9
Integration of Genomics in Nursing Research: An Example
Karen E. Wickersham and Susan G. Dorsey
The groundbreaking accomplishments of the Human Genome Project (Guttmacher & Collins, 2003) transformed health care research. Innovative studies aimed at understanding the mechanisms underlying acute and chronic conditions have led to the discovery of new therapeutic strategies for many of those conditions (Jenkins, Grady, & Collins, 2005), including cancer, cystic fibrosis, and neuropathic pain. As we transition to an era of precision medicine (Precision Medicine Initiative [PMI] Working Group, 2015), the application of genomic research has implications for the prevention, diagnosis, treatment, and management of acute and chronic conditions, as well as the symptoms associated with these conditions (Jenkins et al., 2005). As the largest and most trusted group of health care workers in the United States and around the world (Calzone et al., 2010), nurses are uniquely positioned to participate in the translation of genomic research from “bench to bedside and back” (Conley & Tinkle, 2007, p. 17). This chapter addresses the integration of genomics in nursing research by:
• Defining the terms genetics and genomics and providing a brief overview of current technological methods used in genomic research
• Discussing the genomic nursing science blueprint and resulting research questions for nursing inquiry
• Discussing Exemplar 1: Genomic nursing research performed at the National Institute of Nursing Research
• Discussing Exemplar #2: Extramural nursing research examining neuropathic pain related to spinal cord injury
• Summarizing future directions for genomic nursing research
WHAT IS GENETICS/GENOMICS?
Genomic research is one of the many “omics” that are now being investigated in health care research (e.g., transcriptomics, metabolomics, epigenomics and lipid-omics, and others; Cheek, Bashore, & Brazeau, 2015). The terms genetics and genomics are often used interchangeably but, in fact, are different concepts. Genetics is the study of single genes and generally refers to heredity and inherited traits. Genomics refers to the study of the structure, content, function, and evolution of all nucleotide sequences in the entire genome of a species (Cheek et al., 2015; Johnson, Giarelli, Lewis, & Rice, 2013), and takes into account the influences of the environment, personal lifestyle, and psychosocial and cultural factors (Feero & Collins, 2010).
Current approaches to genomic research (Conley et al., 2013), from a methodological perspective, include sequence-based and array-based platforms (Biesecker, 2012; Rizzo & Buck, 2012). Both of these technology platforms can be used to conduct genomic studies such as genome-wide association studies (GWAS; Marian, 2012), gene expression profiling and transcriptomics analyses (Arao, Matsumoto, Maegawa, & Nishio, 2011), and the examination of differential DNA methylation, which is one important aspect of epigenomic analyses (Emes & Farrell, 2012). While a complete review of all platform technologies and their use is beyond the scope of this chapter, we briefly review the conceptual foundation for the conduct of GWAS, transcriptomics, gene expression analysis, and epigenomics, as well as the relative advantages and disadvantages of each methodological platform.
TYPES OF GENOMIC STUDIES
Genome-Wide Association Studies
GWAS are defined by the National Institutes of Health (NIH) as studies of common genetic variation across the entire human genome, and are designed to identify genetic associations with observable traits (Mitchell, Ferguson, & Ferguson, 2007)—in other words, to observe an association of a phenotype with a particular genotype. The goal of GWAS is to identify single nucleotide polymorphisms (SNPs) in genes that may be associated with disease or symptom susceptibility, or to provide new information about how a particular gene, set of genes, or genes in a canonical signaling pathway might be associated with disease outcomes and/or symptom severity. Several technology platforms, including array-based and sequence-based, can be used to conduct these types of studies. GWAS are performed in unrelated individuals with an attribute of interest (Conley et al., 2013); for example, osteoarthritis pain (Campbell, Trudel, Wong, & Laneuville, 2014; Hsu et al., 2015), which guides the understanding of genetic variation between those with and without disease.
In GWAS, hundreds of thousands of SNPs are examined for an association to a disease in thousands of individuals, ultimately allowing health care professionals to use the information to design effective strategies to screen for or predict disease (Feero & Collins, 2010). The GWAS are not guided by hypotheses. Rather, it is a hypothesis-generating approach that has the potential to identify disease-associated markers in genes that were not previously associated with the particular disease or symptom under study (Wung, Hickey, Taylor, & Gallek, 2013). One major challenge with GWAS as a method is that the GWAS results provide information about the association of functional genetic variants with a phenomenon of interest, but not about causation (Conley et al., 2013). GWAS require large sample sizes, which allows screening for many conditions simultaneously; however, GWAS results are usually limited to the patient sample from which they were developed and do not take into account information about environmental or other nongenetic risk factors (Conley et al., 2013; Pearson & Manolio, 2008). While GWAS results can be used to inform clinical practice and incorporating genomic research findings in clinical practice has been challenging, the results from GWAS can be helpful in initiating conversations with patients about other risk factors for disease (Conley et al., 2013). One example of success in incorporating GWAS findings in clinical practice is the testing of non-small-cell lung cancer (NSCLC) adenocarcinomas for variants in the gene that codes for the epidermal growth factor receptor (EGFR) proteins, which is now considered to be the standard of care (National Comprehensive Cancer Network® [NCCN] guidelines). Searching for these mutations prior to initiating treatment is crucial, because patients with NSCLC adenocarcinoma with sensitizing mutations in EGFR generally respond better to EGFR tyrosine kinase inhibitor therapy (Paez et al., 2004; Sequist et al., 2007). However, the mutations themselves are not predictive of patient survival.
Gene Expression Profiling/Transcriptomics
Gene expression profiling, often referred to as transcriptomics, investigates the relative expression level of messenger ribonucleic acid (mRNA) in various tissues or circulating plasma. The transcriptome refers to the entire set of mRNAs that are expressed within a single cell or tissue at a specific time or in response to disease or symptom burden (Wang, Gerstein, & Snyder, 2009). As an overall approach, transcriptomics is used to investigate underlying genomic mechanisms in health and disease (Spies et al., 2002). Typically, this involves using either sequence-based or array-based platforms to screen for changes in the expression of hundreds to thousands of genes and transcripts simultaneously (Zhou, Chen, Han, Wang, & Chen, 2014). A gene is defined as the locus, or region, of the genome that produces a mature mRNA that is translated into a protein. A transcript refers to the set of alternatively spliced isoforms that are produced by a single gene locus (Gingeras, 2007). Transcriptome analysis is particularly useful to examine the molecular features of a disease or symptom before and after treatment at a specific point in time or to examine expression changes in cases versus controls. Transcriptome data can be used to identify candidate genes or canonical pathways that were altered at the mRNA level that can then be used to predict susceptibility or offer insights into potential new therapeutic targets for further analysis (Harrington, Rosenow, & Retief, 2000; Kurella et al., 2001; Noordewier & Warren, 2001).
Transcriptomic analysis has become especially important in determining the risk of recurrence and survival for women with early-stage breast cancer (Solin et al., 2012). For example, Oncotype DX™ (Genomic Health, Redwood City, California, www.genomichealth.com) is a validated, multigene, expression-based assay that provides information about individualized prognosis, targeted treatment, and surveillance of 21 genes based on the biology of a patient’s breast cancer (Albain et al., 2010; Dowsett et al., 2010; Paik et al., 2004, 2006; Solin et al., 2012). The test is meant for use in newly diagnosed women with early-stage (stage I, II, or IIIa), node-negative or node-positive, and estrogen-receptor-positive (ER+), human epidermal growth factor receptor 2 (HER2)-negative breast cancer. Both the American Society of Clinical Oncology® and the NCCN have incorporated Oncotype DX testing into their treatment guidelines for breast cancer (Sparano et al., 2015). Exemplar #2 later in this chapter reviews the use of transcriptomics to identify a potential new therapeutic pathway target to treat chronic neuropathic pain following spinal cord injury (SCI).
Epigenomics
Epigenomics is the study of DNA, using either array-based or sequence-based platforms. But, unlike GWAS, epigenomics produces data related to chemical and structural changes in DNA that impact gene regulation (e.g., changes in methylation) rather than providing nucleotide sequence information (Conley et al., 2013). Disruption of epigenetic networks due to histone modifications, localized changes to chromatin structure, activities of noncoding ribonucleic acids (RNAs), and DNA methylation can result in heritable silencing of genes without changing their coding sequence (Egger, Liang, Aparicio, & Jones, 2004), and can impact injury, illness, pain, and treatment response (Petronis, 2010). For example, because many complex pain conditions such as rheumatoid and osteoarthritis are not associated with heritable factors (Lessans & Dorsey, 2013), epigenomic research has helped to identify potential mechanisms of pain and areas of potential therapeutic response (Lessans & Dorsey, 2013; Mogil, 2012).
METHODS FOR GENETIC AND GENOMIC ANALYSES
Next-Generation Sequencing (NGS)
NGS methods directly examine the DNA or RNA sequence and can be used to conduct high-resolution mapping of 5’—C—phosphate—G—3’ (CpG) dinucleotide methylation of DNA to examine epigenomics (Conley et al., 2013; Lan et al., 2011; Metzker, 2010). Genome sequencing uses high-throughput technology to provide information about the order of or methylation status of nucleotide bases in a predetermined length (typically 25–150 base pairs), which is termed a short read. Prior genome sequencing technologies accomplished this by examining one molecule at a time using bidirectional Sanger sequencing (Katsanis & Katsanis, 2013); however, advances in technology have made it possible to investigate millions of nucleotides simultaneously (Conley et al., 2013). NGS has become more efficient, with corresponding increases in sequence throughput. Thus, it has also become less expensive over the past 10 years (Figure 9.1; National Human Genome Research Institute [NHGRI], 2015), and it is likely that a sub-$1,000 whole genome sequence will become a reality.
Advantages of NGS include direct capture of sequence data at a digital resolution of individual nucleotides or methylation of CpG sites that can be mapped back to a reference genome; the ability to detect novel SNPs, genes, and transcripts; and the ability to simultaneously interrogate the entire genome, transcriptome, or methy-lome (Malone & Oliver, 2011). Challenges include the requirement of a multinode computational infrastructure, the need for significant amounts of data storage (files can be terabytes in size), and the need for significant bioinformatics support to implement open-source workflows in R or other programming languages to perform genome alignments and data analysis.
FIGURE 9.1 DNA sequencing costs from the National Human Genome Research Institute (NHGRI). The costs associated with DNA sequencing performed at sequencing centers funded by the NHGRI from 2001 to 2015 (NHGRI, 2015). Costs include labor, administration, management, utilities, reagents, consumables, sequencing instruments and other large equipment, informatics activities directly related to sequence production (e.g., laboratory information management systems and initial data processing), and submission of data to a public database. Data from 2001 to October 2007 represent the costs of generating DNA sequence using Sanger-based chemistries and capillary-based instruments (“first-generation” sequencing platforms). Data beginning in January 2008 represent the costs of generating DNA sequence using “second-generation” (or “next-generation”) sequencing platforms.
Source: NHGRI (2015).
Microarray and Other Array-Based Technologies
DNA microarrays can be used to conduct GWAS, transcriptomic, and epigenomic studies. Modern arrays are fabricated on silicon, glass, or plastic substrates and have thousands of probe sets designed to capture small DNA, RNA, or methyl-ated CpG sites (Heller, 2002). In general, labeled complementary (c) RNA or cDNA is hybridized to the arrays and then the fluorescence signal intensity generated by hybridization with individual probe sets is scanned for analysis. Unlike NGS, where each nucleotide is read directly, array-based analysis depends upon transforming the analog fluorescence signal to a value representing relative abundance of gene or transcript expression or calling a SNP for GWAS (DNA). Advantages of microarray technology include relatively short processing time from total RNA or DNA to results that can be analyzed (typically two days), straightforward and mature data analytic workflows that can be implemented in open-source platforms or commercial software packages, the ability to analyze results on most typical desktop computers without the need for advanced computational infrastructure, and relatively small data storage requirements. In our experience, challenges include difficulties inherent in transforming analog to digital signals, off-target hybridization, and limitation of the analysis to the a priori content on the array. So, novel findings are limited.
In sum, we have provided a brief review of current approaches to genomic research that can be used in GWAS, transcriptomic, and epigenomic studies to conduct innovative research aimed at understanding underlying mechanisms of acute and chronic conditions or symptoms and guiding the discovery of new treatments, therapies, or management strategies for those conditions or symptoms. The next section discusses how these approaches may be included in a blueprint for genomic nursing research.
THE BLUEPRINT FOR GENOMIC NURSING SCIENCE
Advances in technologies utilized in conducting genomic research are changing the approach to screening, diagnosis, treatment, prevention, prognosis, and surveillance of chronic illness (Calzone et al., 2012). Because nurses are the largest and most trusted group of health care workers (Calzone et al., 2010; U.S. Department of Health and Human Services, Health Resources and Services Administration [HRSA], Bureau of Health Professions, 2010), they are uniquely poised to implement genomics into clinical nursing and research as a discipline (Calzone et al., 2010). As such, the NIH and HRSA have led several initiatives to prepare nurses to apply genomics in patient care (Jenkins et al., 2005). For example, in 2004, the NHGRI and the National Cancer Institute collaborated to establish the U.S. Genetic/Genomic Nursing Competency Initiative (GGNCI). The GGNCI obtained agreement on essential genomic nursing competencies that apply to all registered nurses regardless of specialty, role, or academic degree (Jenkins & Calzone, 2007). In 2012, the GGNCI published graduate genetic and genomic competencies (Greco, Tinley, & Seibert, 2011). Parallel efforts by the NIH included the Summer Genetics Institute (SGI), which is sponsored by the National Institute of Nursing Research (NINR) and provides participants with a foundation in molecular genetics appropriate for use in research and clinical practice.
In 2012 the NHGRI of the NIH convened a Genomic Nursing State of the Science Advisory Panel to determine gaps in genomic nursing science and to create a blueprint to focus research to address those gaps (Calzone et al., 2013). The primary goals of the advisory panel were to: (a) review and evaluate the available evidence on genetic/genomic competency and nursing practice, and (b) establish a research agenda based on a systematic evaluation of the current state of the science (Calzone et al., 2013). The advisory panel concluded that the two key areas for nursing research would generate evidence about how genomic nursing can contribute to improved client (i.e., person, family, communities, and populations) health outcomes, taking into account the context in which health care is delivered. A description of the blueprint is found in Table 9.1. Four main themes concerning a need for genomic research were identified by the advisory panel: (a) health promotion and disease prevention, including risk assessment, communication, decision support; (b) advancing quality of life for individuals and their families, including symptom management, management of disease states, client self-management; (c) innovation, including technology development, informatics support systems, and environmental influences; and (d) training (e.g., capacity building, education; Calzone et al., 2013). Cross-cutting themes included health disparities, cost, policy, and public education.
As a follow-up to the blueprint, NINR held a Genomic Nursing Science Workshop in August 2013 (NINR, 2013). During this workshop, experts from diverse disciplines identified research questions that were aligned with the NINR’s strategic plan and that would guide genomic nursing research in the areas of symptom science, wellness, self-management, end-of life, and palliative care. Specific research questions, which can be located on the NINR website (www.ninr.nih.gov)included:
• What are the biological, physiological, or omic mechanisms underlying symptoms and patient outcomes?
• Based on individual omics, environmental factors, and behavior, what are the most effective and targeted interventions that can be expedited for translation to reduce risk and promote health?
• What are the relative contributions of omic markers and phenomic data in predicting individual responses to therapeutic interventions that improve patient outcomes such as quality of life?
• For high-risk patients who are at the end of life, how can genetic assessment and DNA banking be used to address familial risk?
TABLE 9.1 Nursing Genomic Science Blueprint Mapping to the NINR Strategic Plan Areas
NINR Strategic Plan Areas | Specific Nursing Research Categories | Advisory Panel Genomic Nursing Research Topic Areas |
Health promotion and disease prevention | Risk assessment | a. Biological plausibility (e.g., pathways, mechanisms, biomarkers, epigenetics, genotoxicity) b. Comprehensive screening opportunities (e.g., family history, identify risk level [population-based average and elevated]) c. Components of risk assessment (e.g., biomarkers, family history) d. Risk-specific health care decision making |
Communication | a. Risk communication (e.g., interpretation, timing, risk reports to the health care provider and client) b b. Informed consent c. Direct-to-consumer marketing and testing (e.g., uptake, utilization, dissemination) | |
Decision support | a. Informed consent b. Match of values/preferences with decision made c. Risk perception/risk accuracy d. Effect of decision support on decision quality (e.g., knowledge, personal utility) | |
Advancing the quality of life | Family | a. Family context (e.g., family functioning and structure, family relationships, and communication) b. Ethical issues c. Health care provider communication with families |
Symptom management | a. Biological plausibility (e.g., pathways, mechanisms, biomarkers, epigenetics) b. Clinical utility c. Personal utility d. Pharmacogenomics (e.g., therapy selection, medication titration) e. Decision making f. Evidence-based effectiveness of approaches | |
Disease states (encompassing acute, common complex and chronic) | a. Genomic-based interventions that reduce morbidity and mortality b. Gene/environment interactions (e.g., epigenetics, genotoxicity) c. Pharmacogenomics d. Evidence-based effectiveness of treatments/support | |
Client self-management | a. Collecting and conveying information that informs self-management (e.g., family history) b. Lifestyle behaviors c. Environmental exposure and protection (e.g., occupational) d. Synergy of client and provider expectations (e.g., client/family-centered care) e. Personal utility | |
Innovation | Technology development | a. Incorporation of new technologies (e.g., whole genome sequencing) b. Ethics c. Policy and guidelines to support applications d. Applications (e.g., clinical and analytic validity, clinical utility) e. Genomic bioinformatics f. Translation, dissemination, implementation i. Use of technology in information delivery ii. Performance improvement by provider (e.g., point-of-care support) iii. Resources that support genomic research (e.g., registries of tools, best practices, nursing outcomes) |
Informatics support systems | a. Data storage and use to facilitate research process and outcomes b. Facilitate cross-generational sharing of genomic data (e.g., family history, laboratory analyses) c. Managing, analyzing, and interpreting genomic information (e.g., sequencing data) d. Point-of-care decision support for client and health care provider e. Common terminology and taxonomy f. Common formats for data storage/exchange and queries | |
Environmental influences (encompassing physical, social environments and policy context) | a. Evidence-based guidelines b. Health care reform c. Economics (e.g., cost-effectiveness) d. Regulatory gaps and/or variability | |
Training | Capacity building | a. Training future nursing scientists in genomics b. Preparing nursing faculty in genomics c. Education of current and future workforce in genomics (e.g., research nurse coordinators, advanced practice nurses, other health care professionals) d. Preparation of nurse scientists to lead interprofessional teams e. Preparation of clinical and administrative leaders to advance appropriate genomic/genetic integration into practice f. Innovative uses of biorepositories (e.g., informed consent, result interpretation) g. Bioethics |
Education | a. Optimal methods to train the existing nursing workforce in genomics b. Optimal methods to train the nursing leadership in genomics to support genomic translation, research, and practice c. Optimal methods to integrate nursing genomic competencies in basic prelicensure and postlicensure in academic programs | |
Cross-cutting themes | Health disparities | a. Racial, ethnic, socioeconomic, and cultural influences on disease occurrence and response to disease and treatment b. Genomic health equity (e.g., access) c. Diseases that disproportionately affect specific groups (e.g., minorities) d. Targeted therapeutics e. Overcoming misinformation and genomic “myths” |
Cost | a. Cost-effectiveness b. Comparative effectiveness c. Value | |
Policy | a. Policy as context of science b. Research to inform policy | |
Public education | a. Health literacy b. Genomic literacy |
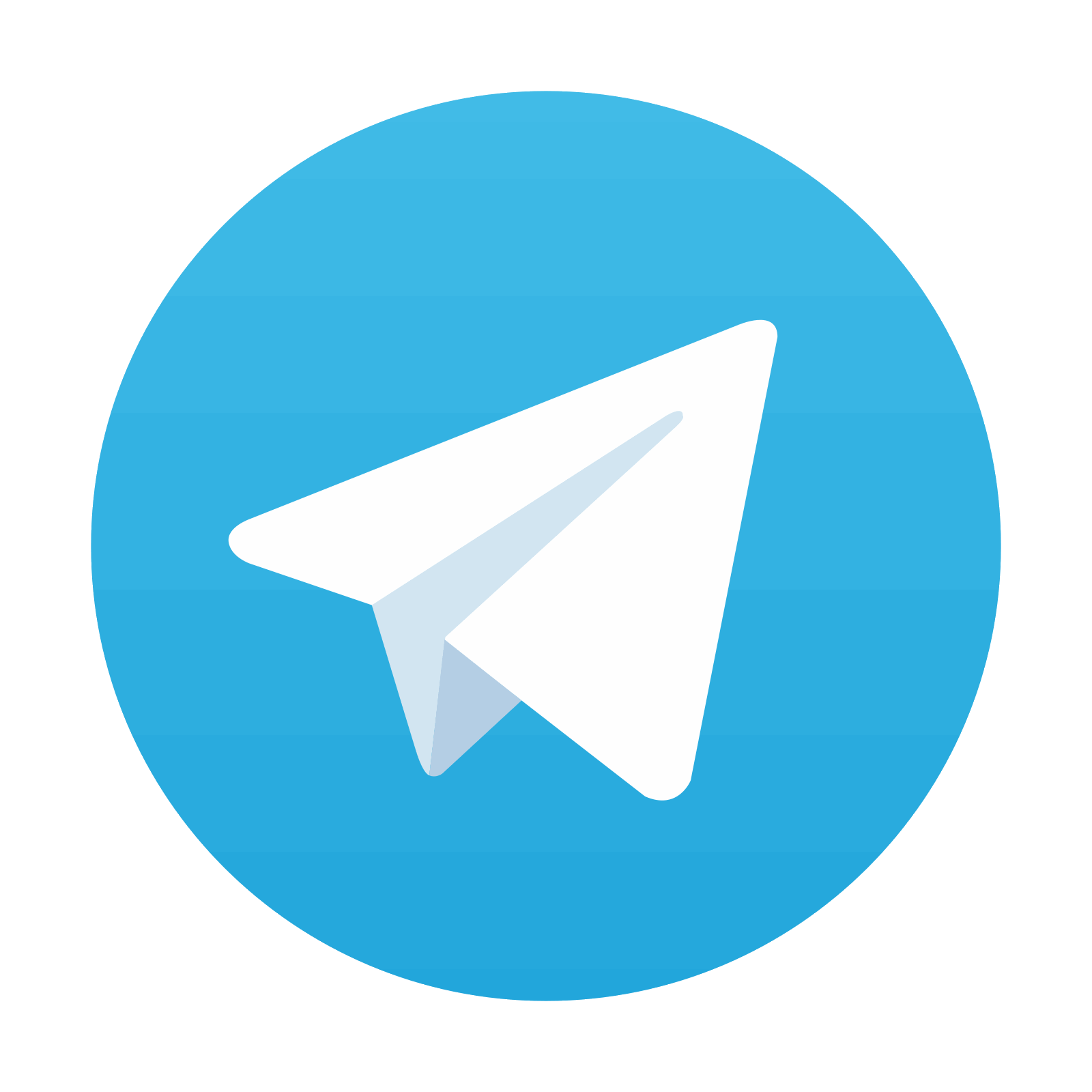
Stay updated, free articles. Join our Telegram channel

Full access? Get Clinical Tree
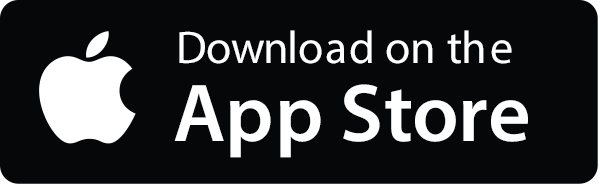
