12
TEST AND ITEM ANALYSIS: INTERPRETING TEST RESULTS
After a test is scored, the teacher needs to interpret the results and use these interpretations to make grading, selection, placement, or other decisions. To accurately interpret test scores, the teacher needs to analyze the performance of the test as a whole and of the individual test items, and to use these data to draw valid inferences about student performance. This information also helps teachers prepare for posttest discussions with students about the exam. This chapter discusses the process of performing test and item analyses. It also suggests ways in which teachers can use posttest discussions to contribute to student learning and seek student feedback that can lead to test-item improvement.
Interpreting Test Scores
As a measurement tool, a test results in a score—a number. A number, however, has no intrinsic meaning and must be compared with something that has meaning to interpret its significance. For a test score to be useful for making decisions about the test, the teacher must interpret the score. Whether the interpretations are norm referenced or criterion referenced, a basic knowledge of statistical concepts is necessary to assess the quality of tests (whether teacher-made or published), understand standardized test scores, summarize assessment results, and explain test scores to others.
Test Score Distributions
Some information about how a test performed as a measurement instrument can be obtained from computer-generated test- and item-analysis reports. In addition to providing item-analysis data such as difficulty and discrimination indexes, such reports often summarize the characteristics of the score distribution. If the teacher does not have access to electronic scoring and computer software for test and item analysis, many of these analyses can be done by hand, albeit more slowly.
222When a test is scored, the teacher is left with a collection of raw scores. Often these scores are recorded according to the names of the students, in alphabetical order, or by student numbers. As an example, suppose that the scores displayed in Table 12.1 resulted from the administration of a 65-point test to 16 nursing students. Glancing at this collection of numbers, the teacher would find it difficult to answer such questions as:
1. Did a majority of students obtain high or low scores on the test?
2. Did any individuals score much higher or much lower than the majority of the students?
3. Are the scores widely scattered or grouped together?
4. What was the range of scores obtained by the majority of the students? (Brookhart & Nitko, 2019)
To make it easier to see similar characteristics of scores, the teacher should arrange them in rank order, from highest to lowest (Miller, Linn, & Gronlund, 2013), as in Table 12.2. Ordering the scores in this way makes it obvious that they ranged from 42 to 60, and that one student’s score was much lower than those of the other students. But the teacher still cannot visualize easily how a typical student performed on the test or the general characteristics of the obtained scores. Removing student names, listing each score once, and tallying how many times each score occurs results in a frequency distribution, as in Table 12.3. By displaying scores in this way, it is easier for the teacher to identify how well the group of students performed on the exam.


TABLE 12.3 Frequency Distribution of Raw Scores From Table 12.1
RAW SCORE | FREQUENCY |
---|---|
61 | 0 |
60 | 1 |
59 | 0 |
58 | 0 |
57 | 1 |
56 | 1 |
55 | 2 |
54 | 3 |
53 | 2 |
52 | 3 |
51 | 0 |
50 | 0 |
49 | 0 |
48 | 1 |
47 | 1 |
46 | 0 |
45 | 0 |
44 | 0 |
43 | 0 |
42 | 1 |
41 | 0 |
224The frequency distribution also can be represented graphically as a histogram. In Figure 12.1, the scores are ordered from lowest to highest along a horizontal line, left to right, and the number of asterisks above each score indicates the frequency of that score. Frequencies also can be indicated on a histogram by bars, with the height of each bar representing the frequency of the corresponding score, as in Figure 12.2.
A frequency polygon is another way to display a score distribution graphically. A dot is made above each score value to indicate the frequency with which that score occurred; if no one obtained a particular score, the dot is made on the baseline, at 0. The dots then are connected with straight lines to form a polygon or curve. Figure 12.3 shows a frequency polygon based on the histogram in Figure 12.1. Histograms and frequency polygons thus show general characteristics such as the scores that occurred most frequently, the score distribution shape, and the range of the scores.

FIGURE 12.1 Histogram depicting frequency distribution of raw scores from Table 12.1.

FIGURE 12.2 Bar graph depicting frequency distribution of raw scores from Table 12.1.
Note: f, frequency; X, scores.

FIGURE 12.3 Frequency polygon depicting frequency distribution of raw scores from Table 12.1.
Note: f, frequency; X, scores.
225The characteristics of a score distribution can be described on the basis of its symmetry, skewness, modality, and kurtosis. These characteristics are illustrated in Figure 12.4. A symmetric distribution or curve is one in which there are two equal halves, mirror images of each other. Nonsymmetric or asymmetric curves have a cluster of scores or a peak at one end and a tail extending toward the other end. This type of curve is said to be skewed; the direction in which the tail extends indicates whether the distribution is positively or negatively skewed. The tail of a positively skewed curve extends toward the right, in the direction of positive numbers on a scale, and the tail of a negatively skewed curve extends toward the left, in the direction of negative numbers. A positively skewed distribution, thus, has the largest cluster of scores at the low end of the distribution, which seems counterintuitive. The distribution of test scores from Table 12.1 is nonsymmetric and negatively skewed. Remember that the lowest possible score on this test was 0 and the highest possible score was 65; the scores were clustered between 42 and 60.
Frequency polygons and histograms can differ in the number of peaks they contain; this characteristic is called modality, referring to the mode or the most frequently occurring score in the distribution. If a curve has one peak, it is unimodal; if it contains two peaks, it is bimodal. A curve with many peaks is multimodal. The relative flatness or peakedness of the curve is referred to as kurtosis. Flat curves are described as platykurtic, moderate curves are said to be mesokurtic, and sharply peaked curves are referred to as leptokurtic (Waltz, Strickland, & Lenz, 2010). The histogram in Figure 12.1 shows a bimodal, platykurtic distribution.

FIGURE 12.4 Characteristics of a score distribution.

FIGURE 12.5 The normal distribution.
The shape of a score distribution depends on the characteristics of the test as well as the abilities of the students who were tested (Brookhart & Nitko, 2019). Some teachers make grading decisions as if all test score distributions resemble a normal curve, that is, they attempt to “curve” the grades. An understanding of the characteristics of a normal curve would dispel this notion. A normal distribution is a bell-shaped curve that is symmetric, unimodal, and mesokurtic. Figure 12.5 illustrates a normal distribution.
Many human characteristics, such as intelligence, weight, and height, are normally distributed; the measurement of any of these attributes in a population would result in more scores in the middle range than at either extreme. However, most score distributions obtained from teacher-made tests do not approximate a normal distribution. This is true for several reasons. The characteristics of a test greatly influence the resulting score distribution; a very difficult test tends to yield a positively skewed curve. Likewise, the abilities of the students influence the test score distribution. Regardless of the distribution of the attribute of intelligence among the human population, this characteristic is not likely to be distributed normally among a class of nursing students or a group of newly hired RNs. Because admission and hiring decisions tend to select those individuals who are most likely to succeed in the nursing program or job, a distribution of IQ scores from a class of 16 nursing students or 16 newly hired RNs would tend to be negatively skewed. Likewise, knowledge of nursing content is not likely to be normally distributed because those who have been admitted to a nursing education program or hired as staff nurses are not representative of the population in general. Therefore, grading procedures that attempt to apply the characteristics of the normal curve to a test score distribution are likely to result in unwise and unfair decisions.
Measures of Central Tendency
One of the questions to be answered when interpreting test scores is, “What score is most characteristic or typical of this distribution?” A typical score is likely to be in the middle of a distribution with the other scores clustered around it; measures of central 227tendency provide a value around which the test scores cluster. Three measures of central tendency commonly used to interpret test scores are the mode, median, and mean.
The mode, sometimes abbreviated Mo, is the most frequently occurring score in the distribution; it must be a score actually obtained by a student. It can be identified easily from a frequency distribution or graphic display without mathematical calculation. As such, it provides a rough indication of central tendency. The mode, however, is the least stable measure of central tendency because it tends to fluctuate considerably from one sample to another drawn from the same population (Miller et al., 2013). That is, if the same 65-item test that yielded the scores in Table 12.1 were administered to a different group of 16 nursing students in the same program who had taken the same course, the mode might differ considerably. In addition, as in the distribution depicted in Figure 12.1, the mode has two or more values in some distributions, making it difficult to specify one typical score. A uniform distribution of scores has no mode; such distributions are likely to be obtained when the number of students is small, the range of scores is large, and each score is obtained by only one student.
The median (abbreviated Mdn or P50) is the point that divides the distribution of scores into equal halves (Miller et al., 2013). It is a value above which fall 50% of the scores and below which fall 50% of the scores; thus, it represents the 50th percentile. The median does not have to be an actual obtained score. In an even number of scores, the median is located halfway between the two middle scores; in an odd number of scores, the median is the middle score. Because the median is an index of location, it is not influenced by the value of each score in the distribution. Thus, it is usually a good indication of a typical score in a skewed distribution containing extremely high or low scores (Miller et al., 2013).
The mean often is referred to as the “average” score in a distribution, reflecting the mathematical calculation that determines this measure of central tendency. It is usually abbreviated as M or . The mean is computed by summing each individual score and dividing by the total number of scores, as in the following formula:
where M is the mean, ∑X is the sum of the individual scores, and N is the total number of scores. Thus, the value of the mean is affected by every score in the distribution (Miller et al., 2013). This property makes it the preferred index of central tendency when a measure of the total distribution is desired. However, the mean is sensitive to the influence of extremely high or low scores in the distribution, and, as such, it may not reflect the typical performance of a group of students.
There is a relationship between the shape of a score distribution and the relative locations of these measures of central tendency. In a normal distribution, the mean, median, and mode have the same value, as shown in Figure 12.5. In a positively skewed distribution, the mean will yield the highest measure of central tendency and the mode will give the lowest; in a negatively skewed distribution, the mode will be the highest value and the mean the lowest. Figure 12.6 depicts the relative positions of the three measures of central tendency in skewed distributions.

FIGURE 12.6 Measures of central tendency in positively and negatively skewed distributions.
The mean of the distribution of scores from Table 12.1 is 52.75; the median is 53.5. The fact that the median is slightly higher than the mean confirms that the median is an index of location or position and is insensitive to the actual score values in the distribution. The mean, because it is affected by every score in the distribution, was influenced by the one extreme low score. Because the shape of this score distribution was negatively skewed, it is expected that the median would be higher than the mean because the mean is always pulled in the direction of the tail.
Measures of Variability
It is possible for two score distributions to have similar measures of central tendency and yet be very different. The scores in one distribution may be tightly clustered around the mean, and in the other distribution, the scores may be widely dispersed over a range of values. Measures of variability are used to determine how similar or different the students are with respect to their scores on a test.
The simplest measure of variability is the range, the difference between the highest and lowest scores in the distribution. For the test score distribution in Table 12.3, the range is 18 (60 − 42 = 18). The range is sometimes expressed as the highest and lowest scores, rather than a difference score. Because the range is based on only two values, it can be highly unstable. The range also tends to increase with sample size; that is, test scores from a large group of students are likely to be scattered over a wide range because of the likelihood that an extreme score will be obtained (Miller et al., 2013).
The standard deviation (abbreviated as SD, s, or σ) is the most common and useful measure of variability. Like 229the mean, it takes into consideration every score in the distribution. The standard deviation is based on differences between each score and the mean. Thus, it characterizes the average amount by which the scores differ from the mean. The standard deviation is calculated in four steps:
1. Subtract the mean from each score (X − M) to compute a deviation score (x), which can be positive or negative.
2. Square each deviation score (x2), which eliminates any negative values. Sum all of the squared deviation scores (∑x2).
3. Divide this sum by the number of test scores to yield the variance.
4. Calculate the square root of the variance.
Although other formulas can be used to calculate the standard deviation, the following definitional formula represents these four steps:
where SD is the standard deviation, ∑x2 is the sum of the squared deviation scores, and N is the number of scores (Miller et al., 2013).
The standard deviation of the distribution of scores from Table 12.1 is 4.1. What does this value mean? A standard deviation of 4.1 represents the average deviation of scores from the mean. On a 65-point test, 4 points is not a large average difference in scores. If the scores cluster tightly around the mean, the standard deviation will be a relatively small number; if they are widely scattered over a large range of scores, the standard deviation will be a larger number.
Other Test Characteristics
In addition to interpreting the test score distribution and measures of central tendency and variability, teachers should examine test items in the aggregate for evidence of bias. For example, although there may be no obvious gender bias in any single test item, such a bias may be apparent when all items are reviewed as a group. Similar cases of ethnic, racial, religious, and cultural bias may be found when items are grouped and examined together. The effect of bias on testing and evaluation is discussed in detail in Chapter 16, Social, Ethical, and Legal Issues.
Interpreting an Individual Score
Interpreting the Results of Teacher-Made Tests
The ability to interpret the characteristics of a distribution of scores will assist the teacher to make norm-referenced interpretations of the meaning of any individual score in that distribution. For example, how should the teacher interpret P. Purdy’s score of 53 on the test whose results were summarized in Table 12.1? With a median 230of 53.5, a mean of 52.75, and a standard deviation of 4.1, a score of 53 is about “average.” All scores between 49 and 57 fall within one standard deviation of the mean, and thus are not significantly different from one another. On the other hand, N. Nardozzi can rejoice because a score of 60 is almost two standard deviations higher than the mean; thus, this score represents achievement that is much better than that of others in the group. The teacher should probably plan to counsel L. Lynch, because a score of 42 is more than two standard deviations below the mean, much lower than others in the group.
However, most nurse educators need to make criterion-referenced interpretations of individual test scores. A student’s score on the test is compared with a preset standard or criterion, and the scores of the other students are not considered. The percentage-correct score is a derived score that is often used to report the results of tests that are intended for criterion-referenced interpretation. The percentage correct is a comparison of a student’s score with the maximum possible score; it is calculated by dividing the raw score by the total number of items on the test (Miller et al., 2013). Although many teachers believe that percentage-correct scores are an objective indication of how much students really know about a subject, in fact they can change significantly with the difficulty of the test items. Because percentage-correct scores are often used as a basis for assigning letter grades according to a predetermined grading system, it is important to recognize that they are determined more by test difficulty than by true quality of performance. For tests that are more difficult than they were expected to be, the teacher may want to adjust the raw scores before calculating the percentage correct on that test.
The percentage-correct score should not be confused with percentile rank, often used to report the results of standardized tests. The percentile rank describes the student’s relative standing within a group and therefore is a norm-referenced interpretation. The percentile rank of a given raw score is the percentage of scores in the distribution that occur at or below that score. A percentile rank of 83, therefore, means that the student’s score is equal to or higher than the scores made by 83% of the students in that group; one cannot assume, however, that the student answered 83% of the test items correctly. Because there are 99 points that divide a distribution into 100 groups of equal size, the highest percentile rank that can be obtained is the 99th. The median is at the 50th percentile. Differences between percentile ranks mean more at the highest and lowest extremes than they do near the median.
Interpreting the Results of Standardized Tests
The results of standardized tests usually are intended to be used to make norm-referenced interpretations. Before making such interpretations, the teacher should keep in mind that standardized tests are more relevant to general rather than specific instructional goals. In addition, the results of standardized tests are more 231appropriate for evaluations of groups rather than individuals. Consequently, standardized test scores should not be used to determine grades for a specific course or to make a decision to hire, promote, or terminate an employee. Like most educational measures, standardized tests provide gross, not precise, data about achievement. Actual differences in performance and achievement are reflected in large score differences.
Standardized test results usually are reported in derived scores such as percentile ranks, standard scores, and norm group scores. Because all of these derived scores should be interpreted in a norm-referenced way, it is important to specify an appropriate norm group for comparison. The user’s manual for any standardized test typically presents norm tables in which each raw score is matched with an equivalent derived score. Standardized test manuals may contain a number of norm tables; the norm group on which each table is based should be fully described. The teacher should take care to select the norm group that most closely matches the group whose scores will be compared to it (Miller et al., 2013). For example, when interpreting the results of standardized tests in nursing, the performance of a group of baccalaureate nursing students should be compared with a norm group of baccalaureate nursing students. Norm tables sometimes permit finer distinctions such as size of program, geographical region, and public versus private affiliation.
Item Analysis
In addition to test statistics, teachers also should examine indicators of performance quality for each item on the exam. When used together, multiple data points—difficulty index, discrimination index, and point biserial correlation coefficient—provide a rich source of information about the performance quality of test items (Ermie, n.d.). However, teachers should not depend solely on these statistical data to judge the quality of exam items. Decisions about individual test items should be made in the context of the content and structure of the item, the teacher’s expectations about how the items would perform, and an accurate interpretation of the item statistics (Brookhart & Nitko, 2019).
Computer software for item analysis is widely available for use with electronic answer sheet scanning equipment. Commercially available computer testing applications usually also provide services that produce user reports of item-analysis statistics. For teachers who do not have access to such equipment and software, procedures for analyzing student responses to test items by hand are described in detail later in this section. Regardless of the method used for analysis, teachers should be familiar enough with the meaning of each item-analysis statistic to correctly interpret the results.
Exhibit 12.1 offers an example of a computer-generated item-analysis report. This example lists only the item-analysis data for each of the exam items, without also including the wording of the items and any codes that the teacher may have used to 232classify the content of the items (e.g., content domain, cognitive level, client needs). This format is useful for quickly scanning the data to identify potential problems. Later examples will illustrate item-analysis reports that display the item content, any classification codes, and additional statistics related to the performance of each item’s answer options.
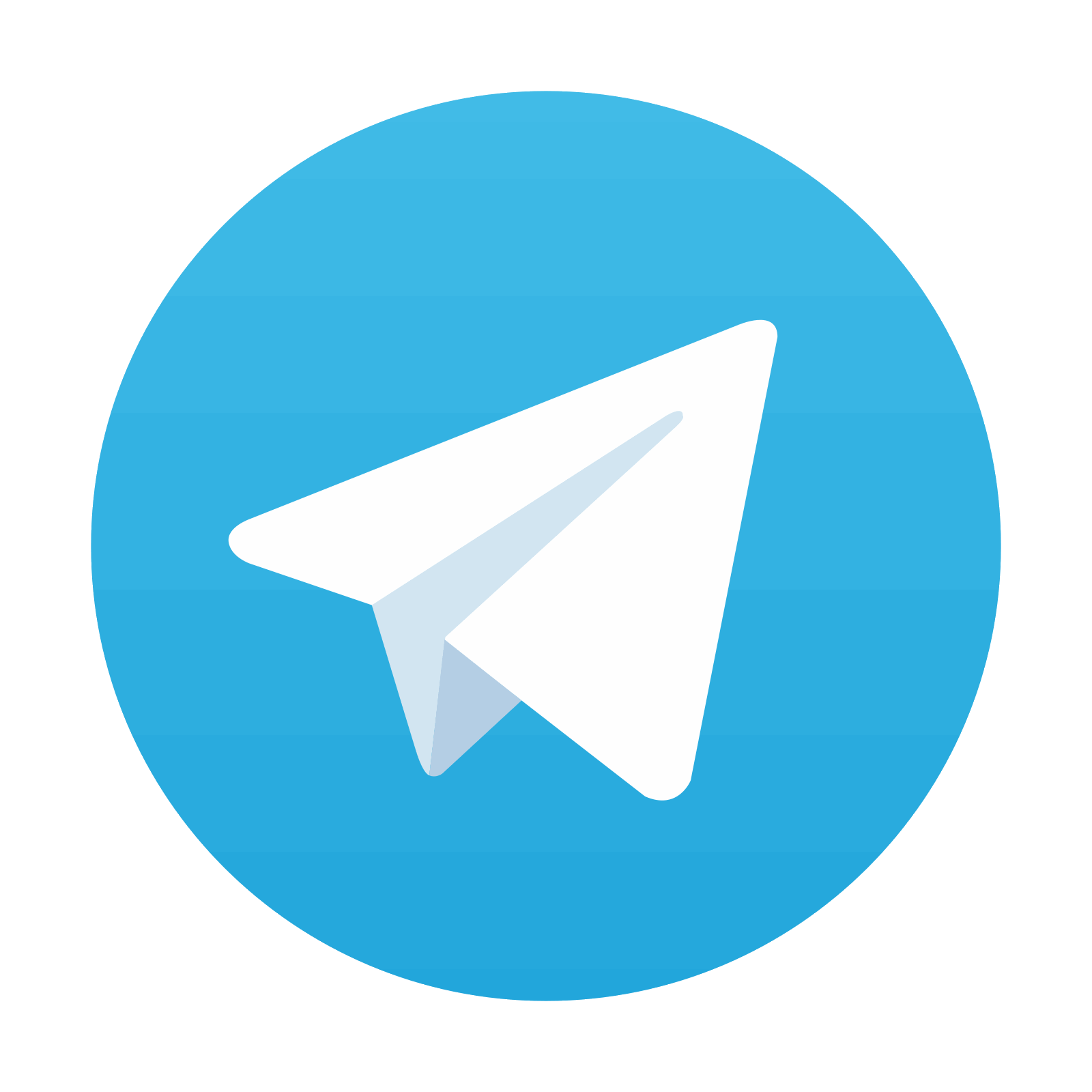
Stay updated, free articles. Join our Telegram channel

Full access? Get Clinical Tree
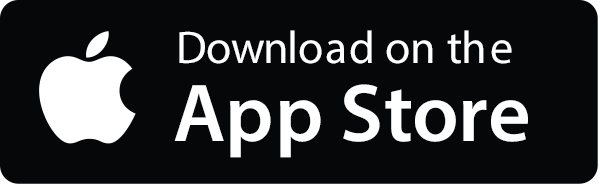
