of the intervention. If the changes precede delivery, then they cannot be attributed to the intervention because they occurred irrespective of the intervention. Accordingly, it is necessary to assess the mediators and outcomes before, during, and after the intervention is provided. Finding changes in the mediators and the outcomes during and following treatment is ground for inferring causality, especially when the patterns of change are consistent with the propositions of the intervention theory. Covariation. This criterion operationalizes the structural relationship and counterfactual claim that underpin simple and multi‐causality. Ideally, covariation is demonstrated when, the same clients are subjected to two conditions: (1) non‐exposure to the health intervention and (2) receipt of the intervention. Evidence supporting covariation shows no changes in the ultimate outcomes under the first condition and improvement in the outcomes under the second condition. In most situations, meeting this ideal requirement is unrealistic and logistically impossible. For instance, it may not be feasible (or ethically acceptable) to withhold treatment when the health problem is acute and experienced at high levels of severity. Therefore, it is recommended to create two groups of participants who experience the health problem addressed by the intervention under evaluation. One group receives and the other is not exposed to the intervention. Participants in both groups have to be comparable in their experience of the health problem and in their personal, health, or clinical characteristics. Evidence supporting covariation is represented in the following pattern of findings: Participants in the two groups are comparable before delivery of the intervention; participants who receive the intervention show the hypothesized changes in the mediators and the ultimate outcomes during and following the treatment period; participants who do not receive the intervention exhibit no changes in the mediators and the ultimate outcomes; participants in the two groups differ in the levels of mediators and the ultimate outcomes reported during or following the treatment period (Cook et al., 2010). Contiguity and congruity. These two criteria of causality are inter‐related. The criterion of contiguity reflects the time lag between delivery of the intervention and the occurrence of changes in the ultimate outcomes. Traditionally, the changes were expected to occur within a rather short time interval following intervention delivery. With longer time frames, other factors may take place and influence the impact of the intervention on the outcomes. Contiguity is supported by observing the hypothesized changes in the outcomes in participants who were provided the intervention, immediately (e.g. within one to two weeks) following treatment completion. The criterion of congruity has to do with the magnitude, size, or amount of the changes in the ultimate outcomes. Traditionally, the magnitude of these changes was expected to be commensurate with the nature and dose of the intervention. For instance, interventions that are highly specific to the health problem, intense, and of high dose can be logically anticipated to yield large changes in the outcomes. With the acknowledgement of multi‐causality, the contiguity and congruity criteria are reframed to account for the indirect impact of health interventions on the ultimate outcomes, mediated through the hypothesized mechanism of action. As detailed in Chapter 4 and 5, the mechanism of action proposes that a health intervention is expected to induce changes in the immediate and intermediate outcomes that mediate its effects on the ultimate outcomes. Accordingly, the time lag reflecting contiguity and the magnitude of change quantifying congruity differ for the mediators and the ultimate outcomes. For the mediators, small‐to‐moderate changes are anticipated during and following either immediately or within a relatively short time, such as one to two weeks after, treatment completion. For the ultimate outcomes, changes are expected to increase within a longer time frame after treatment completion, once changes in the mediators are produced. Therefore, the criteria of contiguity and congruity are examined simultaneously. Generating evidence supporting contiguity and congruity requires the collection of data on the mediators and the ultimate outcomes before, during, and after the intervention delivery. Relevant statistical tests are used to analyze the data. Evidence supporting these two criteria should be consistent with the following pattern of findings (see Chapter 4 and 5 for illustrative examples): Ruling out other plausible causes of the intervention effects. This criterion is applicable to both the traditional and the recent perspectives on causality, and is considered the most important or defensible warrant of causality (Cook et al., 2010). This criterion implies that the intervention effects on the mediators and consequently the outcomes are not confounded by other factors. This implies that the changes in the mediators and outcomes can be solely and uniquely attributed to the intervention. Other factors that could contribute to changes in mediators and outcomes include conceptual or substantive (e.g. characteristics of clients) or methodological (e.g. measurement). These factors introduce bias or present threat to the validity of conclusions or inferences regarding the effectiveness of the intervention (discussed in Section 10.2). Ruling out these plausible threats is done in two ways. The first entails the application of experimental control over the conditions under which the intervention is delivered. This control consists of eliminating (i.e. holding constant) possible sources of bias, as is done in the randomized controlled or clinical trial (RCT) or experimental design. For instance, the control is exerted through the selection of clients with similar characteristics and random assignment of participants to treatment conditions. Elimination of biases increases the confidence in validly attributing changes in the mediators and outcomes to the intervention. The second way to rule out possible threats involves the a priori identification of potentially confounding factors (based on the propositions of the intervention theory); collection of data on these factors; and examination of their influence on the implementation of the intervention and changes in mediators and outcomes (Nock et al. 2007; Schafer & Kang, 2008). The influence of these factors is examined in the recently proposed pragmatic approach to intervention research, as explained in Chapter 15. The primary concern in evaluation studies is to generate evidence that would support the validity of inferences or conclusions on the causal effects of the intervention. Validity refers to the approximate truth of the inferences (Shadish et al., 2002; Tengstedt et al., 2018). In other words, validity has to do with the correctness of the claim that changes in the mediators and outcomes are attributable to or caused by the intervention; that is, the claim accurately corresponds with reality (Salimi & Ferguson‐Pell, 2017; Sidani, 2015). Many conceptual and methodological factors introduce biases that threaten validity, which leads to erroneous conclusions. Three types of erroneous or incorrect inferences are frequently mentioned in the literature. Type I error. This type of error is committed when the intervention is claimed to be effective, when in reality it is not. These positive findings instigate additional research to further evaluate the effectiveness of the intervention within the same or different client populations and contexts. However, the beneficial effects found in the initial study are not reproduced or are refuted in subsequent studies. Lack of replicability is increasingly reported for different health interventions (Amrhein et al., 2017; Crawford et al., 2016; Woodman, 2014). The end result is a waste of research and related resources (Yordanov et al., 2015). Type II and III error. These two types of error are committed when the intervention is claimed to be ineffective, when in reality it is. However, they differ in the types of factor considered as contributing to the findings. A variety of factors operate in contributing to type II error, such as small sample size and unreliable measures of the mediators and the ultimate outcomes. Less‐than‐optimal delivery of the intervention (i.e. with low fidelity) is the main factor leading to type III error. Both types of error result in the abandonment of a potentially effective intervention. The term “bias” and “threat to validity” are used interchangeably to refer to any factor or process that tends to systematically (i.e. above and beyond chance) deviate or distort the inferences about the effects of the intervention, away from truth/reality (Chavalarias & Ioannidis, 2010; Kumar & Yale, 2016). Conceptual and methodological factors may introduce bias. Conceptual factors are related to the characteristics (e.g. literacy level) and behaviors (e.g. withdrawal) of clients who are exposed to the intervention; the characteristics (e.g. therapeutic relationship) of interventionists who provide the intervention; and the characteristics (e.g. accessibility of resources) of the context or environment in which the intervention is applied. The intervention theory identifies possible conceptual factors and outlines their direct or indirect (e.g. moderating) influence on the delivery, mechanism of action (i.e. mediators), and outcomes of the intervention (see Chapter 5 for examples). Methodological factors are related to the design and conduct of the evaluation study that have the potential to distort the findings, which is manifested in an over‐ or underestimation of the intervention’s effects. A wide range of methodological factors has been identified, such as those associated with attrition, measurement, and sample size. Indeed, a very large number of biases has been identified in the context of research (e.g. Shadish et al., 2002) and of practice (e.g. Lilienfeld et al., 2014). In research, the biases have been listed for different types of validity, and recently for different stages of an evaluation study. The stages and examples of biases include: Chavalarias and Ioannidis (2010) reviewed the literature to map out the types of bias. They found that 235 terms are mentioned to represent different types of bias; the most common biases can be categorized as associated with the execution of the study (e.g. confounding) and publication of findings. It is beyond the scope of this book to review all types of bias. However, the most commonly mentioned categories of bias threatening each type of validity during the study execution stage are discussed next. Four types of validity are delineated to reflect the ways in which conceptual and methodological factors weaken the accuracy of the claim regarding the causal effects of the intervention on the mediators and the ultimate outcomes. These include construct, internal, statistical, and external validity. In addition, the term “social validity” is resurging in the extant literature. The definition of each type of validity is presented. The biases that threaten each type of validity and the pathway through which the biases operate are discussed. It is important to note that different terms (used in different disciplines) are sometimes used to refer to the same bias; these are identified. Strategies to address the bias and thus to enhance validity are briefly mentioned next, and detailed in later chapters in this section of the book. Construct validity has to do with the operationalization of the concepts investigated in an evaluation study, with a primary focus on the intervention, mediators, and ultimate outcomes. The operationalization should be congruent with the conceptualization of the intervention, mediators, and ultimate outcomes as specified in the intervention theory. Nonalignment may introduce contamination or confounding that may result in incorrect inferences about the hypothesized intervention effects (Tengstedt et al., 2018). The biases threatening construct validity are: inaccurate implementation of the intervention, researcher expectancies, inaccurate measurement of the mediators and the ultimate outcomes, and clients’ reactivity to treatment and measures. Overview. As discussed in Chapter 5, the intervention theory specifies the active ingredients characterizing the intervention, and the components operationalizing them. Inadequate explication of the active ingredients may result in the delineation of components (including content, activities, and treatment recommendations) that either are not well aligned with the intended active ingredients or reflect components comprising other interventions. Accordingly, the intervention as implemented may be contaminated with components reflecting other interventions (Tengstedt et al., 2018). The deviations in the operationalization of the intervention contribute to incorrect inferences about the intervention’s causal effects because the observed changes in the mediators and the ultimate outcomes cannot be accurately attributed to the intervention as intended. Strategies. This bias can be minimized by systematically developing the intervention theory (Chapter 4 and 5) and conducting a thorough assessment of theoretical fidelity (see Chapter 9). Inaccurate implementation of the intervention also extends to its actual delivery by interventionists to participants. Shifts or variations in providing the interventions represent issues of operational fidelity, leading to type III error of inferences. These issues and strategies to address them have been discussed in detail in Chapter 6 and 9. Overview. Different terms have been used to refer to this bias: researcher or experimenter expectancy (Shadish et al., 2002), researcher therapeutic allegiance (Wilshire, 2017), and performance bias (Mansournia et al., 2017). This bias illustrates the researchers’ enthusiasm for the intervention under evaluation and their expectation that it will be successful in achieving the hypothesized beneficial effects. The enthusiasm is, intentionally or unintentionally, transferred to research staff. Research staff’s behaviors may be altered. For example, interventionists deliver the favored (by the researchers) intervention optimally and the comparison treatment poorly. Data collectors over‐rate participants’ performance on the mediators and the ultimate outcomes, and detect fewer failures or side effects among participants in the intervention group (Hróbjartsson et al., 2012). Data collectors also interact positively with participants, which translates into participants’ favorable research experience and desire to please the researchers. Data analysts conduct additional unplanned analyses to identify statistically significant effects of the favored intervention; this is well illustrated in the statement: “if you torture your data long enough, they will confess” (Fleming, 2010). The researchers’ and research staff’s enthusiasm is positively transferred to participants. Participants’ responses to the intervention are explained in detail in the section on client reactivity. Overall, researchers’ expectancies yield differences in the delivery of the intervention and the comparison treatment. Consequently, these differences in performance, more so than the intervention itself, are responsible for the observed effects quantified in the differences in the mediators and the ultimate outcomes between the intervention and comparison treatment groups. Furthermore, researcher expectancies could contribute to an overinterpretation of the results while overlooking the study limitations (Wilshire, 2017). Strategies. Three strategies are proposed to minimize this bias. First is the provision of adequate training to research staff in the skills required to assume their responsibilities and to interact with clients participating in the study, and frequent monitoring of their performance over the study period. Second is the application of the principle of blinding where possible. At a minimum, blinding involves not divulging to research staff and participants, which treatment is the experimental intervention under evaluation and which is the comparison treatment (Chapter 15). The third strategy is to consider the results of additional analyses as exploratory (rather than definitive indicators of intervention effects), requiring confirmation in future studies.
CHAPTER 10
Overview of Evaluation of Interventions
10.1 NOTION OF CAUSALITY
10.1.1 Traditional Perspective
10.1.2 Recent Perspective
10.1.3 Criteria for Inferring Causality
10.2 VALIDITY
10.2.1 Types of Erroneous Inferences
10.2.2 Types of Biases
10.2.3 Types of Validity and Related Bias
10.2.3.1 Construct Validity
Definition
Inaccurate Implementation of the Intervention
Researcher Expectancies
Inaccurate Measurement of Mediators and Ultimate Outcomes
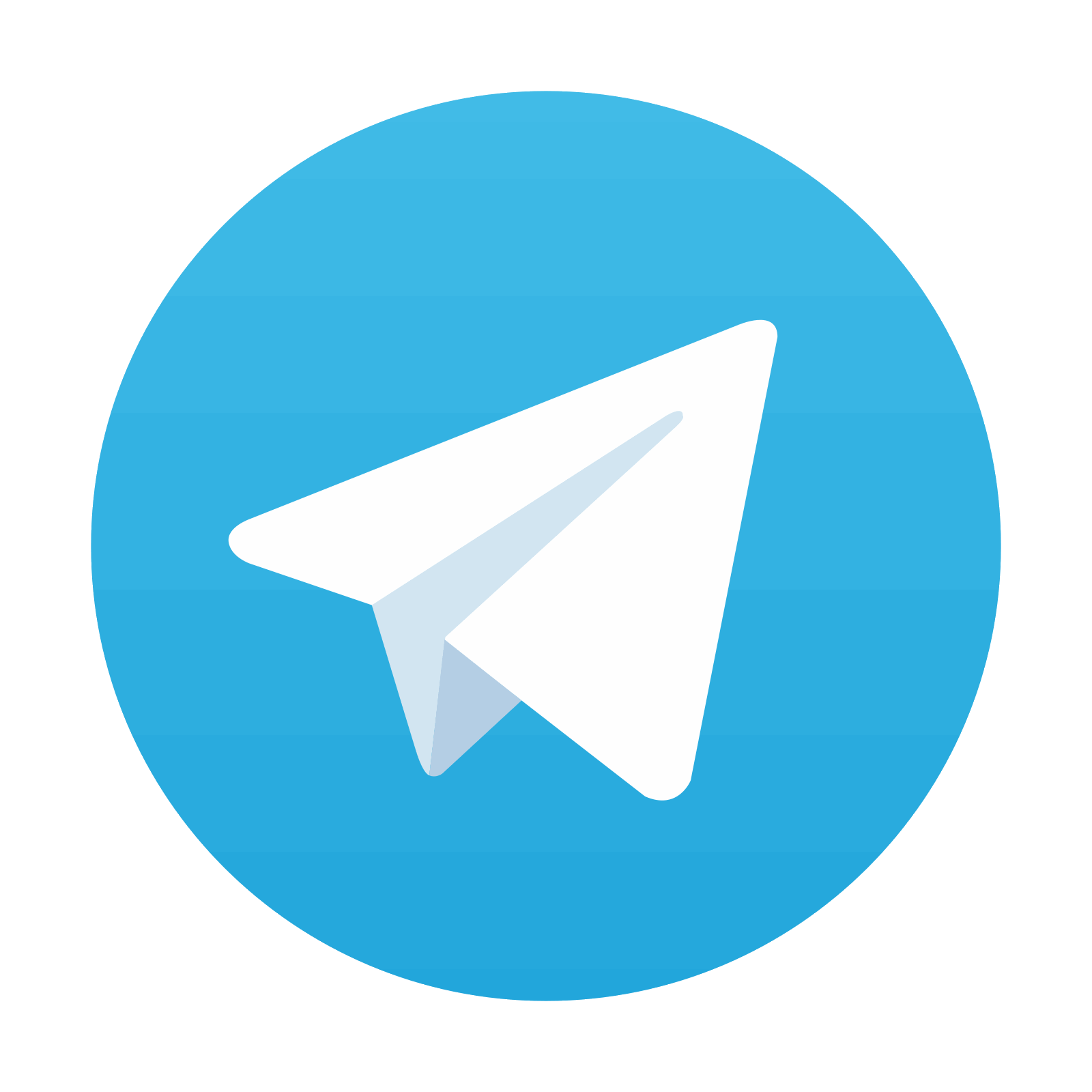
Stay updated, free articles. Join our Telegram channel

Full access? Get Clinical Tree
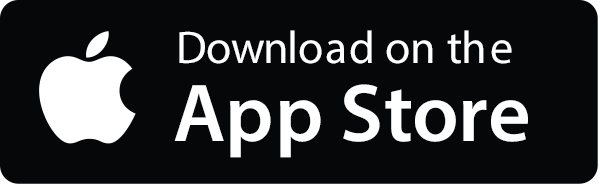
