Chapter 4 Epidemiology
Defining epidemiology
Epidemiology can be defined as:
The study of the distribution and determinants of health-related states or events in specified populations, and the application of this study to control of health problems [emphasis added]. (Last 1995 p 55)
Distribution refers to the pattern or frequencies of health events by person (who gets affected), place (where it happens) and also time (when it happens). We will talk more about this when we consider ‘person, place and time’ in more detail, together with the ways we capture and measure health outcomes. Determinant refers to both the causes of, and risk factors for, health events. These can include any aspect of the environment we live in (biological, physical, cultural, social, etc.) including living organisms (e.g. viruses and bacteria), physical entities (e.g. radiation, pollution and dangerous machinery), lifestyle (e.g. stress and diet), social factors (e.g. poverty), and genetic factors (e.g. inherited or changed genes that cause genetic diseases). We will revisit health determinants when we consider how we might measure these exposures when trying to understand the patterns of disease that we see in populations. The World Health Organization (WHO) defines health as ‘a state of complete physical, mental, and social wellbeing and not merely the absence of disease or infirmity’ (WHO 1948). Health could include a specific disease state, the absence of a disease state, a quality of life rating, life expectancy or the incidence of mental illness or physical injury. Population refers to a group of people with definable commonalities, for example, the people who reside in a certain city or country, a school community or a certain age or ethnic group. Control of health problems refers to reducing the burden of a health problem in a population. Epidemiology quantifies the burden of disease, the strength of association between exposures and health states, the magnitude of risk at the population level, and the potential benefit of interventions, and, through this, drives evidence-based health policy and practice.
A brief history of epidemiology (1700s onwards)
Looking at the history of epidemiological ideas allows you to understand how scientific discoveries have fundamentally changed the ways in which humans see themselves. These days, we take it for granted that many diseases can be prevented through, for example, good nutrition. However, this idea had not been proven prior to James Lind’s work in preventing scurvy among British sailors by including citrus fruit in their diets in 1747 (The James Lind Library, see ‘Useful websites’ at the end of the chapter). Perhaps the best-known historical examples of epidemiological studies are those of John Snow and his investigations of cholera epidemics in London during the mid 1800s.
John Snow was a British physician who is considered by many epidemiologists to be the father of modern epidemiology. His story was set in nineteenth-century London, where millions lived an impoverished life in overcrowded and unsanitary slums, susceptible to devastating outbreaks of infectious diseases such as cholera. During the early 1800s, it was thought that cholera was airborne; however, Snow did not believe in the ‘miasma’ (bad air) theory, and was certain that the infectious agent entered the body through the mouth. In 1849, he published an essay entitled On the Mode of Communication of Cholera and, in August 1854, when a cholera outbreak occurred in Soho, London, Snow used epidemiological investigative skills to identify a water pump in Broad Street as the source of the disease. He presented his findings to the local government officials, and they decided to remove the handle of the pump the next day. The cases of cholera immediately began to diminish. Although John Snow’s ‘germ’ theory was not accepted until after his death, he was a prominent physician and certainly one of the founders of epidemiology. Should you wish to read more about the life and times of John Snow, there are a number of online resources available (see ‘Useful websites’ at the end of the chapter).
Measuring the occurrence of exposures of interest and of health outcomes
Prevalence refers to the number of people in a defined population who have a specific disease or condition or exposure at a certain point in time (e.g. at the time the data were collected in a national health survey). Prevalence data can provide an indication of the extent of a health problem (number of cases and severity of disease) and thus assist in the planning of health services. Measuring prevalence basically involves counting cases. However, data on prevalence are much more meaningful if converted into a proportion, by dividing the count by the total number of people in the population from which the cases arose (e.g. the number of people who completed the health survey). Prevalence can be reported as a percentage (i.e. multiply by 100), or per 10 000 or 100 000 in the population if it is a rare event (Example 4.1).
Prevalence is calculated using the following equation:
It is worth taking a little time to think about why we need both incidence measures. Many epidemiological studies follow people over time to see who develops certain health outcomes in a population deemed to be ‘at risk’. Not everyone will be followed up for the full study period (some drop out of the study, die from other causes, etc.), and we need to take this into account so that we do not underestimate disease incidence relative to time at risk. For example, say in a group of 100 people who have had a coronary artery bypass graft, 24 have had a myocardial infarction (MI) after 2 years. This gives a 2-year incidence risk of 24%. This could also be expressed as a risk of 12 MIs per 100 person-years. However, that assumes that everyone was followed up for the full 2 years; but instead you find that on average, the follow-up time was really only 18 months. This should then be reported as 12 MIs per 150 person-years (which is the same as saying 16 MIs per 100 person-years). So you can see we may underestimate the disease incidence, or disease risk if we did not take into account the variation in the individual follow-up periods (Example 4.2).
Example 4.2 Incidence of hearing loss among workers in heavy industry
What is the cumulative incidence of hearing loss in these workers?
Incidence rate and cumulative incidence are calculated using the following equations:
If you would like to read further on the concept of person-time see Essential Epidemiology (Webb et al. 2011 pp 41–45).
Most introductory epidemiology textbooks discuss the measures described above; if you are interested in reading further see Epidemiology (Gordis 2004).
Epidemiological study design
Study designs differ with respect to the number of observations made, whether data are collected prospectively or retrospectively, the data collection procedures used, whether individuals or groups are studied, and the availability of subjects or existing data. Epidemiologists use a range of study designs, as illustrated in Figure 4.1.
Observational epidemiology
Activity
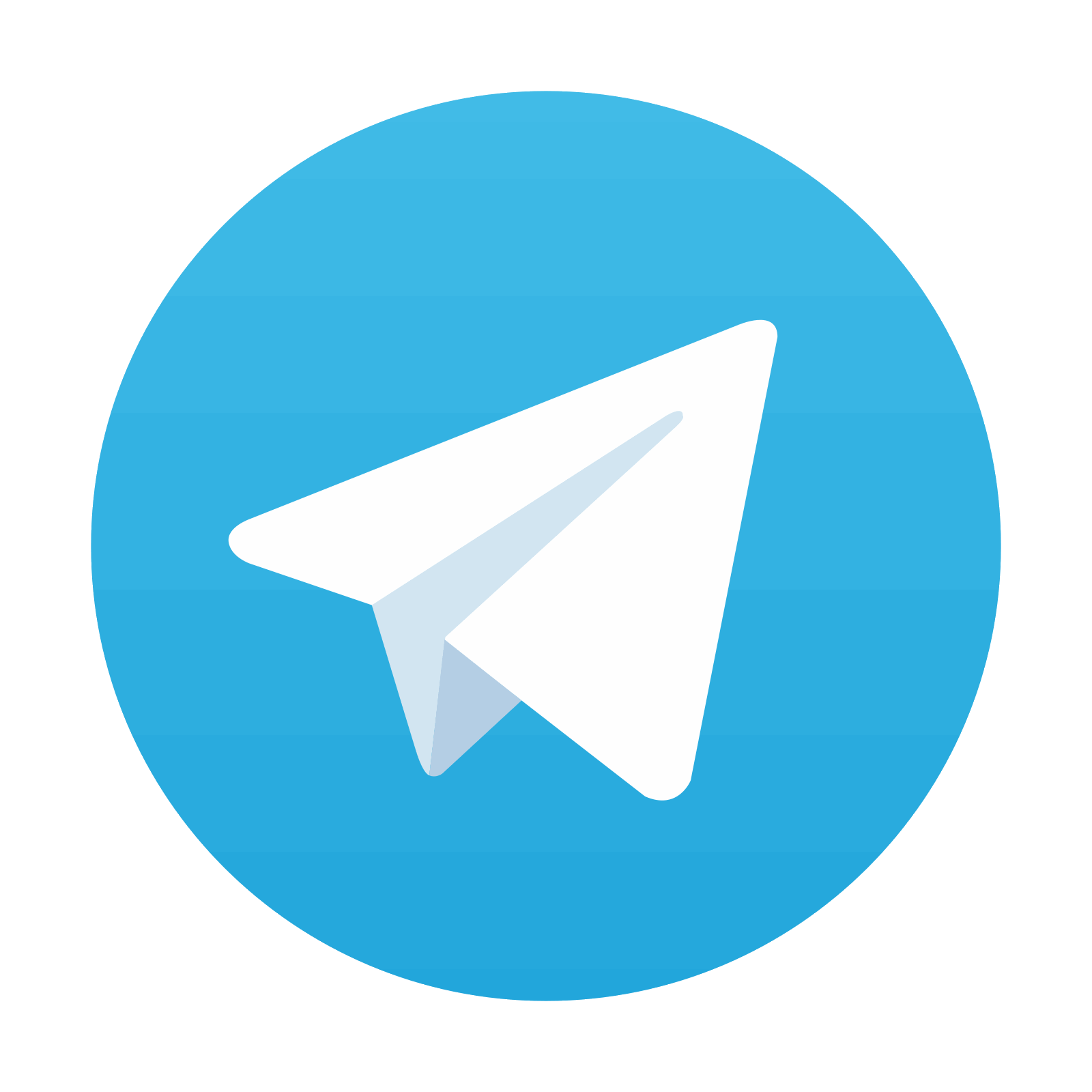
Stay updated, free articles. Join our Telegram channel

Full access? Get Clinical Tree
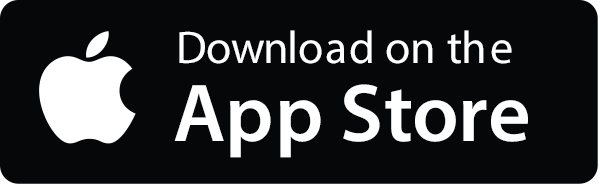
