Chapter 25. Longitudinal Studies
Roger Watson
▪ Introduction
▪ Why do we need longitudinal studies?
▪ Who should be included in longitudinal studies?
▪ Longitudinal studies are not easy
▪ Methodological considerations
▪ Questionnaires
▪ Analysis
▪ Single-case studies
▪ Multivariate analysis
▪ Conclusion
Introduction
Chapter 17was concerned with the ways data can be obtained using a range of non-experimental methods using cross-sectional studies. An extension of the use of survey methods, longitudinal studies, are considered in this chapter. If you wish to know how something changes over time either as a result of an intervention or for no other reason than the passage of time – a prime example being ageing (see, for example, Wenger et al 1999) – then longitudinal studies are required.
Longitudinal studies are characterised, as distinct from cross-sectional studies, by the collection of data at two or more time points (Watson 1998). Two or three time points – although there are exceptions – are common because there are many difficulties associated with gathering data across more time points (McKenna et al 2006). This chapter will consider some of the analytical methods associated with longitudinal studies but will mainly be concerned with addressing the practical aspects of longitudinal studies.
Why do we need longitudinal studies?
It is possible to study ageing, for example, using cross-sectional or longitudinal studies. If we wish to study whether or not age and cardiovascular disease are linked we could take a large sample of people with a wide range of ages at one time. We are very likely to find that there is a link between ageing and cardiovascular disease but we may be limited in the use to which that information could be put. If we wish to make short-term projections about use of health service resources then the data may be useful. However, if we wish to make long-term projections about the link between age and cardiovascular disease the data are less useful. In any such study, the older people who were included have lived through very different times and experiences from the younger people in the study who would, in turn, become the older people of the future. During the lifetime of the older people in the study, many changes influencing cardiovascular disease will have taken place such as nutrition, improvements in health services, awareness of smoking and sedentary lifestyles and these will, possibly, have had more influence on the younger than the older people in the sample. Therefore, it may not be valid to compare young and old people sampled at the same time and this is referred to as a ‘cohort effect’ (Polit & Hungler 1995). The influence of such a cohort effect on any future predictions of the link between age and cardiovascular disease is very hard to predict. For example, it may lead to fewer older people having cardiovascular disease due to better health education and health care. Conversely, it may also lead to more older people with cardiovascular disease in future as improvements in health care increase the survival time of those with cardiovascular disease.
The way to overcome the cohort effect is to carry out a longitudinal study whereby the same group of people is followed over a long time with several data collection points. Cross-sectional studies are still used, however, especially in studies of ageing. They are quick and relatively cheap. If we wanted to study the effect of ageing over a 50-year period, the person initiating the study is unlikely to be alive at its conclusion and few researchers would be willing to commit to this. However, a notable exception is the Baltimore Longitudinal Study on Ageing established in 1958 by Nathan Shock (http://www.grc.nia.nih.gov/branches/blsa/blsa.htm– accessed 30 April 2007). On the other hand, in educational studies, where the progress of students is being followed over a programme there is little excuse for not conducting a longitudinal study, especially when personnel changes, curriculum changes and so forth may induce a profound cohort effect.
Who should be included in longitudinal studies?
Longitudinal studies can include repeated cross-sectional studies where the same data are gathered at subsequent times regardless of who is included at each time. Such studies are favoured by the organisations that test political affiliations. However, such a design is relatively weak because the same people are not necessarily sampled at each time (Watson 1998). Such studies are called trend studies (Polit & Hungler 1995). The purpose of longitudinal studies is to measure change and the weaknesses in repeated cross-sectional studies are obvious and mainly relate to the fact that any change that is observed may simply be due to the fact that different people have been included in the cross-sectional samples. While efforts should be made to ensure that such samples are representative of the population and that bias is minimised, it is impossible to be certain that what is observed is not an artefact of who has been included at each time.
The strongest design in longitudinal studies is one where the same people are included at each time (Watson 1998). These studies are called cohort studies (Parahoo 2006 and Polit 1995). This is especially true where the effect of an intervention is being studied. In such a study, only those who have had the intervention, and possibly a control group, could possibly be of interest and, to be certain that it was the intervention that was being studied, only people studied before the intervention or at the first point in the study should be included (Elliott & Hayes 2003). A baseline for each subject has to be established to provide a mean baseline for the group receiving the intervention and for the control group. The introduction of other subjects later in the study would threaten the validity of the results.
Longitudinal studies are not easy
However, despite the superiority of longitudinal over cross-sectional or even repeated cross-sectional designs, they are inherently difficult to conduct. They are expensive due to their length and it can be difficult to obtain funding; they do not offer a very ‘quick return’ for research funding investment, making them unattractive to some researchers and research funding bodies. However, the primary problem with longitudinal studies is that of attrition(Polit & Hungler 1995): the loss of subjects throughout the study and the concomitant difficulty of finding people who are, initially, willing to enter into a long-term relationship with a research team and, thereafter, willing to remain in the study. Even if people enter a longitudinal study, with the best will in the world, it may be difficult or impossible to find them in subsequent waves of a study – especially if the time between waves is significant (years) and the study is very long. The reasons are obvious: apart from dying or not wishing to remain in the study, people move home during the course of a study. In addition, the research team may change or funding may not be secure for the proposed duration of the study.
Attrition
The problem of attrition is the main consideration in any longitudinal study. Given that it is quite possible to have 50% attrition in a study with relatively short periods between waves and with a population that is not mobile (Watson 1999a and Watson 1999b), then you can imagine the problem in studies with extended periods between waves and including mobile populations. There is no easy solution to this problem. The solution most often applied is to ensure that the initial wave of the study begins with a sample size that is sufficient to withstand attrition over several waves, leaving a sufficient sample size for analysis across all the time waves. Clearly, with no effort to stem attrition between waves, this is very expensive and wasteful. For example, if the aim is to have a sample size of 200 people in the final wave of a study to permit robust statistical analysis and there are four waves in the study, then the initial sample size, if attrition is estimated at 50% between each wave of the study, would have to be 1600 people. If we also take into account that there may only be a 50% return rate of data at any of the waves of the study, then the problem is further compounded and the initial sample size needs to be 3200. This is a very large sample size for any research study and the initial expense will be very high. The likelihood of conducting a study which requires the above numbers will also be dependent on the group that is being studied. For example, if you wish to study the general population, then this could relatively easily be carried out in one city. However, if you wanted to study student nurses, then you would have to include nearly all of the departments of nursing in the UK.
Addressing attrition
It is clear that one of the major problems to be addressed in longitudinal studies is attrition. The most cost-effective way of conducting a longitudinal study is to keep the initial sample size as small as possible and then to maintain a high proportion of that sample for the duration of the study. In addition, the duration of the study should be as long as necessary to gather the data of interest and no longer. For example, if two waves of a longitudinal study will provide you with all the information you need about some phenomenon then a third wave is unnecessary. Several strategies can be applied to maintain a sample in a longitudinal study. However, the key strategy is to ensure the commitment of the people who are entering at the start of the study. In effect, this is no different from ensuring that people participate in any study, whether cross-sectional or longitudinal. However, any negative experiences of participating in the first wave of a longitudinal study will compound any deficiencies in your strategy to get people to participate in the first place. Most of what follows is based on experience of conducting longitudinal studies with nursing students (Watson 1999a and Watson 1999b); the BMJ (http://bmj.bmjjournals.com/epidem/epid.7.html– accessed 30 April 2007) and the Centre for Longitudinal Studies also offer excellent advice (http://www.cls.ioe.ac.uk– accessed 30 April 2007).
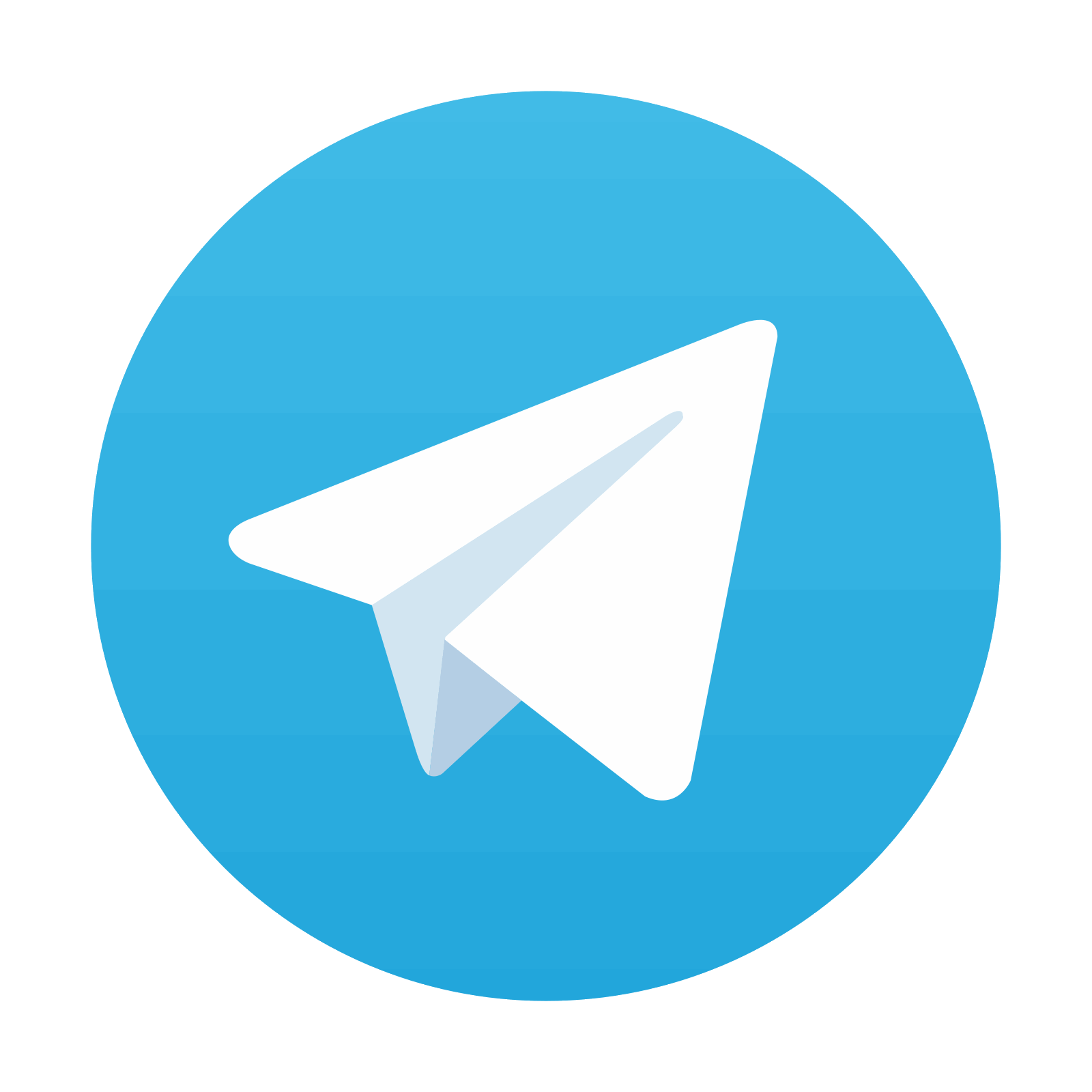
Stay updated, free articles. Join our Telegram channel

Full access? Get Clinical Tree
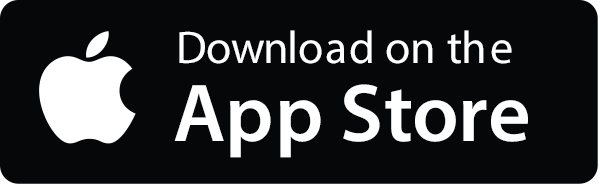

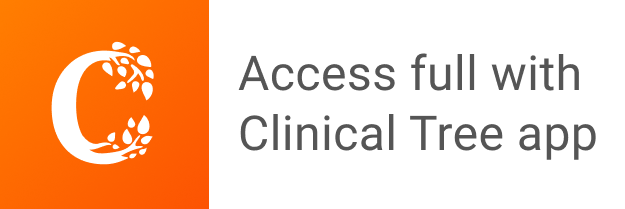