8687CHAPTER 6
Measuring Outcomes in Cardiovascular Advanced Practice Nursing
Anna Gawlinski, Kathy McCloy, Virginia Erickson, Elizabeth Vandenbogaart, and Anna Dermenchyan
Chapter Objectives
1. Identify outcomes of concern to advanced practice registered nurses (APRNs) and compare these to the outcomes currently being measured in your institution and your patient population
2. Discuss advantages and disadvantages of each of the methods that are used for outcome measurement projects (e.g., research, research utilization, evidence-based practice [EBP], and quality improvement [QI] frameworks)
3. Analyze the relative effectiveness of interventions that have been shown to decrease medication discrepancies
4. Discuss the role of the APRN in working with interdisciplinary teams to improve outcomes in patients with cardiovascular disease
Chapter Discussion Questions
1. How does the medication reconciliation process defined in this chapter compare with the interventions implemented by the APRNs in the exemplar medication reconciliation outcome measurement project?
2. How do the outcomes of concern to APRNs listed in Exhibit 6.1 compare with outcomes currently being measured in your institution and your patient population?
3. Identify two to three clinical issues and related outcomes in your practice. Which research designs or other frameworks would be most appropriate to use in an outcome measurement project? Why?
884. How effective are the interventions described in the literature in decreasing medication discrepancies? Design a study to address the medication discrepancy issue for your patient population and discuss why you chose that design.
5. Discuss the interdisciplinary team’s project to improve outcomes in patients with heart failure (HF). What aspects of the project could be beneficial to your own patient population?
EXHIBIT 6.1 Select Outcomes for Advanced Practice Nursing
Clinical (Care-Related) Outcomes Mortality Morbidity Infection: hospital associated, urinary tract infection, ventilator or catheter related Hand hygiene compliance rates Developing medical conditions such as acquiring heart failure from uncontrolled hypertension Loss of motor function Physiological response Blood pressure, heart rate Temperature Lung sounds Hemodynamic pressures Weight and weight management Serum/urine level of glucose Wound healing, skin integrity Symptom management Pain Fatigue Nausea, vomiting Nutritional status/management Sleep maintenance Restraint use Smoking cessation Substance use/abuse (illicit or prescriptive) Low birth weight, preterm infants Rates of adherence to best practices |
Psychosocial Outcomes Coping, stress management Mentation Return to work Role functioning Family functioning/coping Anxiety 89Depression Sexual functioning Caregiver strain/burden Knowledge patient/caregiver: disease/condition, medications, diet, treatment regime, psychomotor skills Health literacy Cultural sensitivity Underserved populations Staff nurse knowledge |
Functional Outcomes Quality of life Self-care: bathing, eating, dressing self, administration of nonparenteral medication Mobility Communication Return to Work School Normal activity/social interaction Symptom control |
Fiscal Outcomes Length of stay (hospital and/or intensive care unit) Readmission rates to hospital, home care, other services Emergency department visits Health care services utilization Cost per episode of care Resource utilization: ancillary services, community/other services Patient flow, improve throughput Access and barriers to health care Disparities in care received and outcomes Savings from APRN practice Staff nurse retention rates |
Satisfaction Patient experience Care provided Services provided Care provider Family Care provided to family member Services provided/available Payer Provider |
APRN, advanced practice registered nurse.
Source: Adapted from Urden (1999); Kleinpell-Nowell and Weiner (1999); and Newhouse et al. (2011).
90National health care policy makers and health care professionals have increasingly advocated for the measurement and monitoring of patient safety, QI, and health care outcomes. The development and implementation of national practice guidelines that are based on the best available research have provided clinicians with interventions that can improve patients’ outcomes (McClellan, McGinnis, Nabel, & Olsen, 2007). Yet these practice guidelines are not consistently used, and practices vary from clinician to clinician and from institution to institution, resulting in poor outcomes for patients (Centers for Medicare & Medicaid Services [CMS], 2012; Institute of Medicine, 2001; Melnyk & Fineout-Overholt, 2015).
This emphasis on EBP has resulted in increased focus and incentives for those institutions and providers that perform well on indicators of safety and quality, with measurable outcomes. For example, the CMS and The Joint Commission (TJC) have set standards for performance measurement for patients hospitalized with HF that include measures of use of research-based therapies, such as angiotensin-converting enzyme inhibitors and beta-blockers, as well as reduction in hospital readmission rates (CMS, 2011; TJC, 2012).
Cardiovascular disease in particular lends itself to measurement of such quality indicators and outcomes. Cardiovascular disease is the leading cause of mortality and health care cost for men and women in the United States (Mozaffarian et al., 2016). These statistics, along with the high acuity and chronicity of cardiovascular disease and the availability of published national guidelines outlining “best practices,” contribute to the pressing need for outcome measurement in the field of cardiovascular nursing (Mozaffarian et al., 2016).
Although a great deal of effort has been devoted to developing EBP guidelines for cardiovascular disease, more data are needed to demonstrate how these guidelines can be translated into practice and what their subsequent effect is on outcomes in everyday clinical settings. Cardiovascular APRNs are in a key position to use their expert knowledge of research-based practices and outcome measurement to generate data that demonstrate successful translation of these guidelines into the clinical setting.
The purpose of this chapter is to provide an overview of outcome measurement in advanced practice nursing, discuss methods that can be used for outcome measurement, and describe the role of the cardiovascular APRN in outcome measurement. An outcome measurement project is presented to demonstrate the unique role of the APRN in using an EBP approach to implement changes that result in positive measurable outcomes. A discussion follows that demonstrates how the APRN-initiated outcome project provided important preliminary data for a system-wide QI initiative to improve outcomes in patients with HF. The contributors’ aim is to present exemplars of outcome projects and share processes that can be replicated to reduce variations in practice and improve outcomes in cardiovascular patients.
CLINICAL OUTCOMES
Clinical and patient outcomes are defined as the end results of care that can be attributed to the health care services provided, such as treatments, procedures, and planned interventions (Kleinpell & Alexandrov, 2014). Outcomes are the consequences of 91treatment or interventions (Finkelman & Kenner, 2012; Kleinpell & Alexandrov, 2014). They can be used to characterize the results (effect) of an intervention, treatment, or system-level process of care. Clinical outcomes demonstrate the value and effectiveness of care and can be assessed for individuals, populations, and organizations (Kapu & Kleinpell, 2013).
Outcomes are often quantified or measured through the use of indicators, which are referred to as metrics. Outcome indicators or metrics provide estimates that reflect the degree to which patients are affected by their care (Stanik-Hutt, 2012). Indicators must be valid and reliable measures that are related to the outcome of interest. For example, to measure the adequacy of cholesterol management (a clinical outcome) in a patient with coronary artery disease, indicators of this outcome would include levels of various components of a patient’s blood lipid panel such as the total cholesterol, the low-density lipoprotein (LDL), and the high-density lipoprotein (HDL). Indicators provide a picture of the progress toward achievement of the outcome, whereas outcomes can be considered predictors of end performance (Finkelman & Kenner, 2012).
Indicators or metrics that are reported to agencies outside the internal organization are referred to as performance measures (Finkelman & Kenner, 2012). In the past two decades, a wide array of standardized health care performance measures have been developed, and a large number of these have been endorsed by the National Quality Forum (NQF). The NQF is a private, not-for-profit membership organization whose purpose is to develop a national strategy for health care quality measurement and reporting. The NQF currently develops, reviews, and measures performance standards for health care, and promotes national standardization of these quality performance measures (Finkelman & Kenner, 2012).
A substantial growth has occurred in the number of entities using health care performance measures for a variety of purposes. For example, quality and efficiency performance measures are now embedded throughout the U.S. health care system. The purposes for their widespread use are (a) QI, (b) public reporting, (c) regulation (e.g., accreditation, certification, credentialing, and licensure), and (d) payment applications (e.g., financial incentives, tiered payment; Damberg et al., 2012).
Recently, the Patient Protection and Affordable Care Act (2010) authorized the Hospital Value-Based Purchasing (VBP) program as an initiative focused on improving hospital performance for quality measures related to clinical processes of care, hospital-acquired conditions, outcomes of care, and the patients’ experience of care. In this program, CMS withholds a portion of hospital reimbursement each year and redistributes these funds as incentive payments to hospitals on the basis of their performance on these measures (CMS, 2011).
Performance measures are often benchmarked or compared with other institutions. Benchmarking is a process to identify best practices, which, when implemented, can lead to superior performance (DesHarnais, 2013). It is the use of external comparisons to understand how one is doing compared to one’s peers and/or one’s competitors (practitioners or institutions). Data of performance measures are compared between health care systems or within a single health care system (DesHarnais, 2013). These comparisons allow APRNs to identify areas of strengths and weaknesses in relation to best practice.
92 NURSING OUTCOMES
The care of patients often requires the expertise of several health care professionals. When many health care providers interact with patients and contribute to their care, it may be difficult to attribute successful clinical patient outcomes to any one provider or treatment. Thus, identifying outcomes that can be attributed only to nursing care can be a challenge, because attribution requires a high level of confidence that the outcome is a direct result of that provider’s care (Dennison & Hughes, 2009).
For example, an HF patient who was discharged and begins to experience symptoms of increasing dyspnea and weight gain at home may be treated by several health care providers (RN, APRN, and registered dietitian [RD]). At hospital discharge, the RN provided the patient with detailed discharge instructions regarding the important aspects of HF management, including specific instructions regarding symptoms to immediately report to his or her health care provider (i.e., dyspnea and/or weight gain, 2 pounds in 24 hours or 5 pounds in 4 days). The RD counseled the patient in-hospital, reviewed the recommended guidelines for a low-sodium diet, and provided instructions about reading labels and interpreting sodium content.
The cardiovascular APRN was notified of the new onset of the patient’s symptoms and asked the patient to schedule a clinic appointment. During the clinic appointment, the APRN assesses for contributing factors, such as physiologic changes in cardiac function, medication nonadherence issues, and dietary indiscretion. On the basis of the assessment, the APRN orders additional diagnostic tests (e.g., brain natriuretic peptide, echocardiogram), reinforces education regarding the need for the patient to adjust diuretic therapy on the basis of daily weight, and reinforces specifics about medication and dietary regimens. A referral is also made to the RD. The RD reviews the recommended low-sodium diet and helps the patient make better food selections. Upon follow-up, when the patient reports less dyspnea and decreased weight, the question arises as to which health care professional was responsible for the achievement of these positive outcomes. Was it the RN, the APRN, or the RD, or the whole team (Stanik-Hutt, 2012)?
Attempts to measure outcomes that can be attributed to nursing care have resulted in a definition of nurse-sensitive outcomes. Nurse-sensitive outcomes are defined as outcomes that are sensitive enough to measure the effect of nursing practice (Finkelman & Kenner, 2012; Joseph, 2007). They represent the impact of nursing interventions and describe the effect of what nurses do in response to the patient’s condition. Several nursing care outcomes that reflect nursing performance have been selected for national reporting. These nursing care outcomes include measures of patient-centered outcomes such as mortality among surgical inpatients with treatable serious complications, prevalence of pressure ulcers, falls (with and without injury), use of restraints, and hospital-associated infections such as catheter-related urinary tract infections, central-catheter-associated bloodstream infections, and ventilator-associated pneumonias. Additional measures include nursing-centered intervention processes, such as smoking-cessation counseling, and system-centered structures and processes such as nursing staff skill mix and nursing care hours per patient day (Finkelman & Kenner, 2012; Kurtzman & Corrigan, 2007; Stanik-Hutt, 2012).
Researchers at the University of Iowa have provided leadership in the area of nurse-sensitive outcomes by creating the nursing interventions classification (NIC) 93and nursing outcomes classification (NOC), which link nursing interventions to diagnoses and outcomes. This research team has contributed to identifying outcomes and related measures at the individual patient, family, and community levels, which can be used to evaluate nursing care across the patient care continuum. Individual patients’ outcome data can be aggregated in a number of ways to assess the effectiveness of nursing care within an organization and across various settings (Moorhead, Johnson, Maas, & Swanson, 2008). This research team is disseminating and publishing their work to facilitate more consistent documentation of nursing interventions and outcomes.
CLASSIFICATION OF OUTCOMES
Historically, the classification of health care outcomes has used medical definitions known as the “five Ds”: death, disease, disability, discomfort, and dissatisfaction (Gawlinski et al., 2013; Lohr, 1988; Urden, 1999), but classification of outcomes varies. For example, outcomes can be categorized as generic and broad-based outcomes that pertain to all patients and health care providers (e.g., quality of care, access, cost, patients’ satisfaction, and utilization of service). Outcomes have also been categorized in other ways, such as patient/care related, system related, practitioner or performance related, and cost/financial related (Kleinpell & Alexandrov, 2014). Other categories of outcomes are clinical, psychological, functional, and satisfaction related (Kleinpell & Alexandrov, 2014; Urden, 1999).
OUTCOME MEASURES USED IN ADVANCED PRACTICE NURSING
APRNs are often faced with the dilemma of what constitutes an outcome and which outcomes should be measured. The outcomes that best reflect clinical practice and the goals of treatment are the most meaningful and most amenable to measurement (Gawlinski, 2007; Gawlinski et al., 2013). Several websites (Table 6.1) and publications provide APRNs with excellent resources on selected health outcome information and specific outcome measures and instruments (Academy Health, 2011; Gawlinski et al., 2013; Moorhead et al., 2008). For example, Fulton and Baldwin (2004) published an annotated bibliography reflecting clinical nurse specialist practice and outcomes (Fulton & Baldwin, 2004). Urden (1999) published a list of a broad spectrum of outcomes using clinical, physiological, psychological, functional, fiscal, and satisfaction categories (Urden, 1999). Newhouse et al. (2011) presented a systematic review of APRN outcomes from 1990 to 2008 examining patient satisfaction, patient self-reported perceived health, functional status, glucose, lipid and blood pressure control, duration of mechanical ventilation, emergency department (ED) or urgent care visits, hospitalizations, length of stay, cost, complications, and mortality (Newhouse et al., 2011). This systematic review demonstrated that APRN care promotes patient access to care, reduces complications, and results in improved patient knowledge, self-care management, and patient satisfaction. Kleinpell (2003) provided a list of sources for identifying outcome measures and outcome instruments for analyzing the impact of APRN care (Kleinpell, 2003). Exhibit 6.1 provides a listing of select outcome measures that can be used by APRNs according to these published literature reviews.
94TABLE 6.1 Selected Health Outcome Information Websites
Organization | Website |
Academy for Healthcare Research and Quality | |
Centers for Disease Control and Prevention (CDC) | |
Centers for Medicare & Medicaid Services | |
Health Resources and Services Administration (HRSA) | |
Healthy People 2020 | |
Institute for Healthcare Improvement (IHI): Transforming Care at the Bedside | www.ihi.org/engage/initiatives/completed/TCAB/Pages/default.aspx |
Institute for Healthcare Improvement | |
Institute of Medicine of the National Academies | |
International Society for Quality of Life Research (ISOQOL) | |
The Joint Commission on Accreditation of Healthcare Organizations | |
National Academy of Medicine (formerly Institute of Medicine) | |
National Database of Nursing Quality Indicators® | www.pressganey.com/solutions/clinical-quality/nursing-quality |
National Guideline Clearinghouse (NGC) | |
National Committee for Quality Assurance | |
National Quality Measures Clearinghouse (NQMC) | |
National Patient Safety Foundation | |
Nursing Quality Forum | |
Patient-Reported Quality of Life Instruments Database | |
Registered Nurses Association of Ontario—“Clinical Practice Guidelines Program” | |
University of Alberta—“Evidence-Based Medicine Toolkit” | |
University of Iowa College of Nursing | www.nursing.uiowa.edu/cncce/nursing-outcomes-classification-overview |
U.S. Department of Health and Human Services—Hospital Compare | |
U.S. National Library of Medicine (NLM)/HTA 101: Introduction to Health Technology Assessment | www.nlm.nih.gov and www.nlm.nih.gov/nichsr/hta101/ta10101.html |
In 2011, Academy Health issued a list of publications (books and journals) and electronic resources (bibliographic databases and websites) that provides health care professionals with a comprehensive library of core and essential health outcome 95resources. This comprehensive list of health outcome resources can be accessed at www.nlm.nih.gov/nichsr/corelib/houtcomes-2011.html and has been reviewed and revised.
APRNs are at the forefront of improving care through outcome measurement. They serve as critical members of the health care team. Because of their key role in the health care system, APRNs frequently lead outcome measurement and QI initiatives. By virtue of their graduate education preparation, clinical knowledge, and critical thinking skills, APRNs have an essential role in evaluating outcomes for improvement efforts. Additionally, APRN core competencies demonstrate expertise and provide additional areas for measuring outcomes. These core competencies include expert coaching and advice, consultation, research skills, clinical and professional leadership, collaboration, and ethical decision making (Hamric, Hanson, Tracy, & O’Grady, 2012). For these reasons, as well as the APRNs’ consistent presence with patients and their patient advocacy role, APRNs frequently possess an integrated, holistic, and broader view of what constitutes an important outcome. Using this view, APRNs not only measure traditional physiologic outcomes, but also include outcomes related to psychosocial, functional, behavioral, symptoms, quality of life (QOL), knowledge, and satisfaction. Because a change in one outcome may influence changes in another outcome, APRNs are interested in the interaction and relationship of these outcomes. For example, exacerbation of a patient’s symptoms can interfere with a patient’s physiologic, psychosocial, and functional status and with QOL. APRNs are especially interested in the management and control of symptoms.
In APRN practice, examples of physiologic outcomes of concern may include pulse, blood pressure, lipid levels, blood glucose levels, weight, and other physiologic parameters. Relevant psychosocial outcomes may include the patient’s mood, attitudes, and abilities to interact with others. For functional outcomes, a patient’s mobility, physical independence, and ability to participate in desired activities of daily living would be considered important. Behavioral outcomes of significance may include adequacy of coping with health care needs or a patient’s ability to follow (adhere to) recommended care. Symptoms, such as pain, dyspnea, and fatigue would require an assessment and evaluation independent from the diseases that cause them. QOL is another natural outcome of interest for APRNs. QOL is defined as a patient’s general perception of his or her physical and mental well-being that can be affected by many factors including disease and injury, stress and emotions, symptom control and functional status, as well as others. Finally, the patient’s knowledge level would be a valuable outcome to measure for APRN practice. This measure could include an individual patient’s understanding of health-related information such as his or her disease/condition, medications, diet, and aspects of treatment regime. Although patient satisfaction is a quality indicator for all health care providers, patient satisfaction with the care, communication, and compassion provided by the APRN would be particularly meaningful feedback for the individual practitioner (Stanik-Hutt, 2012).
Outcomes specific to the role of the APRN are also of interest. These types of role-based outcomes are frequently related to the health care system and to cost. They may include clinic wait times, hospital length of stay, bed occupancy rate, timely discharge, cost per adjusted discharge, and so forth.
96Typically, the APRN is part of a larger entity, where multiple clinicians work as a health care team. Consequently, identifying outcomes specific to the impact of APRN care may not be a standard component of an institution’s outcome measurement program. For example, Moote, Krsek, Kleinpell, and Todd (2011) reported that health care organizations that employed 200 or more APRNs did not consistently collect focused outcomes to assess the specific contribution of APRN care (Moote et al., 2011). However, researchers in some studies (Albert et al., 2010; Moote et al., 2011; Newhouse et al., 2011) have evaluated APRN utilization and/or comparability with other health care providers. Results of these studies indicate that patients’ outcomes of care provided by APRNs in collaboration with physicians are similar and in some ways better than care provided by physicians alone for the populations and settings included (Newhouse et al., 2011). Albert et al. (2010) examined the influence of care by APRNs and physician assistants (PAs) on the delivery of guideline-recommended therapies for outpatients with HF (Albert et al., 2010). Compared to no APRNs or PAs, a cardiology practice with more than 2.0 APRNs or PAs was associated with greater use of implantable cardioverter defibrillator therapy, delivery of HF education, and equivalent use of drug and cardiac resynchronization therapies. Utilization of APRNs and PAs in academic health centers was initially cited as primarily for resident duty hour restrictions. However, secondary reasons for employing APRNs and PAs included improving patient throughput, increasing patient access, improving patient safety, reducing length of stay, and improving continuity of care (Moote et al., 2011). To date, further research is needed that identifies and tests metrics specific to the measurement of APRN practice. With the development of further regulatory agency requirements, such as CMS pay for performance initiatives (CMS, 2011), and the TJC practice standards (TJC Resources, 2011), measuring and highlighting APRN-specific outcomes is an important and essential component of advanced practice nursing (Kapu & Kleinpell, 2013).
Generally, outcome measures in the cardiac population have focused on phenomena such as the effect of an aggressive cholesterol management program implemented by an APRN, the effect of applying EBPs to manage HF, the effect of APRN practice on management and prevention of postoperative complications, interventions to promote QOL, and evaluation of a spectrum of physiological responses to APRN interventions (e.g., blood pressure, heart rate, hemodynamics, urine output, daily weight, nutrition, and symptom control; Becker et al., 2005; Bergenson & Dean, 2006; De la Porte et al., 2007; DeWalt et al., 2006; Kutzleb & Reiner, 2006; Meyer & Miers, 2005; Paez & Allen, 2006; Paul, 2000). Sample outcome measures that are of concern in the cardiovascular population are listed in Exhibit 6.2.
In an era of high-quality, safe, effective, and cost-containing health care, several professional organizations have taken the lead to form expert panels that identify EBPs and associated quality and performance measures for select high-volume and high-risk patient populations. This is especially true in the area of prevention, diagnosis, and treatment of patients at risk or patients with cardiovascular disease. For example, in 2010, the American Heart Association (AHA) identified Strategic Impact Goals for cardiovascular disease and stroke. These goals include that by 2020, cardiovascular health of all Americans will improve by 20%, while simultaneously reducing deaths from cardiovascular diseases and stroke by 20%. To help achieve this goal, the AHA created Life’s Simple 7 Metrics. Four of the metrics are related to health behaviors (i.e., diet quality, physical activity, smoking cessation, body mass index [BMI]) and three of the metrics are related to health factors (i.e., serum level cholesterol, blood pressure, blood glucose level). These metrics include targets for individual patients and clinicians to achieve. Thus, APRNs are in a unique position to focus on these goals to improve overall cardiovascular health and reduce risk. APRNs are now challenged to develop and test novel and robust strategic interventions for individual patients, populations, and the health care system that aid in achieving these goals. Organizations and their related website links to these performance outcome measures can be found in Table 6.2; sample outcomes of concern for select cardiovascular populations can be found in Exhibit 6.2.
97EXHIBIT 6.2 Selected Outcomes for the Cardiovascular Population
Reduction of Cardiac Risk Factors Control of hypertension (blood pressure) Diabetes control (blood glucose, glucose A1c) Body mass index, obesity, weight loss Lipid (cholesterol, high- and low-density lipoproteins, triglycerides) Physical activity Diet quality (e.g., low fat, low sodium, low sugar intake) Knowledge: medications, diet, treatment regimen, motor skills, condition specific Smoking cessation Alcohol intake Obstructive apnea screening Family history/genetic screening and counseling Self-care interventions Patient adherence |
Acute Coronary Syndrome Guideline-directed therapy (i.e., cardioprotective medication regimen) Frequency prescribed Frequency of reaching target goals for lipid levels Presence or absence of chest pain Presence or absence of arrhythmias Resuscitation outcomes: CPR/defibrillation bystander/EMS/in hospital; time to treatment, survival Time to BCLS, defibrillation, ACLS (bystander, EMS, in-hospital) Time to hypothermia treatment intervention protocol Time to reperfusion Access and barriers to care Gender/disparity differences Depression Resource utilization Echocardiograms, EKGs, blood tests, chest radiographs, etc. Pharmacist, case manager, home health, dietician, social services, physical therapy, etc. Length of stay (hospital, cardiac care unit) Cost per case Functional status Cardiac rehabilitation referral and attendance (or participation in exercise program) In addition to those in Reduction of Cardiac Risk Factors |
Heart Failure Readmission rates Symptom management 98Guideline-directed management therapy Frequency prescribed Adherence to medication regimen Daily weight monitoring Fluid limit Adherence to low-sodium diet Obstructive sleep apnea screening Referral for implantable cardioverter defibrillator and device management Pulmonary artery pressure sensor measurements (referral for implant effect of monitoring) Functional status Referral to cardiac rehabilitation and attendance (or participation in exercise program) Anxiety Depression Mortality rates Referral for palliative care Referral for mechanical assist device and device management Referral for advanced therapies such as heart transplant Resource utilization Echocardiograms, EKGs, blood tests, chest radiographs, etc. Pharmacist, case manager, home health, dietician, social services, physical therapy, etc. In addition to those mentioned previously in Reduction of Cardiac Risk Factors |
After Percutaneous Transluminal Angioplasty/Stent Placement Reocclusion rates Hematoma rates Bleeding requiring transfusion Other vascular complications Guideline-directed management therapy Antiplatelet and antilipid therapy Functional status before and after Sheath removal techniques and products In addition to those mentioned previously in Reduction of Cardiac Risk Factors |
After Coronary Artery Bypass Graft Pain control Sedation Hemodynamic stability Early extubation Blood transfusion Early mobility 99Infections Surgery related (e.g., sternal, saphenous vein graft) Hospital associated Wound healing, skin integrity Cardioprotective meds Prescribed Adherence Functional status Referral to cardiac rehabilitation and attendance (or participation in exercise program) Quality of life Depression Cognition |
ACLS, advanced cardiac life support; BCLS, basic cardiac life support; CPR, cardiopulmonary resuscitation; EMS, emergency medical services.
INSTRUMENTS AND APPROACHES TO OUTCOME MEASUREMENT
The use of valid and reliable measures and instruments is important in an outcome project and contributes to the level of confidence that one can have in the results (Polit & Beck, 2017a). Many valid and reliable instruments can be used to measure common health care outcomes such as QOL and functional status (Polit & Beck, 2017a); such instruments include self-report questionnaires or scales, symptom checklists, visual analog scales, and numeric rating scales (Nolan & Mock, 2000; Polit & Beck, 2017b).
In general, three major approaches can be used for outcome measurement: (a) outcome measurement evaluation, (b) outcome management, and (c) outcome research. Outcome evaluation is the monitoring and/or measuring of the extent to which providers meet the clinical or cost outcome goals of their patients or institutions. Outcome management is a systematic improvement of outcomes by acting on information gained from outcome measurement, often using the tools of continuous QI. Outcome research is a type of controlled, empirical assessment of the effect of a given intervention, product, or technology on patient, cost, or service outcomes (Polit & Beck, 2017c). The determination of which approach is used is dependent on the outcome of interest, available resources, and feasibility. Within these three major approaches, several methods can be used for outcome measurement projects, and these are discussed in the following section.
100TABLE 6.2 Selected Organization Websites and Quality Measures for Cardiovascular Populations
Organization Guideline and Website | Quality Measures for Selected Populations |
AHA Heart Disease and Stroke Statistics 2016 Update: Chapters 1–13 http://circ.ahajournals.org/content/circulationaha/early/2015/12/16/CIR.0000000000000350.pdf | Quality measures for cardiovascular risk reduction |
AHA Heart Disease and Stroke Statistics 2016 Update: Chapters 17, 19, and 20 http://circ.ahajournals.org/content/circulationaha/early/2015/12/16/CIR.0000000000000350.pdf | Quality measures for acute myocardial infarction |
2014 AHA/American College of Cardiology (ACC) Guideline for the Management of Patients with Non-ST-Elevation Acute Coronary Syndromes: Chapter 8 http://content.onlinejacc.org/article.aspx?articleid=1910086 | Quality measures for non-ST-elevated acute coronary syndrome |
AHA Get With the Guidelines ACTIONS Registry, and GWTG Resuscitation | Quality measures for after resuscitation |
American College of Cardiology Foundation (ACCF)/AHA/American Medical Association (AMA)–Physician Consortium for Performance Improvement (PCPI) 2011 Performance Measures for Adults with Heart Failure | Quality measures for heart failure |
AHA Heart Disease and Stroke Statistics 2016 Update: Chapters 20 and 23 http://circ.ahajournals.org/content/circulationaha/early/2015/12/16/CIR.0000000000000350.pdf | Quality measures for heart failure |
AHA Get With the Guidelines ACTIONS Registry, and GWTG Resuscitation | Quality measures for heart failure |
ACC/AHA/SCAI/AMA-Convened PCPI/NCQA 2013 Performance Measures for Adults Undergoing Percutaneous Coronary Intervention | Quality measures for adults undergoing percutaneous coronary intervention |
The Society of Thoracic Surgeons—Quality Performance Measures www.sts.org/quality-research-patient-safety/quality/quality-performance-measures | Quality measures for thoracic surgery |
AHA, American Heart Association; GWTG, Get With the Guidelines; SCAI, Society for Cardiovascular Angiography and Interventions.
METHODS FOR OUTCOME MEASUREMENT
There are several methods that can be used for outcome measurement in advanced practice nursing using research, EBP, or QI frameworks. The APRN can use any of these methods to evaluate the impact of a new intervention on patient, system, and fiscal outcomes. Each of these methods has its advantages and disadvantages. No single set of research designs or methods is uniquely appropriate for outcome studies. The design and methods used will depend on the state of knowledge about a particular phenomenon and the resources available to the investigator. The APRN will choose among these 101methods depending on the variables under study, the outcome measures under study, the instruments used for measurements, and the feasibility in terms of time and resources (Gawlinski et al., 2013).
This section first reviews the most common research designs used by APRNs for outcome measurement projects (Figure 6.1) and discusses the strengths and weaknesses of each of these methods (Table 6.3). A discussion follows that describes other methods for outcome measurement, such as the research utilization process, EBP models, and QI frameworks.
Designs
Randomized Controlled Trials
The “gold standard” for evaluating the efficacy of drugs and other treatments and interventions in health care is the randomized controlled trial (RCT), or true experiment. RCTs must meet the following three criteria: (a) random assignment to the treatment or control group, (b) manipulation of the independent variable (treatment), and (c) a control or comparison group (Polit & Beck, 2017d). RCTs are concerned with efficacy, that is, with the question of whether a treatment works under ideal conditions. Because efficacy is most easily determined in a homogeneous study sample, RCTs typically have strict inclusion and exclusion criteria and are conducted under highly controlled conditions (O’Mathúna & Fineout-Overholt, 2015; Stevens, 2015). However, the same homogeneity that allows the researcher to determine the impact of the intervention in a well-defined population also limits the extent to which the findings of an RCT apply to the diverse patients usually seen in clinical practice (O’Mathúna & Fineout-Overholt, 2015; Stevens, 2015). Thus, these studies have strong internal validity (attribution of causality), but weak external validity (generalizability).
FIGURE 6.1 Research designs used by advanced practice registered nurses for outcome measurement projects.
RCT, randomized controlled trial.
102TABLE 6.3 Research Designs: Advantages and Disadvantages
Design and Description | Advantage | Disadvantage |
Randomized controlled trial (RCT) Requires: Randomization Manipulation of independent variable Control group Aim: Determine causation | Strong internal validity (confidence that effect was caused by manipulated variable) Most powerful design to test cause-and-effect relationships Provides strong evidence of a cause-and-effect relationship Confounding variables controlled for by randomization Clear measure of efficacy of intervention | Poor external validity (generalizability) Can study only a limited number of variables Complicated and time-consuming Expensive Difficult to maintain integrity of intervention unless done by the research team Ethical and/or logistical issues with randomization May not be able to manipulate variables of interest Does not measure effectiveness of intervention in clinical practice |
Quasi-experimental Lacks either: Randomization or control group Aim: Determine causation | More amenable to real-life clinical situations Practical, inexpensive, feasible Strong external validity (generalizability) Confounding variables may be able to be controlled by statistical means | Weaker internal validity (less causal attribution; i.e., less confidence that outcome was caused by manipulated variable) Confounding variables may be unknown or unable to be controlled for If there is no control group, alternative explanations cannot be ruled out |
Descriptive Complete picture of current state Aim: Description | Simple Relatively unlimited number of variables | Does not address relationships between or among variables High risk of confounding and bias |
Correlation Examine relationship(s) between and among variables Aim: Comparison/association | Test relationships between and among variables Can collect a large amount of data Can predict, as well as describe, association(s) Relatively simple Can study the relationship between many variables | Unable to determine cause–effect relationship Due to complex interactions of patient characteristics, tendency for variables to be related to one another (e.g., anxiety, coping, compliance) |
Case–control “Case” has outcome of interest, “control” does not Aim: Retrospective comparison over time | Can use with relatively small sample sizes Time saving because design is retrospective: Research begins with outcome and searches for antecedent variable (“cause”), which has already occurred | May be difficult to select an appropriate control group Confounding variables may be unknown Limited to one outcome Confounding variables and bias are a concern Need to demonstrate comparability between cases and controls |
Cohort Follow groups of subjects over time—exposure of interest Aim: Prospective comparison over time | Can assess several outcomes Temporal sequence is known Can be used to evaluate effectiveness in other populations of interventions found to be efficacious in RCT Stronger than retrospective comparison | Requires large sample size More resources needed to follow up subjects over time, especially if lengthy follow-up Results may not be identified for years Danger of attrition/dropout of subjects Confounding variables may be unknown |
Ex post facto Evaluate effect of a naturally occurring event on subsequent outcome Aim: Identify possible causation | More realistic setting Can be used where a more rigorous experimental approach is not possible Can be a valuable exploratory tool to identify possible cause-and-effect relationships for further study May be able to control for some antecedent variables with statistical procedures | May be unable to identify all relevant variables, unknown if the causative factor has been identified Poor internal validity if unknown or unidentified variables provide an alternative explanation of relationship The antecedent variable may be a combination of multiple factors Outcome may result from different antecedents on different occasions |
103Qualitative Develop concepts and themes Use words to explain phenomena Natural setting and context Inductive process (use specific data to elaborate theory and concepts) Aim: Explanation/understanding | Used when phenomena are poorly understood Requires a small number of subjects compared with quantitative studies Richness of understanding lived experiences Can complement quantitative research Used to explore complex phenomena | Unable to determine causality or comparison Requires extensive training Time-consuming |
Despite their significant strengths, RCTs also have important limitations. They are typically expensive, time-consuming, and designed to answer a single research question or test a small number of hypotheses. Additionally, the intensive follow-up necessary for most study protocols often bears little resemblance to follow-up patterns in usual clinical practice (O’Mathúna & Fineout-Overholt, 2015; Stevens, 2015). Finally, the importance of clinical significance versus statistical significance cannot be overlooked. Results that do not reach statistical significance do not exclude the possibility of a clinically important relationship (Gaskin & Happell, 2014; Polit & Yang, 2015). Thus, although rigorous RCTs establish the efficacy of a specific therapy in a limited patient population receiving close follow-up, they often leave remaining questions about the impact of therapy in populations not resembling those enrolled in the trial.
Quasi-Experimental
In some situations, a true experimental design is not feasible because of ethical or logistical constraints. In such circumstances, nonrandomized quasi-experimental studies are often the most appropriate design with which to test cause-and-effect relationships (Polit & Beck, 2017d). Quasi-experimental designs are differentiated from experimental designs by lack of random assignment and/or lack of a control or comparison group. However, they are similar to an experiment in that a variable is manipulated and comparison is made to a group not receiving the intervention.
Quasi-experimental designs do not have the rigor created by random assignment and control groups for comparison; rather, comparisons are made with nonequivalent groups or with periodic measurement of the same group (Polit & Beck, 2017d). Quasi-experimental designs are at risk for threats to internal validity, that is, factors that provide an alternative explanation for associations between the independent and dependent variables, and therefore have limited ability to make cause-and-effect statements (Polit & 104Beck, 2017d). Attributing causation is strengthened if the researcher is able to account for alternative explanations of intervention–outcome relationships.
Many nursing outcome studies use a pretest/intervention/posttest method. These research designs can collect data from the same subject over time (within subjects), or from different participant groups (between subjects) at the same or different times. A within-subject design, also known as a repeated-measure design, tests the same subject at two or more time points. In between-subject designs, each participant participates in one and only one group.
Nonexperimental/Observational
Many studies measuring health care outcomes are observational in nature, in contrast to studies that recruit patients in a controlled fashion, as with RCTs. The advantage of an observational method is that patients’ circumstances and outcomes of interventions are studied in a heterogeneous population, which better reflects actual practice environments. The obvious disadvantage is that without the controls imposed by an experimental study, observational results are subject to error and can be misleading or misinterpreted (Polit & Beck, 2017d). Threats to validity, such as bias, or confounding or random error, can provide an alternative explanation other than the effect of the independent variable for changes in the dependent variable after intervention.
Bias is any factor or influence that produces a systematic distortion or error in the study results (Polit & Beck, 2017e). Selection bias is especially problematic in nonrandomized studies; that is, such studies have the concern that subjects who elect to participate are systematically different from those who decline participation. Two main factors that can contribute to selection bias are self-selection, when the sample selects itself, and convenience sampling, when individuals are selected because they are easy to obtain (Polit & Beck, 2017e). For example, in a study of caregivers of terminally ill patients, the researcher may choose a sample of subjects who responded to a recruitment flyer (self-selection) or a sample of caregivers who attend a support group (convenience sample). Either sample is probably not representative of the total population of caregivers of terminally ill patients.
Confounding variables are variables that are associated with both the independent and the dependent variable in such a way that the relationship between the intervention and outcome is actually due to a third (known or unknown) variable, and the nature of the association between the independent and dependent variable is misrepresented (O’Mathúna & Fineout-Overholt, 2015). The effect of a confounding variable can offer an alternative explanation for a relationship between an intervention and an outcome (Carson, 2010). For example, researchers may find an association between consuming a vegetarian diet and lower BMI and draw the conclusion that the diet is the cause of lower BMI. However, this association may be confounded by the fact that vegetarians are perhaps more health conscious in general and exercise more regularly. Thus, exercise may be the true cause of the difference in BMI. The use of multivariate statistical models such as linear or logistic regression may be able to “adjust” for confounding variables in some situations (Carson, 2010).
Random error is essentially nonsystematic bias; that is, the factor or influence that produces error is as likely to influence results in one way as another (Polit & Beck, 2017e). Random error is due to variability in the data that occurs purely by chance. Because of 105random error, one cannot unequivocally state that the results obtained in a study are real rather than arising by chance. The probability that the outcome variable has occurred purely by chance is expressed as the p value. The most effective way of minimizing random error in a study is to increase the sample size (Polit & Beck, 2017e). The classic example of correcting random error is the coin toss. When tossing a coin, we expect heads to occur 50% of the time. If the coin were tossed 10 times, it is likely that the number of heads–tails would not be 5−5, but may be for example 6−4 or 3−7. However, if the coin were tossed 1,000 times, we would be more likely to approach 50−50 (or actually 500–500) on heads coming up.
Descriptive
The descriptive research design is the most basic. It simply describes the nature of the phenomenon of interest. Data are gathered for descriptive research in three ways: observation of actions, appraisal of records or documents, and results of surveys specifically designed to measure variables of interest. Descriptive research involves observing, describing, and documenting events or phenomena and then organizing, tabulating, depicting, and describing the data elements. Descriptive studies also report summary data such as measures of central tendency (mean, median, mode) and measures of dispersion (range and standard deviation). These designs describe what actually exists, determine the frequency with which it occurs, and categorize the information (Polit & Beck, 2017d). Descriptive statistical techniques allow very large amounts of data to be made meaningful to the researcher.
Descriptive data are the foundation of any quantitative study and can serve as a foundation for theory generation or hypothesis testing in a quantitative study (Polit & Beck, 2017d). The fact that descriptive studies are “simple” and one cannot infer causality from them does not diminish the value of the descriptive study design. An example of an important, purely descriptive research is the decennial U.S. Census, the purpose of which is to accurately and precisely describe the population of the entire country.
Correlation
Correlation determines whether, and to what degree, a relationship exists between two or more variables. This design is used to determine if changes in one or more variables are related to changes in another variable (Melnyk, Morrison-Beedy, & Cole, 2015). Correlation can tell you only that two or more phenomena are related, not that a causal relationship exists. The degree of the relationship between variables is expressed as a correlation coefficient. The correlation coefficient indicates the strength of relationship (strong, weak, or none) as well as the direction of the relationship, that is, positive, in which variables move in the same direction, or negative, in which variables move in opposite directions. Correlation coefficients range in value from –1.0 (strongly negative) to +1.0 (strongly positive), with 0 indicating no relationship.
Correlation designs can be either descriptive or predictive. Descriptive correlation simply describes the variables under study and the relationship(s) between and among them. Predictive correlation predicts the amount of variance in one or more variables based on the amount of variance in other variable(s), given that the predictor variable occurs earlier in time (Melnyk et al., 2015).
106 Case–Control and Cohort
In the case–control study design, “cases” with a disease or condition are identified within a population of patients, and investigators go back a period of time before onset of the disease or condition and assess the presence of potential risk factors or other influences preceding the disease or condition (Melnyk et al., 2015). For example, K. D. Burns et al. (2009) conducted a case–control study to identify risk factors for delirium in patients undergoing elective heart surgery (K. D. Burns et al., 2009). The “cases” were the 11 patients (29%) who had developed postoperative delirium and the “controls” were the 27 patients (71%) who did not. Numerous preoperative, intraoperative, and postoperative variables were included in the analysis to determine risk factors for delirium. The factors most strongly associated with delirium were recent alcohol use, intubation time, time in the intensive care unit (ICU), and postoperative creatinine levels (K. D. Burns et al., 2009).
In the cohort study design, the presence of risk or predictor variables is measured at baseline within a selected population. Investigators then follow subjects over time to determine which patients have the disease or developed the condition of interest. Typically, cohort studies are prospective in nature, that is, subjects are followed forward in time (Melnyk et al., 2015). Perhaps the most famous cohort study in the United States is the Framingham Heart Study, begun in 1947 by the U.S. Public Health Service, and continued under the auspices of the National Heart, Lung, and Blood Institute (NHLBI) of the National Institutes of Health (NIH). The study initially enrolled a random sample of 5,209 subjects, aged 30 to 59 in the town of Framingham, Massachusetts (Dawber, Meadors, & Moore, 1951). Since 1948, the subjects have continued to return to the study every 2 years for a detailed medical history, physical examination, and laboratory tests. In 1971, the study enrolled a second generation, 5,124 of the original participants’ adult children and their spouses, to participate in similar examinations. In 2002, enrollment of a third generation of participants was begun. Since 1951, investigators have published 2,346 studies based on Framingham Heart Study data in peer-reviewed medical journals.
Ex Post Facto
Literally translated, ex post facto means “from what is done after.” In ex post facto studies, the researcher retrospectively examines the effects of a naturally occurring event on a subsequent outcome for the purpose of establishing a causal link between them. Ex post facto studies are similar to case–control studies in that they begin by observing an existing condition or state of affairs. Subsequently, the researcher searches back in time for plausible causes, relationships, or associations between the variables (Giuffre, 1997). The study begins with preexisting groups that are already different in some respect(s) and searches in the past for the factor(s) that brought about the differences (Cohen, Manion, & Morrison, 2000).
By convention, the antecedent variable is often referred to as the “independent variable” or “cause,” and the outcome variable is also called the “dependent variable” or “effect” (this despite the fact that ex post facto designs can only weakly predict cause and effect). For the purposes of clarity, the authors use the terms “antecedent” and “outcome” variable.
107Ex post facto designs are used when the researcher is interested in cause-and-effect relationships, but it is not possible to manipulate variables (i.e., to conduct an experimental study). Results of these ex post facto studies can provide a beginning sense of cause-and-effect relationships that can subsequently be tested with more rigorous research methods. It is an inexpensive study design in that the phenomena of interest have already occurred and no interventions are performed.
One of the major challenges for researchers in using an ex post facto design is to identify preexisting groups that are comparable to a randomly assigned group, that is, the challenge is to ensure that the only difference in the groups is the presence or absence of the outcome or “effect” variable (Giuffre, 1997). One of the most common means of achieving this comparability is to match the subjects in the “effect” and “no effect” groups on variables known or believed to influence the outcome variable. The difficulty with this strategy is that it assumes that the investigator knows what all the relevant factors are that may be related to the outcome variable. Similarly, factors that could influence the antecedent variable must be accounted for; again, there is the assumption that the investigator can identify all the relevant factors (Cohen et al., 2000).
Finally, the researcher must recognize that no single antecedent variable may be the “cause” of the outcome. Many antecedent variables may be interrelated or the result of more than one variable interacting with others. Likewise, a particular outcome may result from different antecedents on different occasions.
Qualitative
Qualitative studies describe human responses in a particular situation and context and the meaning that human beings bring to the situation (Powers, 2015a). Qualitative studies are often exploratory in nature and seek to generate new insight or understandings, using inductive (starting with data and developing hypotheses) rather than deductive (starting with hypotheses and testing them with data) approaches (Powers, 2015a).
The three most often used qualitative designs are ethnography, phenomenology, and grounded theory (Powers, 2015a). Ethnography is the direct description of a group, culture, or community—the culture as experienced by its members. Although ethnographic methods originated in cultural anthropology, they can also be used to describe the culture of a health care organization or patient care unit. Phenomenology uses in-depth interviews and open-ended discussion to describe and interpret the lived experience or phenomenon under study and to derive a depth of understanding and meaning from the experiences of participants.
Grounded theory uses data points (verbatim quotations) to generate or modify a theory. Data are collected from in-depth interviews and open-ended discussion to understand processes and behaviors as individuals move through experiences over time. The purpose of this design is to generate a theory that is “grounded” in real-life empirical data (Corbin & Strauss, 2008). The developing theory evolves during data collection and analysis as a part of the research process and the sample of participants is constantly developed to explore insights that emerge from the data (Corbin & Strauss, 2008).
The application of grounded theory is illustrated in a landmark study (Conrad, 1985) using data from 80 in-depth interviews of people with epilepsy. The study analyzed the 108meaning of medications in the lives of the subjects to determine why people did or did not take their medications. From the interview data, it was found that, from the patients’ perspective, the issue was more of controlling dependence, and the practicalities of frequent medication administration. Conrad developed the theory of self-regulation from his belief that what appeared to be noncompliance (from a medical perspective) was actually a form of asserting control over a disorder.
Qualitative research designs are often used in areas in which data or knowledge is inadequate or in which conventional theories may not be applicable. Qualitative methods can be used to understand complex social processes to capture essential aspects of a phenomenon from the perspective of study participants, and to uncover beliefs, values, and motivations that underlie individual health behaviors (Powers, 2015b).
Qualitative research can be distinguished from quantitative research in several ways. First, quantitative research uses numbers (e.g., frequency or magnitude of variables) to describe phenomena, whereas qualitative research uses words to describe and understand the complexity and depth of phenomena or experiences. Second, quantitative research tests hypotheses with statistical methods to determine statistical significance, whereas qualitative research seeks to generate hypotheses about a phenomenon, its antecedents, and its aftermath. Third, quantitative research is performed primarily in experimental settings and generates numeric data using valid and reliable instruments to measure predetermined variables. In contrast, qualitative research occurs in natural settings and produces word-based data through open-ended discussions, in-depth interviews, focus groups, observation, and document review (Powers, 2015b).
Mixed Methods
Mixed methods, in which quantitative and qualitative research designs are combined, are increasingly recognized as appropriate and important in health care research because they capitalize on the respective strengths of each approach (Curry, Nemhard, & Bradley, 2009). The NIH published a useful resource in their report “Best Practices for Mixed Methods Research in the Health Sciences” (Creswell, Klassen, Plano Clark, Smith, & the Office of Behavioral and Social Sciences Research, 2011). The report provides practical recommendations for researchers seeking to incorporate mixed-methods research into their applications for NIH “R” series research grants as well as fellowship, career, training, and center grants.
Qualitative studies can generate theories and identify relevant variables to be studied subsequently in quantitative studies. They can also be used in a complementary fashion to yield findings that are broader in scope and richer in meaning than those derived from quantitative data alone (Powers, 2015b).
In the seminal work by Greenhalgh (2002), three ways are described in which qualitative research designs interact with quantitative methods: exploratory, explanatory, and evaluative. First, an exploratory process can be used to generate hypotheses and often serves as the first step in a sequence of research that includes a quantitative stage. Second, qualitative data can be used in an explanatory fashion to further inform relationships between or among variables in a quantitative study. Although a quantitative study could answer the question of whether an intervention is efficacious, a qualitative study could answer the question of why the intervention was successful. Finally, after a quantitative 109study has demonstrated the efficacy of a particular intervention, qualitative data can be used to evaluate why this evidence may not become incorporated into practice or may be incompletely incorporated.
Approaches to mixed-methods studies can vary based on the sequence of studies and the relative emphasis placed on each approach. The qualitative and quantitative elements may be performed concurrently or sequentially and, depending on the phenomena under investigation, one approach or the other may dominate the study design. For example, if a quantitative study yields unexpected or conflicting results, a qualitative follow-up study may help to ascertain important relationships that inform interpretation of the results. Likewise, qualitative studies may be performed to generate hypotheses or characterize a phenomenon about which little is known, which are then further studied using one or more quantitative methods. Strategies to enhance the validity of mixed-methods studies include recognizing the role of each strategy and adhering to the methodological assumptions of each design (Creswell et al., 2011).
Methods
Research Utilization and EBP
APRNs may opt to use existing research to make a practice change and evaluate the effects of implementing a new innovation on specific outcomes. This type of project would use a research utilization approach or framework. Research utilization refers to the “process by which specific research-based knowledge (science) is implemented in practice” (Estabrooks, Walling, & Milner, 2003, p. 5). A disadvantage to using strictly research utilization methods is that traditional researchers use inclusion and exclusion criteria that restrict the subjects to a homogeneous sample, in order to decrease possible biases and variance and to increase the probability of identifying a statistically significant difference (N. Burns & Grove, 2015). Application of research findings to a population other than that studied may be problematic. One of the important steps in research utilization is to critique and synthesize research findings to determine relevance and feasibility in the APRN’s particular practice setting (Stetler, 2001). Increasingly, research utilization is being integrated into the larger concept of EBP.
EBP represents a broader concept than research utilization. When clinicians use the EBP approach, they can incorporate additional levels of evidence and consider the nurse’s clinical expertise as well as the patient’s preferences and values. Sackett et al. originally defined EBP as “the conscientious, explicit and judicious use of current best evidence in making decisions about the care of the individual patient. It means integrating individual clinical expertise with the best available external clinical evidence from systematic research” (Sackett, Rosenberg, Gray, Haynes, & Richardson, 1996, p. 71). Consideration of the individual patient’s values and preferences was considered as part of the health care provider’s clinical expertise (Sackett, Richardson, Rosenberg, & Haynes, 2000). Ingersoll (2000) proposed that EBP should also consider the importance of using theoretical foundations in the evidence-based decision-making process.
There are a number of models for implementing an EBP method for outcome research. Common elements of all EBP models include (a) identifying a clinical problem, (b) gathering evidence, (c) critiquing and synthesizing evidence, (d) implementing practice change, and (e) evaluating the impact of practice change on outcomes. These models can assist 110nurses to systematically approach clinical practice problems and proceed toward actual implementation in a specific practice setting. Use of an EBP model can prevent incomplete or unsuccessful implementations of the practice change, promote timely evaluation, and maximize use of time and resources (Gawlinski & Rutledge, 2008). EBP models that have specific stages or phases that can guide the APRN in an outcome measurement project are described in Table 6.4. EBP models that do not have specific stages or phases, but help describe and conceptualize the many variables and interactions that can occur when making an EBP change, are described in Table 6.5.
Quality Improvement
Finally, a QI process can be chosen to guide an outcome measurement project. The QI method involves systematic processes of data collection and inquiry and refers to activities that use data-based methods to bring about rapid improvements in health care delivery (McLaughlin & Kaluzny, 2013). A basic premise of QI is that measures of good performance reflect good-quality practice and that comparing performance among providers and organizations will encourage better performance (McLaughlin & Kaluzny, 2013).
The QI process includes developing indicators to assess progress toward certain predefined goals and reviewing performance against these measures. Data for QI investigation are derived internally and usually reported only internally. QI evaluates work processes in a cyclic fashion, benchmarks practice against established indicators, and provides a means to continually evaluate and improve established practice (McLaughlin & Kaluzny, 2013).
TABLE 6.4 Selected Evidence-Based Practice Nursing Models and Their Key Components
111TABLE 6.5 Select Evidence-Based Practice Frameworks
| ARCC Model | PARIHS Framework |
Key focus | Organization of department or unit | Understanding key components of EBP |
Key concepts | EBP mentor—an individual who has proficient knowledge and skills in EBP and the passion to help others practice daily from an evidence-based care | Evidence Context Facilitation |
Major proposition | The development of APRNs and other nurses as EBP mentors facilitates an organizational culture change toward evidence-based care | Practice changes are most likely when based upon robust evidence, conducted in a context “friendly” to change, and facilitated well |
Utility—practical implications | Need to . . . Assess and organize culture and readiness for EBP Identify strengths and major barriers to EBP implementation Implement ARCC strategies Develop and use of EBP mentors Interactive EBP skill-building workshop EBP rounds and journal clubs EBP implementation Improve patient, nurse, system outcomes | Need to . . . Critically appraise evidence Thoroughly understand the practice arena before implementing a change Make a strategic plan for facilitation of any practice change—from development through implementation and evaluation |
ARCC, Advancing Research and Clinical Practice through Close Collaboration; EBP, evidence-based practice; PARIHS, Promoting Action on Research Implementation in Health Services.
Source: Adapted from Gawlinski and Rutledge (2008).
QI methods enable organizations to make change in a systematic way, measuring and assessing the effects of a change, feeding the information back into the clinical setting, and making adjustments until they are satisfied with the results (McLaughlin & Kaluzny, 2013). The APRN is in an ideal position to implement and participate in QI activities through the roles of practitioner, teacher, researcher, and consultant. A thorough knowledge of the institution’s QI method as well as skillful implementation of the QI process is required for the APRN to monitor unit-based quality indicators of care, the performance of practitioners, and the overall quality of care provided (Altmiller, 2011).
No one “right” QI method can be applied that will be effective in all organizations. Individual organizations have their own networks, structures, organizational histories, and challenges, which need to be considered in relation to the choice and implementation of QI methods. The specific approach (or combination of approaches) may be less important than the thoughtful consideration of the match and “best fit” for the particular circumstances (Seidl & Newhouse, 2012). A number of QI methods have been adapted to the health care setting (Nicolay et al., 2012). A brief description of these methods is provided in Table 6.6.
112TABLE 6.6 Quality Improvement Models
Model | Description |
Donabedian’s Model of Quality Health Care Donabedian was one of the founders of quality improvement, and the model continues to serve as a unifying conceptual framework for quality improvement All steps are vital: process improvement is limited and temporary, when the structure is not also improved. | Structure: Availability, accessibility, and quality of resources Process: Delivery of health care services by clinicians Outcome: Final results of health care |
Total Quality Management and Continuous Quality Improvement (Deming) Developed in Japan in the 1950s to rebuild and improve their manufacturing industry Terms are now also used to describe more general approaches to quality improvement Important concepts include: Continuous performance evaluation Involves management at all levels Quality is the responsibility of everyone Quality improvement is data driven | Process management: Focus on process and systems to improve, rather than individuals Problem solving: Use of structured approaches based on statistical analysis; data is a key tool Leadership: Management involvement at all levels Employee empowerment: Use of teams to identify problems and opportunities and to take the necessary action |
PDCA (plan–do–check–act) Also known as rapid-cycle QI; promoted by the Institute for Healthcare Improvement (IHI) Repeated short-cycle small-scale changes Begin with easiest changes, repeat cycles to address more complex processes Cyclic and iterative | Plan: A change in process to improve quality Do: Implement the change with a strategic plan for implementation Check: Evaluate and analyze results Act: Adopt, adapt, or abandon |
Six Sigma Developed by Motorola in the 1980s for QI Sigma is the statistical term for variance; 1 Sigma is one standard deviation, 6 Sigma is 6 standard deviations, which represents 99.99966% of possible events Goal is 3.4 failures per million “opportunities” Also cyclic and iterative | Define: Goal of improvement and key people involved Measure: Current system; may be challenging if data are not available for the processes of interest Analyze: Ways to close gap Improve: Implement and evaluate Control: Plans and process to maintain |
Toyota Lean Developed by Toyota in 1940s Basic principle is to minimize waste, both in product and processes Focuses on value to the customer | Value stream map identifies exact steps of the work process and the value associated with the step Processes are aligned for continuous flow of work Create an environment of constant review Extensive use of visual cues to streamline processes and prevent error |
Root Cause Analysis Began as a way to evaluate industrial accidents Systematic retrospective analysis to identify and understand the underlying cause of process failures Extensive use of tools and diagrams of process requires extensive training | Focus on systems and processes Goal is to understand contributory factors that create an environment where errors can happen Required by The Joint Commission (TJC) and other regulatory agencies for all sentinel events Extensive use of tools and diagrams of process requires extensive training |
Failure Modes and Effects Analysis (FMEA) Prospective risk assessment tool developed by the military and National Aeronautics and Space Administration (NASA) to evaluate potential failures and unrecognized hazards. Can be used to evaluate both designs and processes | Identify the system to be analyzed and the individual processes within the system that are the most problematic or high risk Create a process map (diagram) to describe each individual step in the process Identify all potential failures in a process |
113Goal is to identify process failures that would be the most significant and design preventive or mitigating measures before failure occurs Flowcharts and brainstorming are useful tools | Define and anticipate effect of each failure, cause, degree of seriousness, and possible solutions Calculate a risk priority score for each potential failure System or process redesign Reanalysis |
QI, quality improvement.
ROLE OF THE APRN IN OUTCOME MEASUREMENT
The role of the APRN is to integrate education, research, management, leadership, and consultation into his or her clinical practice, making the APRN well-suited to manage the complexities of HF patients and their evolving care needs. The following section describes an evidence-based outcome measurement project initiated by the APRNs employed in the Ahmanson University of California Los Angeles (UCLA) Cardiomyopathy Center, for the purpose of improving patient education and decreasing medication discrepancies during the high-risk transition from hospital to home. Through a detailed plan of interventions, the APRNs created a new process across the continuum from hospitalization to home. APRNs providing in-hospital patient education, facilitating and participating in the patient discharge process, and providing phone monitoring through to the outpatient follow-up clinic visit have resulted in a decrease in medication discrepancies after hospital discharge.
Background
HF continues to be a major public problem resulting in substantial morbidity and mortality. A rapidly increasing aging population coupled with the advancement of life-prolonging interventions has led to increasing rates of HF. HF prevalence increases with age, its incidence accelerates from 20 per 1,000 individuals 65 to 69 years of age to more than 80 per 1,000 individuals older than 85 years of age (Curtis et al., 2008; Yancy et al., 2013; American College of Cardiology Foundation [ACCF]/AHA HF guideline). A 2016 update from the AHA estimated that 5.7 million people older than 20 years of age in the United States have HF, with an incidence of 915,000 new cases diagnosed annually (Atherosclerosis Risk in Communities Study [ARIC] of the NHLBI, 2005–2012; Chang et al., 2014; Mozaffarian et al., 2016). Projections indicate that by 2030, more than 8 million people older than 18 years of age will have HF, a 46% increase in prevalence from 2012 (Heidenreich et al., 2013; Mozaffarian et al., 2016). Although survival after HF diagnosis has improved over time, the mortality rate remains unacceptably high. One in nine deaths in 2009 included HF as a contributing cause (National Center for Health Statistics, 2013; NHLBI, 2013) and approximately 7% of all cardiovascular deaths are due to HF (Go et al., 2013). Among Medicare beneficiaries, the overall 1-year HF mortality has remained high at 29.6% (Chen, Normand, Wang, & Krumholz, 2011; National Center for Health Statistics, 2011), with continued estimates that 50% of people with a diagnosis of HF will die within 5 years of diagnosis. Additionally, in the ARIC study, 30-day, 1-year, and 5-year case fatality rates after hospitalization for HF were 10.4%, 22%, and 42.3%, respectively 114(Chang et al., 2014; Loehr, Rosamond, Chang, Folsom, & Chambers, 2008; Yancy et al., 2013 [ACCF/AHA HF guideline]).
HF also accounts for a large burden in rising health care expenditures, translating into 5 million office visits (National Hospital Ambulatory Medical Care Survey Data, 2009; Voigt et al., 2014), 553,000 ED visits, and 1.1 million hospitalizations per year (Roger et al., 2012). It is the most common diagnosis-related-group discharge in persons older than 65 years (Roger et al., 2012). The indirect and direct costs of HF treatment in the United States now exceed $30 billion annually, a sobering statistic, driving 5.4% of all health care costs (Go et al., 2013). The lifetime burden of hospitalizations in persons with a diagnosis of HF has been reported as 83% of patients hospitalized at least once and 43% of patients hospitalized at least four times (Dunlay et al., 2009). Although physician office visits accounted for $1.8 billion, more than half of the total costs for HF was spent on hospitalizations. Go et al. (2013) estimated the mean cost of an HF-related admission at $23,077 per patient (Go et al., 2013). With a mean 4.7-day length of stay, hospitalization is the predominant contributor to HF costs and is the largest single expense for Medicare. Unfortunately, nearly 25% of HF patients are readmitted within 30 days of discharge (Dharmarajan et al., 2013).
Quality and accountability of practitioners and organizations have become paramount in medical practice particularly in relation to the potential impact for the HF population. Performance measures for HF include process measures and outcome measures. The CMS, TJC, Hospital Quality Alliance, Agency for Health Research and Quality (AHRQ), and ACCF/AHA/Physician Consortium for Performance Improvement (PCPI) define quality metrics and performance measures for patient care and HF management. Process performance measures focus on the aspects of care delivered to a patient and are based on professionally developed and definitive clinical practice guideline recommendations. Performance measures are suitable for use for accountability whereas quality metrics are suitable for QI (Yancy et al., 2013). Medication safety, patient counseling and education, implementation of evidence-based HF guideline management, and adequate postdischarge follow-up are critical elements defined by these organizations. “Hospital Compare,” created through the efforts of the CMS, the Department of Health and Human Services (DHS), and the Hospital Quality Alliance: Improving Care through Information initiative, provides objective and verifiable data regarding HF outcomes. CMS data identified HF as the most frequent diagnosis-related group with the highest readmission rate of any other common medical or surgical condition in Medicare beneficiaries. Thus, HF admission and mortality rates, and in particular, 30-day rehospitalization rates and 30-day mortality rates for HF are now key national outcome measures. These performance measures are now incorporated into the CMS VBP program. With an intended effort to have hospitals pay close attention to HF patients after discharge, the Patient Protection Affordable Care Act of 2010 created new incentives to reduce readmissions. Hospitals with high readmission rates would be penalized as much as 3% of their Medicare reimbursement by 2015 and beyond (Bradley et al., 2013). The VBP program focuses on a hospital’s performance on 25 quality measures related to clinical process of care measures and patients’ care experience. Patient satisfaction determines 30% of incentive payments while improved clinical outcomes generate 70% (CMS, 2011).
Because of the high mortality and tremendous costs associated with HF treatment, adherence to evidence-based therapy is critical. Literature supports the benefits of 115evidence-based guideline medical therapy and lifestyle modification in delaying disease progression and improving survival in patients with HF (Calvin et al., 2012; Fonarow et al., 2010). However, patients’ adherence to these treatment regimens shows high variability, with rates ranging from 10% to as high as 85% (Calvin et al., 2012; Fonarow et al., 2010; Foust, Naylor, Bixby, & Ratcliffe, 2012; Yancy et al., 2013). The most common factors associated with patient nonadherence to HF treatment recommendations and rehospitalization include complicated medical regimens, inappropriate medication reconciliation, poor discharge instructions, lack of patient understanding, lack of health literacy, poor communication among health care providers between sites of care, lack of a plan for appropriate medical follow-up after discharge, lower socioeconomic status, minority status, psychosocial variables, and age (Calvin et al., 2012; Fonarow et al., 2010; Foust et al., 2012; Wiggins, Rodgers, DiDomenico, Cook, & Page, 2013; Yancy et al., 2013). A significant proportion of hospital readmissions are caused by medication-related adverse events. An estimated 19% of discharged patients experienced an adverse event after discharge, of which two thirds were attributed to medications. Additionally, lack of adherence to medications prescribed at discharge is a driver of adverse drug events after discharge (Bristol Calvert et al., 2012; Hubbard & McNeil, 2012). Consequently, medication management is at the core of advanced discharge planning and transitional care for avoidable hospital readmissions.
Clinical Issue
Maximizing evidence-based HF guideline therapy is part of inpatient and outpatient HF management. Despite written hospital discharge instructions and prescriptions provided by the hospital’s cardiac care unit service, a large number of patients had a significant knowledge deficit regarding what medications, purpose or indication, dose or frequency they were prescribed when interviewed at their 1-week hospital follow-up HF clinic visit. The patients and/or care providers were often confused about their medication regimens and changes made while in the hospital. Medication discrepancies can be detrimental to the control of HF symptoms for the patient, resulting in clinical HF progression, fluid overload exacerbation, hypertension, hypotension, hyperkalemia, hypokalemia, renal insufficiency, or liver dysfunction. It became apparent to the cardiomyopathy APRNs that there was a need to bridge the education gap at the transition from hospital discharge to home. The APRNs found that cardioprotective medications initiated, uptitrated, or changed in the hospital were often not continued after discharge. In an effort to improve the discharge transition to home, the APRNs identified the factors contributing to medication discrepancies, which included the following:
New prescriptions not filled because of need for prior insurance authorization, high cost, or inability to go to the pharmacy
Patients experienced side effects and discontinued medicines without consultation with a practitioner
Patients used old prescriptions/medications available at home of the same drug but having a dose or strength different from what was prescribed
Patients expressed that discharge paperwork was overwhelming and/or confusing
116 Patients were uncertain whom to contact regarding medication questions (especially if discharged weekend/after hours)
Elderly patients with functional impairments often had difficulty following and understanding dosing regimen
Patients’ lack of social support to assist with medication management
Patients’ knowledge deficit of the importance of their HF medications
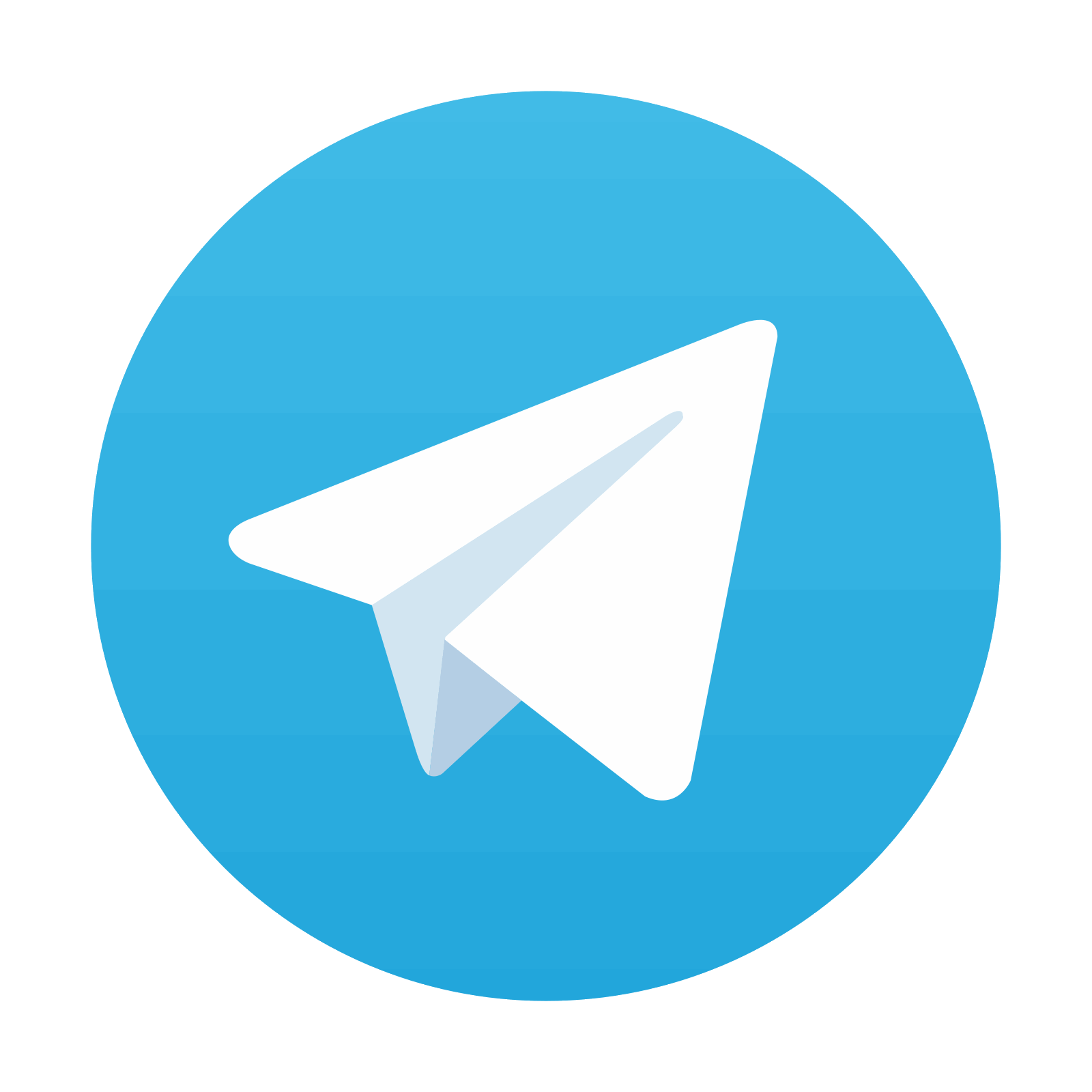
Stay updated, free articles. Join our Telegram channel

Full access? Get Clinical Tree
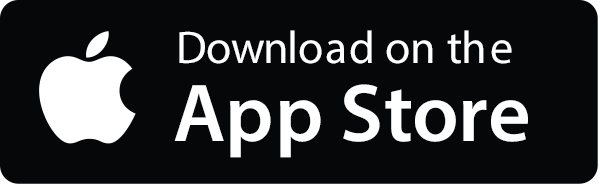
