F • O • U • R In order to provide leadership in evidence-based practice, advanced practice registered nurses (APRNs) require skills in the analytic methods that are used to identify population trends and evaluate outcomes and systems of care (American Association of Colleges of Nursing [AACN], 2006). APRNs need to be able to carry out studies with strong designs and solid methodology, taking into account the factors that can affect study results. This chapter discusses the complexities of data collection and the strengths and weaknesses of study designs used in population research. Critical components of data analysis are discussed, including bias, causality, confounding, and interaction. A dilemma that may occur with population research is the difficulty of controlling for variables that are not being studied but that may have an impact on the results. Finding a statistical association between an intervention and an outcome or an exposure and a particular disease is meaningful only if variables are correctly controlled, tested, and measured. The purpose of a well-designed study is to properly identify the impact of the variable (or variables) under study and to avoid bias and/or design flaws caused by another, unmeasured variable. Statistics are used to analyze population characteristics by inference from sampling (Statistics, 2002, p. 1695). They help us to translate and understand data. Before we can begin to understand a measured difference between groups, we have to identify the variation. But statistical analysis cannot overcome problems caused by a flawed study. When a researcher draws the wrong conclusion because of a problem with the research methodology, the result is a type I or type II error, also referred to as errors of inference. A type I error occurs when a null hypothesis is rejected when in fact it is true. A type II error occurs when one fails to reject a null hypothesis when in fact it is false. Take, for example, an APRN who carries out a study to determine whether a particular intervention improves medication compliance in hypertensive patients. To keep the example simple, the intervention will simply be referred to as “Intervention A.” A null hypothesis proposes no difference or relationship between interventions or treatments. In this case, the null hypothesis is: There is no difference in medication compliance between hypertensive patients who receive Intervention A and those who receive no intervention. Let us assume that the APRN completes the study and carries out the statistical analysis of the data. The following conclusions are possible: 1. There is no significant difference in medication compliance between the two groups. 2. There is a significant difference in medication compliance between the two groups. Now let us assume that the correct conclusion is number 1, but the APRN concludes that there is a difference in medication compliance between the two groups (rejects the null hypothesis when it is true). The APRN has committed a type I error. If the correct conclusion is number 2, but the APRN concludes that there is no difference in medication compliance between the two groups (fails to reject the null hypothesis when it is false), then a type II error has occurred (Table 4.1). When using data or working with data sets, it is critical to understand that mistakes can occur where measurements are involved. There are two basic forms of error of measurement: random error (also known as nondifferential error) and systematic error (also known as bias). Random errors occur as the result of the usual, everyday variations that are expected and that can be anticipated during certain situations. The result is a fluctuation in the measurement of a variable around a true value. Systematic errors occur not as the result of chance but because of inherent inaccuracies in measurement. They are typically constant or proportional to the true value. Systematic error is generally considered the more critical of the two. It can be the result of either a weak study design or a deliberate distortion of the truth. Random error measurements tend to be either too high or too low in about equal amounts because of random factors. Although all errors in measurement are serious, random errors are considered to be less serious than bias because they are less likely to distort findings. Random errors do, however, reduce the statistical power of a study and can occur because of unpredictable changes in an instrument used for collecting data or because of changes in the environment. For example, if one of three rooms being used to interview subjects became overheated occasionally during data collection, making the subjects uncomfortable, it could affect some of their responses. This effect in their responses is an example of a random error of measurement. TABLE 4.1 • Type I and Type II Errors
Epidemiological Methods and Measurements in Population-Based Nursing Practice: Part II
Patty A. Vitale and Ann L. Cupp Curley
ERRORS IN MEASUREMENT
Random Error
| Relationship Does Not Exist | Relationship Exists |
Conclude Relationship Does Not Exist (Fail to reject Null Hypothesis) | Correct Decision | Type II Error (β) |
Conclude Relationship Exists (Reject Null Hypothesis) | Type I Error (α) | Correct Decision Power (1 – β) |
Systematic Error
There are several types of systematic error or bias and all of them can impact the validity of study results. Bias can occur in many ways and is commonly broken down into 2 categories: selection and information bias. Such things as how the study design is selected, how subjects are selected, how information is collected, how the study is carried out (the conduct), or how the study is interpreted by investigators are all forms of potential bias. These problems can result in a deviation from the truth, which can lead to false conclusions.
Selection Bias
Selection bias occurs when the selected subjects in a sample are not representative of the population of interest or representative of the comparison group, and as a result, this selection of subjects can make it appear (falsely) that there is or is not an association between an exposure and an outcome. Selection bias is not simply an error in the selection of subjects for a study, but rather the systematic error that occurs with “selecting a study group or groups within the study” (Gordis, 2014, p. 263). Nonprobability sampling (nonrandom sampling) is strongly associated with selection bias. In nonprobability sampling, members of a target population do not share equal chances of being selected for the study or intervention/treatment group. This can occur with studies using convenience samples or volunteers. People who volunteer to participate in a study may have characteristics that are different from people who do not volunteer, and this can impact the outcome of the results and is simply referred to as volunteer bias. Similarly, people who do not respond to surveys may possess characteristics different from those who do respond to surveys. Thus, it is important to characterize nonresponders as much as possible, as the characteristics of responders may be very different from those of nonresponders and can lead to errors in survey interpretation. The best way to avoid this type of bias is to keep it at a minimum unless the characteristics of nonresponders can be identified and addressed. Another form of selection bias is exclusion bias, and this can occur when one applies different eligibility criteria to the cases and controls (Gordis, 2014). Withdrawal bias can occur when people of certain characteristics drop out of a group at a different rate than they do in another group or are lost to follow-up at a different rate. This can also lead to systematic error in the interpretation of data. APRNs must be aware of these types of error early in their study design. All of these types of systematic error can have an impact on how data are interpreted; therefore, minimizing these types of error through careful assessment of subject selection and eligibility criteria, and monitoring of characteristics in the populations of interest are critical for successful research and program implementation. Finally, probability sampling methods (random sampling) can be used to ensure that all members of a target population have an equal chance of being selected into a study, thereby eliminating the chance of selection bias (Shorten & Moorley, 2014).
Information Bias
Information bias deals with how information or data are collected for a study. This includes the source of data that are collected, such as hospital records, outpatient charts, or national databases. Many of these types of data are not collected for research purposes; so they may be incomplete, inaccurate, or contain information that is misleading. This can complicate data analysis as the information abstracted from these sources may be incorrect and can lead to invalid conclusions. Measurement bias is a form of information bias and occurs during data collection. It can be caused by an error in collecting information for an exposure or an outcome. Calibration errors can occur when using instruments to measure outcomes. This type of bias can also occur when an instrument is not sensitive enough to measure small differences between groups or when interventions are not applied equally (e.g., blood pressure measurements taken using the wrong cuff size). Information bias also includes how the data are recorded and classified. This can lead to misclassification bias, in which a control may be recorded as a case or a case is classified as having an exposure or exposures that he or she did not actually have. Misclassification bias can be subdivided into differential and nondifferential. For example, differential misclassification occurs when a case is misclassified into exposure groups more often than controls. In this case, this type of bias usually leads to the appearance of a greater association between exposure and the cases than one would find if this bias was not present (Gordis, 2014). In nondifferential misclassification, the misclassification occurs as a result of the data-collection methods such that a case is entered as a control or vice versa. In this situation, the association between exposure and outcome may be “diluted,” and one may conclude there is not an association when one really exists (Gordis, 2014). Another example of misclassification bias occurs when members of a control group are exposed to an intervention. This results in contamination bias. An example would be a nurse who floats from the floor where hourly rounding is being carried out to a control floor where no rounding is supposed to occur, but the nurse carries out hourly rounding on the control floor. In this case, contamination bias minimizes the true differences that would have been seen between groups. However, these cases should not be reassigned; in fact, any unexpected or unplanned crossover that occurs should be analyzed in the original group to which it was assigned by the investigator. This is known as the intent-to-treat principle. For example, patients who are assigned to one group or another and crossover intentionally or accidentally to the other group should be analyzed according to their original assignment. Intent to treat simply means that you assign patients to the original group you intended to treat them in from the start of the study regardless of the treatment they received.
If information is obtained from interviews, there can be bias introduced based on how the questions are asked or there may be variance between interviewers in how the questions are prompted to the subject. Recall bias happens when subjects are asked to remember or recall events from the past. For example, people who experience a traumatic event in their lives may recall events of that day more accurately and with more detail than someone asked to recall events from a day without significance. Reporting bias occurs when a subject may not report a certain exposure as he or she may be embarrassed or not want to disclose certain personal information, or the subject may report certain things to gain approval from the investigator (Gordis, 2014). The effects of bias can impact a study in two ways. It can make it appear that there is a significant effect when one does not exist (type I error), or there is an effect but the results suggest there is no effect (type II error) (see Table 4.1).
Finally, an APRN needs to be aware of publication bias (another type of information bias), particularly when carrying out systematic reviews or meta-analyses. Publication bias refers to the tendency of peer-reviewed journals to publish a higher percentage of studies with significant results than those studies with nonsignificant or negative statistical results. This problem has been identified and studied for decades, and there is evidence that the incidence of publication bias is on the increase (Joober, Schmitz, Annable, & Boksa, 2012). Song et al. (2009) completed a meta-analysis to determine the odds of publication by study results. Although they identified many problems that were inherent in studying publication bias (e.g., they pointed out that studies of publication bias may be as vulnerable as other studies to selective publication), they concluded that “[t]here is consistent empirical evidence that the publication of a study that exhibits significant or ‘important’ results is more likely to occur than the publication of a study that does not show such results” (p. 11). Among their recommendations was that all funded or approved studies should be registered and the results publicly available.
There are several issues related to publication bias. It can give readers a false impression about the impact of an intervention, it can lead to costly and futile research, it can distort the literature on a topic, and can be unethical. People who participate in research studies (subjects) are often told that their participation will lead to a greater understanding of a problem. There is a breach of faith when the results of these studies are not published and shared in the scientific community (Joober et al., 2012; Siddiqi, 2011). The publication of both categories of research is ethical and provides a more balanced and objective view of current evidence.
In summary, bias must be recognized and addressed early in the study design. Ultimately, bias should be avoided when possible, but if it is recognized, it should be acknowledged in the interpretation of results and addressed in the study discussion.
CONFOUNDING
Confounding occurs when it appears that a true association exists between an exposure and an outcome, but in reality, this association is confounded by another variable or exposure. An interesting study by Matsumoto, Ishikawa, and Kajii (2010) raised questions about the potential confounding effect of weather on differences found among communities in Japan. They investigated the rural–urban gap in stroke incidence and mortality by conducting a cohort study that included 4,849 men and 7,529 women in 12 communities. On average, subjects were followed for 10.7 years. Information on geographic characteristics (such as population density and altitude), demographic characteristics (including risk factors for stroke), and weather information (such as rainfall and temperature) were obtained and analyzed using logistic regression. The researchers discovered a significant association between living in a rural community and stroke, independent of risk factors. However, further analyses revealed that the actual link may be between the weather and stroke. They proposed that the difference seen in the incidence of stroke in these communities may be related not to living in a rural versus an urban community, but by the weather differences between communities. Low temperatures are known to cause an increase in coagulation factors and plasma lipids, and therefore, differences in weather could have an impact on the incidence of stroke. They cite the small number of communities as a limitation of the study, and for this reason they did not generalize their findings. But they did raise an important point: It is important to be aware of the many variables (e.g., biologic, environmental, etc.) that may confound a relationship in population studies (Matsumoto et al., 2010).
Identification of confounding or other causes of spurious associations are important in population studies. A confounder is a variable that is linked to both a causative factor or an exposure and the outcome. There are many examples of confounders, such as age, gender, and socioeconomic status. Confounding occurs when a study is performed, and it appears from the study results that an association exists between an exposure and an outcome, when, in fact, the association is actually between the confounder and the outcome. Another example of confounding might occur if an APRN carried out a study to determine whether there is a relationship between age and medication compliance without controlling for income. Younger, working patients might be more compliant not because of the age factor, but because they have the resources to buy their medications. If confounding is ignored, there can be long-term implications as the APRN may implement interventions for medication compliance with education programs aimed at older patients without considering problems related to income. The intervention would ultimately not succeed because the relationship is false or not causal due to confounding. By definition, confounders must be known risk factors for the outcome and must not be affected by the exposure or the outcome (Gordis, 2014). Confounding, although difficult to avoid, must be recognized and accounted for in studies.
There are some techniques that an APRN can use to reduce the effects of confounding variables. Random assignment to treatment and nontreatment groups can reduce confounding by ensuring each group has similar shared characteristics that otherwise might lead to spurious associations. In the earlier example, if you were concerned about the socioeconomic status and education level, you may stratify early on for those characteristics and randomly assign from each of those groups so that they are equally represented in your intervention and nonintervention groups. When random assignment is not possible, the matching of cases and controls for possible confounding variables can improve equal representation of subjects and can minimize the effect of confounding. Investigators can match groups or individuals. Group matching allows groups with similar characteristics of interest to be matched to each other. Each group should share a similar proportion of the characteristics of interest. Usually, cases should be selected first, and the control group should be selected with similar proportions of the characteristics of interest (Gordis, 2014).
In individual matching, each individual case is matched to a control with similar characteristics of interest. This is referred to as matched pairs. One has to be careful not to match cases and controls for too many characteristics, as it can be difficult to find a control or the control may be too similar to the case and true differences may not be able to be demonstrated in the analysis phase. Using strict inclusion and exclusion criteria also can be helpful and should be applied similarly for comparison groups. There are limitations to the latter two methods.
Although it is possible to match for known confounding variables, there may be other unknown confounding variables that cannot be controlled for and, if not recognized, can impact study conclusions. If the study groups are matched for gender, then gender cannot be evaluated in the final analysis. Additionally, if the study is matched for too many variables, this can also limit the study as all of the matched variables cannot be studied, and this may limit the ability to make valid conclusions. There is a similar problem with inclusion and exclusion criteria as both criteria should be applied the same to each of the study groups. The method for analyzing data can also help reduce problems related to confounding.
Multivariable regression, for example, can measure the effects of multiple confounding variables. This method is useful only when the variables are recognized and acknowledged. Recognition of confounders requires a basic understanding of the relationship between an exposure and a disease or an outcome, and can also be identified by performing a stratified analysis first. Once confounders are determined, then these variables can be added in and removed from the model one at a time. Interaction needs to be assessed, and the exposure–disease relationship is determined. These inferential methods estimate the contribution of each variable to the outcome while holding all other variables constant in the model. The objective is to include a set of variables that are theoretically or actually correlated with both the intervention and the outcome to reduce the bias of treatment effect. Therefore, the goal of regression analysis is to identify causal relationships by recognizing the confounders to ensure found relationships are real and not spurious (Kellar & Kelvin, 2013; Starks, Diehr, & Curtis, 2009).
INTERACTION
Whenever two or more factors or exposures are being studied simultaneously, the possibility of interaction exists. Interaction occurs when one factor impacts another such that one sees a greater or lesser effect than would be expected by one factor alone. Synergism occurs when the combined effect of two or more factors is greater than the sum of the individual effects of each factor. And conversely, the opposite or negative impact can be seen with antagonism of factors. One example of antagonism is seen with the interaction of exercise and diet. The combination of these two factors can actually reduce the risk of heart disease more than each factor alone. Synergistic models can have an additive effect in which the effect of one factor or exposure is added to another or can have a multiplicative effect in which the effect of one factor multiples the effect of another factor. For example, epidemiologists identified an interactive effect between cigarettes and alcohol; these two factors together have a multiplicative effect on the risk of developing digestive cancers (Sjödahl et al., 2007). There are many synergistic effects that can be found in clinical practice, especially as they pertain to drugs. First-generation antihistamines, such as chlorpheniramine, have a synergistic effect on opioids such as codeine. Patients are warned not to take them in combination as the sedative effects are more significant when taken together. APRNs who carry out investigations need to be aware of the potential interactions when examining the effects of multiple exposures on an outcome. A discussion on how to determine whether a model is multiplicative or additive can be found in more detail in an advanced epidemiologic textbook, but a basic understanding is necessary for interpreting the different outcomes that can occur from multiple exposures.
There are clearly many sources of error that can occur while conducting a study. The informed APRN needs to identify and acknowledge these types of errors and work to minimize them. Therefore, it is essential that APRNs recognize when errors occur and how they can impact a study, and should be familiar with measures that can be taken to avoid or minimize errors.
RANDOMIZATION
Randomized controlled trials (RCTs) are considered inherently strong because of their rigorous design. Random selection of a sample and random assignment to groups are objective methods that can be used to prevent bias and produce comparable groups. Random assignment helps to minimize bias by ensuring that every subject has an equal chance of being selected and that results are more likely to be attributed to the intervention being tested and not to some other extraneous factor such as how subjects were assigned to the treatment or control group. It is impossible to know all of the characteristics that could influence results. The random assignment of subjects to different treatment groups helps to ensure that study groups are similar in the characteristics that might affect results (e.g., age, gender, ethnicity, and general health). As a general rule, the greater the number of subjects that are chosen and assigned into the treatment or nontreatment groups, the more likely that the groups will be similar for important characteristics (Shott, 1990).
Blinding
Another problem encountered in research occurs when investigators or subjects themselves have an effect on study results. This can happen when a researcher’s personal beliefs or expectations of subjects can influence his or her interpretation of the outcome. Sometimes observers can err in measuring data toward what is expected. If subjects know or believe that they are given a placebo or the nonexperimental treatment, it may cause them to exaggerate symptoms that they would dismiss if given the experimental treatment. These actions by both investigators and subjects are not necessarily intentional; they can occur subconsciously.
DATA COLLECTION
As mentioned earlier, how data are collected and analyzed can lead to bias when conducting a study. The training of investigators to ensure that data are collected uniformly from all subjects and the use of a strict methodology for data collection and analysis contribute to a strong study design. Objective criteria should be used for the collection of all data. Strict inclusion and exclusion criteria should be developed in writing so that there are no questions as to what criteria are to be applied to the study. Avoiding subjective criteria is important as it can lead to inconsistent application of criteria. For example, if you chose “ill appearance” as an exclusion criterion, it may be difficult to apply this criterion uniformly as each APRN may have different levels of experience making this assessment. Objective criteria, such as heart rate greater than 120 beats per minute, respiratory rate greater than 24 breaths per minute, or oxygen saturations less than 90%, are easy to apply uniformly. Of course, even those criteria can be incorrectly assessed by someone who is inexperienced; however, one can see that these types of data are more easily reproducible within and between studies. One way to assess reliability between raters in a study is by using the kappa statistic. This statistic tests how reliable different investigators or data collectors are in their assessment or interpretation of data beyond what one would expect by chance alone.
If you were to evaluate two observers without any training, you would expect them to agree a certain percentage of the time and that percentage represents the chance of agreement, usually around 50%. Using the kappa statistic, you can estimate how reliable this agreement is by subtracting out the percentage expected by chance alone. Kappa values that are greater than 0.75 represent excellent agreement, and those values less than 0.40 represent poor agreement. These values, although not perfect, can give an investigator an assessment of how well her or his observers are agreeing with each other in their data interpretation (Landis & Koch, 1977). The kappa statistic appears frequently in the literature, and the APRN should be familiar with its use and limitations (Maclure & Willett, 1987).
One of the first steps in data analysis is to compare the demographic information of each of the groups studied to ensure that they are matched for important characteristics and that they represent the population of interest. Frequencies of data can be generated and compared for similarities and differences. This should be done early on so that any imbalance between groups is addressed before it becomes a problem in the analysis stage. This reiterates the importance of generating strict inclusion and exclusion criteria that can be followed with minimal error.
CAUSALITY
Ernst Mach, an Austrian professor of physics and mathematics and a philosopher, argued that all knowledge is based on sensation and that all scientific measurements are dependent on the observer’s perception. He proposed that “in nature there is no cause and effect” (Hutteman, 2013, p. 102). This is a relevant quote to begin a discussion of causality, because causality is a complex issue faced by all investigators. A single clinical disease can have many different “causes,” and one cause can have several clinical consequences. Causality becomes even more complex when we begin to look at chronic diseases. Chronic diseases can have multiple etiologies. Cardiac disease, for example, has multiple causes such as genetic predisposition, obesity, smoking, lack of exercise, poor diet, or any combination of these factors.
A useful definition of causation for population research is that an increase in the causal factor or exposure causes an increase in the outcome of interest (e.g., disease). With that said, if an association is found between an exposure and an outcome, then the next question is: Is it causal? There are many theories of causation, some of which have been addressed in Chapter 3, but no one theory can explain entirely the complex interactions of an exposure with the development of disease or an outcome.
There are multiple criteria that can help determine causality. No one criterion in and of itself determines causality, but each one may help strengthen the argument for or against causality. One important criterion is the determination of a statistical strength of association. Statistics are used to test hypotheses: Is an exposure or risk factor present significantly more often in a population with the disease than without? If a new intervention is put into place, is there a significant improvement in the targeted outcome? The strength of association is measured by such things as relative risk and attributable risk. Another criterion is the confirmation of a temporal relationship: The suspected exposure or risk factor needs to occur before the disease or outcome. For example, a person needs to smoke before he or she develops lung cancer in order to attribute lung cancer to smoking as a potential causal agent. To show a causal relationship requires the elimination of all known alternative explanations and an experienced investigator will seek out other potential explanations to explain why such a relationship may not exist (Katz, Elmore, Wild, & Lucan, 2013).
Two additional important considerations are scientific plausibility and the ability to replicate findings. Scientific plausibility refers to coherence with our current body of knowledge as it relates to the phenomenon under study. That is, do the results make sense based on what we know about the phenomenon? For example, is it biologically plausible that exposure to cigarette smoke (e.g., benzene, nicotine, tar) could convert normal cells into cancer cells? Additionally, the ability to replicate findings in different studies and in different populations provides strong evidence that a causal association exists. Other criteria for causation include the dose–response relationship. For example, with increasing exposure (e.g., smoking), one can see increasing risk of disease (e.g., lung cancer). Similarly, if one has a cessation of exposure, one would expect a cessation or reduction of disease. Finally, another criterion worth mentioning is consistency with other knowledge. This criterion takes into consideration knowledge of other known factors (e.g., environmental changes, product sales, behavioral changes) that may indicate a causal relationship. For example, if a law is passed that prohibits smoking in public places, it may result in fewer cases of smoking-related diseases reported in area hospitals. These criteria, in concert with a strong study design and methodology, can assist an APRN in determining the likelihood of causality when an association is found between an exposure and an outcome (Gordis, 2014).
Causes can be both direct and indirect. An example of a direct cause would be an infectious agent that causes a disease. Pertussis (whooping cough, a bacterial infection) is caused by Bordetella pertussis. The disease is a direct cause of the organism. Toxic shock syndrome is an example of an indirect cause. Although the staphylococcal organism and its toxins are the direct cause of the syndrome, the indirect cause (and the first factor that was identified) is tampons.
Even the infectious disease process is not simple. Both the host and the environment can have an impact on the infectious disease process. Characteristics of the host (e.g., age, previous exposures, general health, and immune status) can influence the development of the disease. Environmental conditions also play a role. A good example is influenza, which is most prevalent during certain times of the year. Infectious disease departments document these seasonal trends during the year, and they are available for hospitals to review. Such information can assist in antibiotic selection, hospital staffing, and educational campaigns to ensure immunizations or prevention programs are put into place. Awareness of seasonal fluctuations in certain diseases, trends in drug resistance, or changes in the community that affect the overall management of a patient are important in an APRN’s practice. By following these trends, the APRN can better assess the needs of the community and ensure that appropriate resources are available to address the fluctuations that occur naturally in all communities.
SCIENTIFIC MISCONDUCT (FRAUD)
Scientific misconduct includes (but is not limited to) gift authorship, data fabrication and falsification, plagiarism, and conflict of interest. It can have an impact on researchers, patients, and populations (Karcz & Papadakos, 2011). No one wants to believe that there are investigators who commit fraud by deliberately distorting research findings, but it does happen. Unfortunately, in some cases, the fraud is intentional; in other cases it occurs via a series of missteps from methodology to analysis. As mentioned earlier, multiple forms of bias or confounding can be introduced into a study, and these, if ignored, can lead to spurious results. Intentionally ignoring these issues, especially without addressing them as a limitation, can be fraudulent. Acceptance for publication in a prominent peer-reviewed journal and/or evidence that the protocol was approved by an institutional review board (IRB) does not ensure the accuracy and/or ethical conduct of that research.
Perhaps one of the most infamous cases of fraud involved a well-respected peer-reviewed journal. In 1998, The Lancet published an article written by Andrew Wakefield and 12 others that implied a link between the measles–mumps–rubella (MMR) vaccine and autism and Crohn’s disease. Although epidemiologists pointed out several study weaknesses, including a small number of cases, no controls, and reliance on parental recall, it received wide notice in the popular press. It was 7 years before a journalist uncovered the fact that Wakefield altered facts to support his claim and exploited the MMR scare for financial gain. The Lancet retracted the paper in 2010 (Godlee, Smith, & Marcovitch, 2011). A series of articles in the British Medical Journal (Deer, 2011) revealed how Wakefield and his associates distorted data for financial gain. Before this article was retracted, it caused widespread fear among parents and accelerated an antivaccine movement that many blame for the resurgence of infectious diseases among children.
In 2006, a writer for The New York Times (Interlandi, 2006) wrote an article that described a case of fraud that involved a formerly tenured professor at the University of Vermont. Dr. Eric Poehlman was tried in a federal court and found guilty. He was sentenced to 1 year and 1 day in jail for fraudulent actions that spanned 10 years. His misconduct included using fraudulent data in lectures and in published papers, and using these data to obtain millions of dollars in federal grants from the National Institutes of Health (NIH). He pleaded guilty to fabricating data on obesity, menopause, and aging. Interlandi’s article, which includes a very detailed account of Dr. Poehlman’s actions and his downfall, documents how a “committed cheater can elude detection for years by playing on the trust—and self-interest—of his or her junior colleagues” (p. 3).
It is safe to say that the majority of researchers carry out their research with scrupulous attention to detail and with integrity, but APRNs need to be aware that instances such as those mentioned earlier do happen. As stated in Chapter 3, it is important that when an APRN is making decisions related to population-based evaluation, the decisions need to be based on a sound methodological framework that includes ethical considerations of the effect of the research on the population as a whole. It is also important that APRNs are aware that fraud occurs in research and that they should be vigilant not only in how they carry out research but also in how they critically review the results of studies by other investigators.
STUDY DESIGNS
There is no perfect study design; however, there are strategies that can be used to decrease the threat of bias and increase the likelihood that hypotheses are answered accurately. The awareness that bias and confounding can cause a threat to the validity of study results is important and may be unavoidable; however, recognizing these limitations and addressing them within your study is even more critical. The design of high-quality and transparent studies creates a good foundation for evidence-based practice. Table 4.2 outlines the strengths and weaknesses of study designs used in population research.
Randomized Controlled Trials
When carefully designed, RCTs can provide the strongest evidence for the effectiveness of a treatment or intervention. Subjects are randomly assigned to either the intervention group (which will receive the experimental treatment or intervention) or the control group (which will receive the nonexperimental treatment or no intervention). Inclusion and exclusion criteria for the participants must be precise and spelled out in advance.
RCTs are considered strong designs because of their ability to minimize bias; however, if the randomization is not executed in a truly random manner, then the design can be flawed, or if the data are not reported consistently, then errors can lead to invalid conclusions. Consolidated Standards of Reporting Trials (CONSORT) is a method that has been developed to improve the quality and reporting of RCTs. It offers a standard way for authors to prepare reports of trial findings, facilitate their complete and transparent reporting, and aid their critical appraisal and interpretation (CONSORT Group, 2014). The CONSORT checklist items focus on reporting how the trial was designed, analyzed, and interpreted; the flow diagram displays the progress of all participants through the trial (CONSORT Group, 2014). The CONSORT guidelines are endorsed by many professional journals and editorial organizations. They are part of an effort to improve the quality and reporting of research that is conducted to make better clinical decisions. Both the CONSORT checklist and the CONSORT flow diagram can be accessed at www.consort-statement.org/consort-2010.
TABLE 4.2 • Strengths and Weaknesses of Study Designs
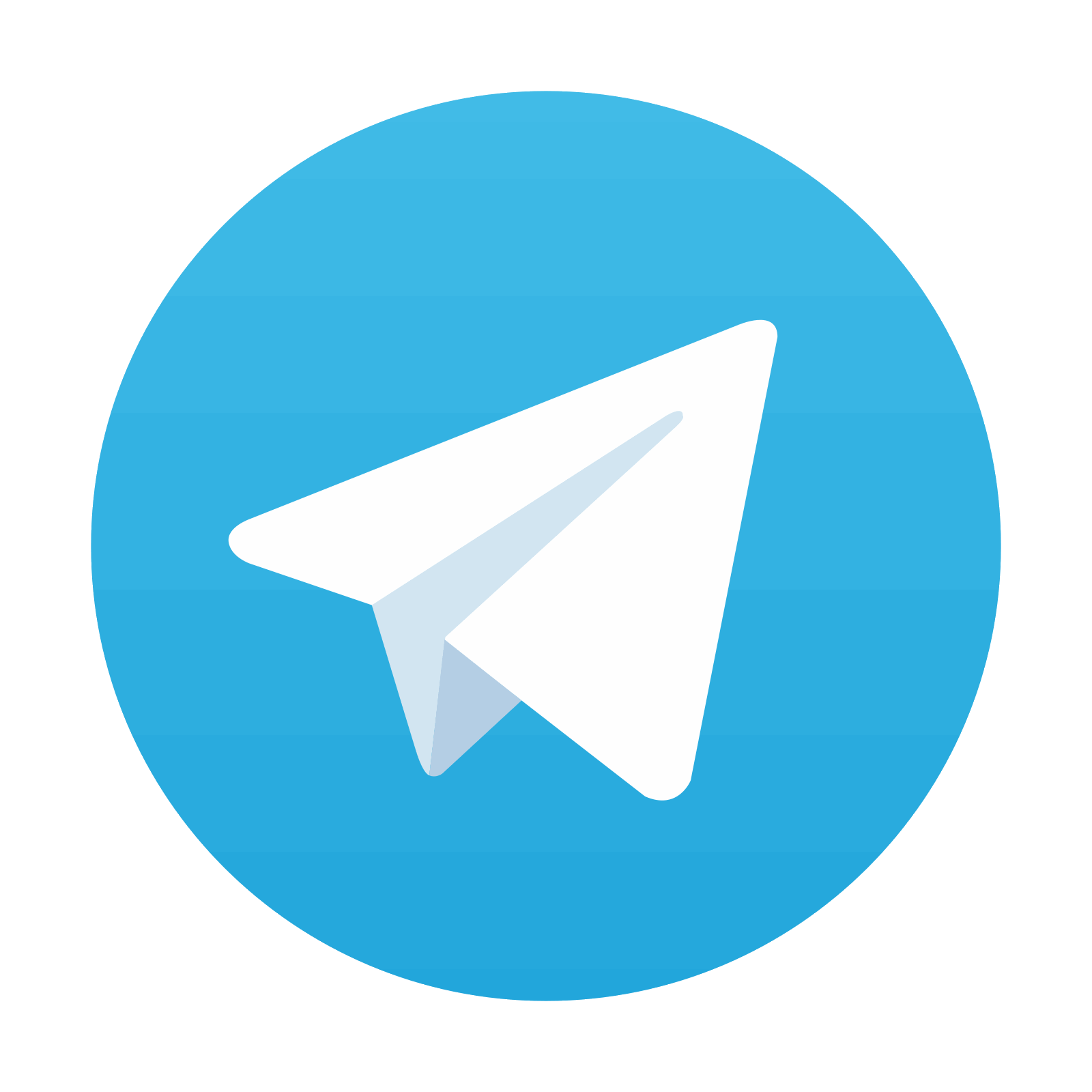
Stay updated, free articles. Join our Telegram channel

Full access? Get Clinical Tree
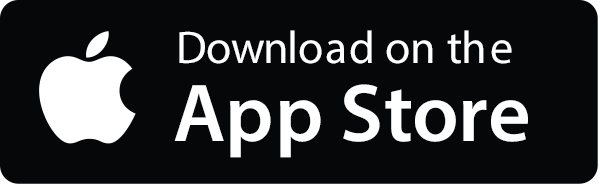
