12. Experiments
Key points
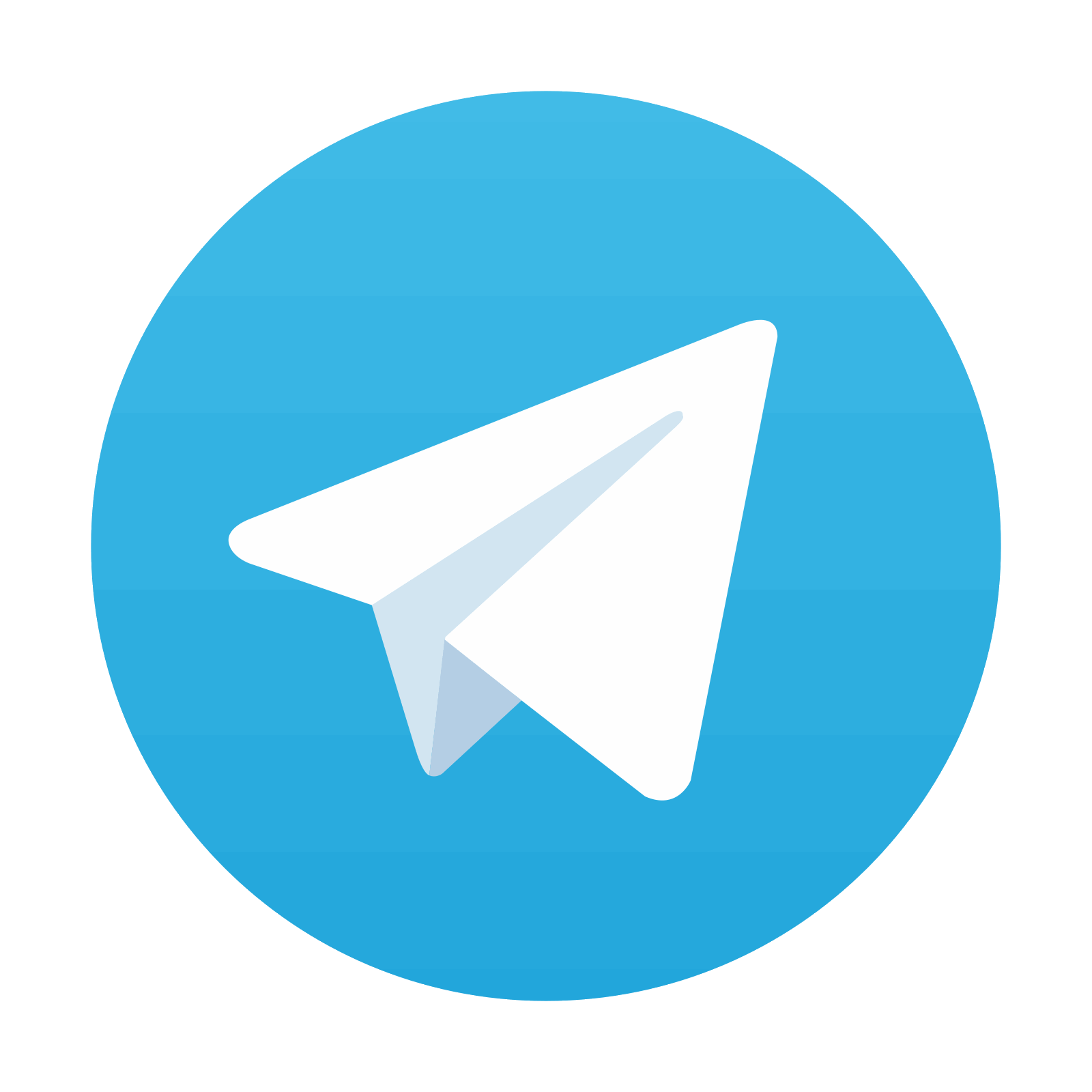
Get Clinical Tree app for offline access
• Experimental designs, particularly in the form of the randomised control trial, have become one of the most respected types of research in evidence-based practice. The reason for this relates to the way that drugs and treatments in the past have been carefully tested to reduce the possibility of other explanations for the results.
• The way experimental studies are carried out can be very complex because they are dependent on the three necessary experimental elements of randomisation, control, and manipulation. In midwifery, it is not always possible, or desirable, to achieve these elements. Sometimes it would be unethical, or would drastically reduce women’s choice or individual midwife’s judgement as to what was best in the particular circumstances, if strict experimental protocols were followed.
• There are alternatives to a full experimental design, such as quasi-experimental, ex post facto and correlation designs. Although these do not produce conclusions that are as ‘strong’ as experimental designs, they can still inform evidence-based practice.
• Despite the status given to experimental designs, they do have limitations. It is not always possible to control for other factors that might explain the results. In addition, it is sometimes an oversimplification to look for one cause for a phenomenon; sometimes there are several.
• The power of this type of design depends on the use of statistical methods, particularly inferential statistics. These identify the role of chance in explaining the difference in the results between groups. The knowledge required to understand this form of research is more demanding than in other methods. However, midwives should not see this as a reason for avoiding experimental approaches, or avoid reading published experimental studies. The effort needed to gain the statistical knowledge and understanding is well worth the reward of being able to confidently use and challenge this research approach.
Evidence-based practice has increased the demand for research that can unambiguously demonstrate the best options for clinical care. Experimental design has established itself as the most widely recognised and respected source of such evidence. In medicine, the experiment frequently takes the form of the randomised control trial (RCT). This method of collecting research data has become so powerful in determining the effectiveness of treatments that it is used by some as a measure against which all other methods are compared. As many clinical procedures in maternity care are influenced by experimental research, it is crucial that midwives can evaluate such studies and not accept them without question.
The purpose of this chapter is to consider the basic principles of experimental design, and to recognise the strengths, as well as the limitations, of this approach. As experiments can be designed in a number of ways, the chapter will also outline some of these various forms.
Why are experiments special?
Experiments are highly regarded in health care and have been traditionally associated with the idea of ‘scientific method’. This may be due to the belief that they are more accurate or ‘objective’ than other forms of data collection. This has led to their prominent position in ‘hierarchies of evidence’ that attempt to indicate the most reliable sources of information. The result is that hierarchies ‘privilege’ the RCT in a way that makes them the main source of knowledge within medicine (Spiby and Munro 2010).
Why do experimental designs have such a high status in health care, particularly in regard to evidence-based practice? The answer lies in what they can achieve and the characteristics they possess. Firstly, they have provided the basis on which a great deal of our current health care knowledge and theory has been based, especially in the form of the randomised control trial. RCTs, according to Burns and Grove (2009), provide the strongest research evidence for practice. This is because they examine the likelihood of a cause-and-effect relationship between variables through the use of statistical calculations. Such calculations determine the extent to which the results of an experiment could have happened by chance and is indicated by the ‘p’ value. This takes the form of decimal number often found in or under a table of results or in the text of a research article. It is reasonably easy to interpret this once you are familiar with the basic idea underpinning probability (see Box 12.1).
BOX 12.1
Probability values
Probability values indicate the extent to which the difference in the results between two groups could have happened by chance. The ‘p’ stands for ‘probability’. This translates into how many times out of a hundred, or even a thousand, the difference between two groups of data could happen purely by chance. The smaller the likelihood that a difference could have happened by chance, the more certain we can be that the experiment has demonstrated a cause-and-effect relationship. In other words, the intervention does produce the desired effect.
The value of ‘p’ is expressed as a decimal, and has to be converted to a fraction to work out the element of chance. Take the example of ‘p<0.05’. We first convert 0.05 to a fraction by drawing a line underneath the numbers so that they become the top line of the fraction; then put a ‘1’ underneath the decimal point, and a ‘0’ underneath every figure after the point. This may sound complicated, but if you write it out for yourself, 0.05 becomes 05/100. In other words, the likelihood of the difference between the results of two groups in the study happening purely by chance is less than 5 in 100 times. Or, put another way, 95 times out of 100 the effect you wanted will be produced by the intervention used in the study.
This figure of p<0.05 is regarded as the minimum value that may suggest a relationship between the dependent and independent variable. Notice that there is still a margin of error. It does not mean that one thing definitely causes the other; the results would have happened purely by chance 5 in 100 times. This means that for 95% of the time you can be satisfied that a cause-and-effect relationship does exist.
The most frequently used values to indicate probability are as follows:
P value | Probability of difference happening by chance |
---|---|
<0.05 | less than 5 in 100 |
<0.01 | less than 1 in 100 |
<0.001 | less than 1 in 1000 |
NS | non-significant (i.e. the probability that chance is responsible for the result is so large that a ‘p’ value is not used). |
It is recommended that you consult a statistics book for more information.
Characteristics of experimental design
What are the essential features of an experiment? Unlike other methods (apart from action research), the experiment is a form of research where the researcher is active in the situation and not just a gatherer of information. The researcher makes something happen and is responsible for controlling the way that something is introduced into the situation. So a common form of the experiment is where there are two groups of participants and the researcher will introduce an intervention to one group but not the other and then see if those in the experimental group have a different outcome to those in the control group. See Box 12.2 for an example.
BOX 12.2
Example of a randomised control trial of extended midwifery support (EMS) on the duration of breastfeeding (McDonald et al. 2010)
The aim of this Australian RCT set in a large public teaching hospital was to evaluate the effects of an extended midwifery support (EMS) programme on the proportion of women who breastfeed fully to 6 months. The sample consisted of 849 women who consented to take part in the study. Participants must have given birth to a healthy, term, singleton baby and wished to breastfeed. The women were allocated at random to either the extended support group (independent variable), where they were offered a one-to-one postnatal educational session and weekly home visits with additional telephone contact by a midwife until their baby was 6 weeks old, or to the standard postnatal midwifery support group (control). The women were first stratified for parity and education level. The main outcome measures (dependent variables) were the prevalence of full and any breastfeeding at 6 months postpartum. The results showed that there was no difference between the groups at 6 months postpartum for either full breastfeeding or any breastfeeding. The researchers concluded that the EMS programme did not succeed in improving breastfeeding rates in a setting where there was already a high initiation of breastfeeding.
According to Burns and Grove (2009: 262), the three elements that confirm a study as a true experiment are:
• randomisation,
• researcher-controlled manipulation of the independent variable (the experimental variable),
• researcher control of the experimental situation, including a control or comparison group.
Together, these three elements help to rule out alternative ways of explaining a particular outcome to a study other than the variable introduced by the researcher. Each of these elements will now be examined.
Randomisation
Randomisation is a term that may apply to both the sampling procedure used in a study (see Chapter 14), and the allocation of individuals to an experimental (sometimes called intervention) or control group. Random sampling occurs when every member of a study population (all those with the relevant characteristics, such as those going home from a midwifery-led unit over a 3-month period) has an equal chance of being included in the study. This is not easy to achieve in a total group, as individuals must first agree to take part in a clinical study; it is not simply a case of picking them out of a population and expecting them to accept a form of intervention allocated to them. In most cases, randomisation refers to random assignment or random allocation. This is the process of allocating participants to either the experimental or control group in a random manner once they have agreed to take part in the study. In other words, an individual entering the study should be allocated to a treatment or intervention group in a way that ensures they have an equal chance of being in either group. The exact method used to randomise those in a study will be explained in Chapter 14. It is a very precise and methodical system and it not ‘haphazard’, which is a misunderstanding of the term.
The purpose of randomisation is to reduce the possibility of bias where people with certain characteristics that might affect the outcome are unevenly distributed between the two groups in a study. The implication of this is that the groups would initially differ from each other, which would make it impossible to rule out the influence of factors built in to the characteristics of those in the two groups. Nelson et al. (2010) support this by stating that experiments are based on the assumption that the groups were similar at the start of the experiment before anything is introduced. If they differ at the end, then it is easier to argue that the difference is due to the experimental variable. Randomisation also ensures that additional factor, called ‘confounding variables’, that may also influence the results are evenly distributed between the two groups. In other words, randomisation should allow the researcher to compare like with like.
In experimental design, the existence of a comparison group that does not receive the independent variable is crucial. The role of the control group is to act as a comparison by establishing what the typical outcome would be if the experimental variable had not been introduced. In evidence-based practice, this is important in deciding whether an intervention would make any difference to the outcome? The control group theoretically remains the same over the experimental period, as they do not receive the treatment or intervention that forms the independent variable. This allows the investigator to reduce the effect of what has variously been called the ‘attention factor’, or the ‘Hawthorne effect’ (this will be explained in more detail later in this chapter). These terms relate to a phenomenon where individuals report a change influenced by their participation in a study. In other words, a change in the dependent variable may be due to a feeling of being ‘special’ and which produces a reaction that ‘mimics’ a real change.
Not all studies have a separate control group. One group can receive two interventions in turn, for example, a conventional approach followed by an experimental approach. In this way, individuals act as their own control (Nelson et al. 2010). It is also possible for two separate groups to receive the same two interventions, but in a different order. Here again, they are acting as their own controls in that they receive both interventions and rule out the possibility that any differences are the result of varying characteristics of those in the two groups. This kind of approach is referred to as a cross-over design study.
Manipulation
The second feature of experimental design is manipulation, which means the experimenter manipulates or introduces the independent variable, usually an intervention or treatment to the experimental group, but withholds it from the control group who receive either an alternative, or nothing (Polit and Beck 2008). In the words of Smith (2008), if there is no intervention introduced by the researcher then there is no experiment! In the example in Box 12.2, the researchers made available to one group extended midwifery support in the form of a one-to-one postnatal educational session, weekly home visits and additional telephone contact by a midwife until their baby was 6 weeks old, while the control group had routine care.
Control
Control is the final feature of experimental design, where the researcher reduces the possible effect of other independent variables on the outcome measure of the study. This means that the experimenter must have the ability to control not only the independent variable but also other elements within the experimental setting that might make a difference to the dependent variable (outcome measure). For example, they must ensure that everyone in the study has an equal chance of being in the experimental group (random allocation). If this is achieved, then the researcher can say that they have controlled for extraneous factors that may influence the dependent variable. In the study in Box 12.2., the researchers controlled for parity and educational level, which might have had an impact on the outcome by first putting the group into parity and education subgroups and randomly choosing from each group so that these factors were equalised through the design of the study
It is the researcher’s ability to achieve maximum control that illustrates the degree of rigour in the study. This includes control over the way any interventions are provided. All procedures must be applied in exactly the same way to each individual so that consistency is achieved and other possible explanations for differences in outcomes eliminated. Measurements of the dependent variable should also be under the control of the researcher. The measuring instrument should be accurate and consistent, and where more than one person is involved in the measurement, the researcher should ensure that everyone is measuring in the same way. This is called inter-rater reliability.
Taken together, we can see all three features of an experiment make extraordinary demands on the skills and power of the researcher and make experiments a very complex form of research.
Blinding
Before moving on, there are two important aspects of control in medical or obstetric research that need to be highlighted, as they are becoming more important in assessing the rigour of those experimental designs with clinical interventions and objectively measured outcomes. These are allocation concealment and blinding. At the start of a study, as individuals are being allocated to the experimental or control group, it is essential that those carrying out the allocation cannot anticipate the group to which the next person will be allocated. This is so they do not tamper with who goes where, on the basis of their knowledge of what the person might receive. Blinding or masking means that those in the study do not know the intervention or treatment an individual has received. This is an attempt to maintain the objectivity of the method by protecting the results from the accusation that they are inaccurate as they have been spoilt or compromised by poor design. Using sealed envelopes so that it is not possible to anticipate the allocation until the envelope has been opened is frequently used in clinical trials to avoid the problem of allocation concealment. Blinding is more complicated and involves more people, as the risk of bias can come from several sources. It involves the risk of people acting differently if they know to which group the individual has been allocated during the course of a clinical trial. Smith (2008) suggests that if masking is not carried out adequately there is an increased chance that measurement estimates can be subconsciously raised in favour of the experimental intervention. Single blinding is where either the person receiving an intervention or the person measuring the outcome is shielded from knowing whether the individual was in the experimental or control group. Double blinding is where both the study individual and those providing care or measuring the outcome are unaware to which group the individual was allocated.
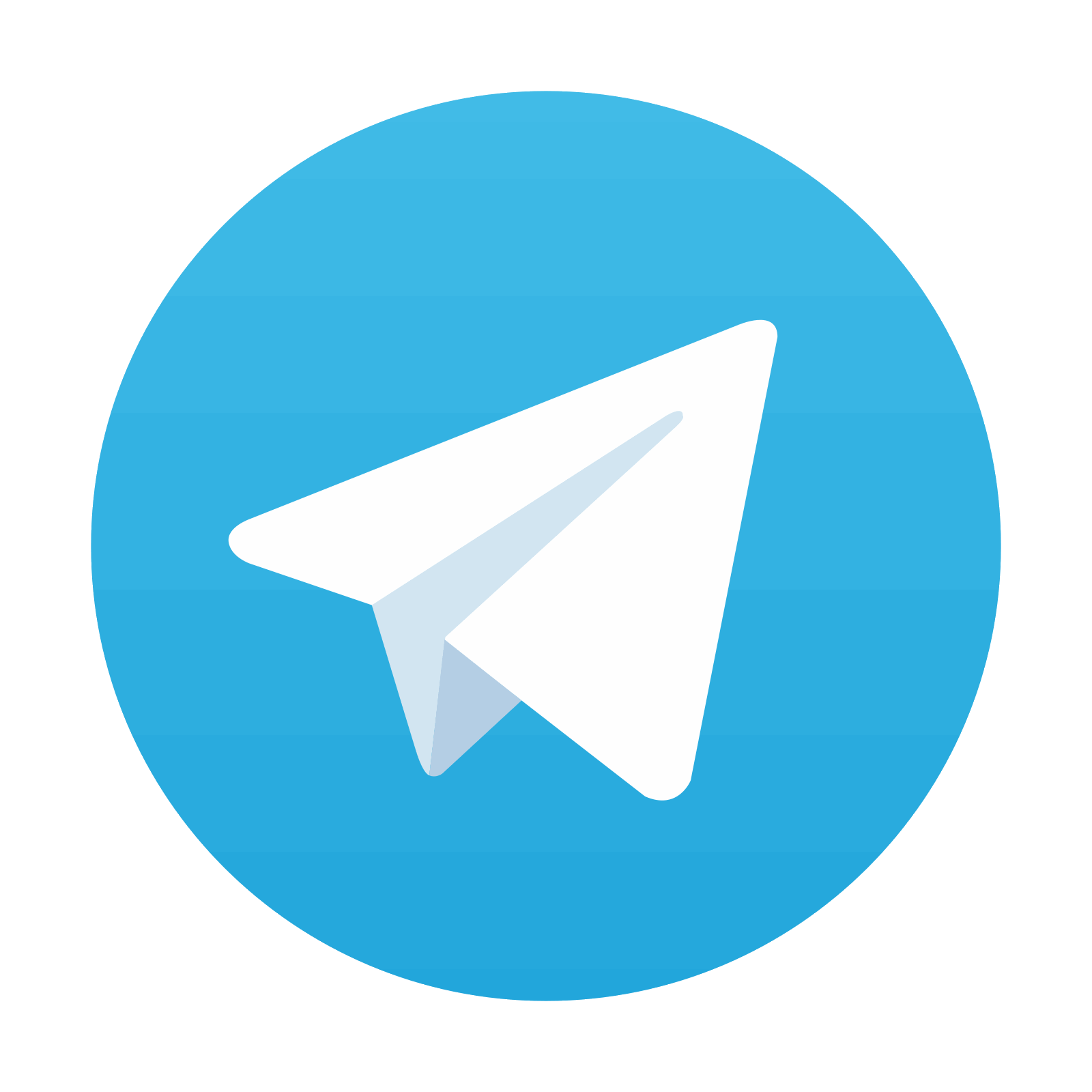
Stay updated, free articles. Join our Telegram channel

Full access? Get Clinical Tree
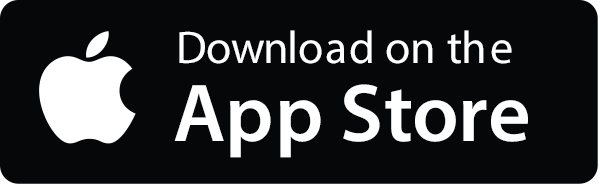

Get Clinical Tree app for offline access
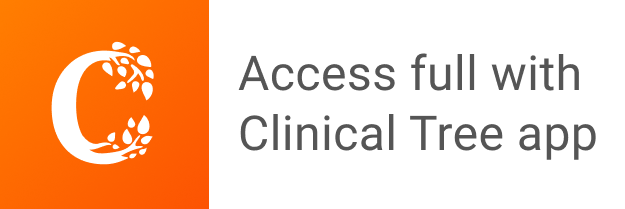