T • H • R • E • E
Epidemiological Methods and Measurements in Population-Based Nursing Practice: Part I
Patty A. Vitale and Ann L. Cupp Curley
Evidence-based practice as it relates to population-based nursing combines clinical practice and public health through the use of population health sciences in clinical practice (Heller & Page, 2002). Epidemiology is the science of public health. It is concerned with the study of the factors determining and influencing the frequency and distribution of disease, injury, and other health-related events and their causes (Gordis, 2014). In addition to epidemiology, an understanding of other scientific disciplines, such as biology and biostatistics, is also important for identifying associations and determining causation when looking at exposures and outcomes as they relate to population health.
Population-based care focuses on populations at risk, analysis of aggregate data, evaluation of demographic factors, and recognition of health disparities. It is concerned with the patterns of delivery of care and outcome measurements at the population or subpopulation level. The purpose of this chapter is to provide readers with an understanding of the natural history of disease and the approaches that are integral for the prevention of disease. We introduce basic concepts that are necessary to understand how to measure disease outcomes and select study designs that are best suited for population-based research. Emphasis is placed on measuring disease occurrence with a fundamental discussion of how to calculate incidence, prevalence, and mortality rates. Successful advanced practice nursing in population health depends on the ability to recognize the difference between the individual and population approaches to the collection and use of data, and the ability to assess needs and evaluate outcomes at the population level. Concepts surrounding survival data are also discussed along with strategies to guide advanced practice registered nurses (APRNs) on how to calculate and interpret survival data.
THE NATURAL HISTORY OF DISEASE
The natural history of disease refers to the progression of a disease from its preclinical state (prior to symptoms) to its clinical state (from onset of symptoms to cure, control, disability, or death). Disease is not something that occurs suddenly, but rather, it is a multifactorial process that is dynamic and occurs over time. It evolves and changes and is sometimes initiated by events that take place years, even decades, before symptoms first appear. Many diseases have a natural life history that can extend over a very long period of time. The natural history of disease is described in stages. Understanding the different stages allows for a better understanding of the approach to the prevention and control of disease.
Stage of Susceptibility
The stage of susceptibility refers to the time prior to disease development. In the presence of certain risk factors, genetics, or environment, disease may develop and the severity can vary among individuals. Risk factors are those factors that are associated with an increased likelihood of disease developing over time. The idea that individuals could modify “risk factors” tied to heart disease, stroke, and other diseases is one of the key findings of the Framingham Heart Study (National Heart, Lung, and Blood Institute and Boston University, 2010). Started in 1948 and still in operation, this study is one of the most important population studies ever carried out in the United States. Before Framingham, for example, most healthcare providers believed that atherosclerosis was an inevitable part of the aging process. Although not all risk factors are amenable to change (e.g., genetic factors), the identification of risk factors is important and fundamental to disease prevention.
Preclinical Stage of Disease
During the preclinical phase, the disease process has begun but there are no obvious symptoms. Although there is no clear manifestation of disease, because of the interaction of biological factors, changes have started to occur. During this stage, however, the changes are not always detectable. Screening technologies have been developed to detect the presence of some diseases before clinical symptoms appear. The Papanicolaou (Pap) smear is an example of an effective screening method for detecting cancer in a premalignant state to improve mortality related to cervical cancer. The use of the Pap smear as a screening tool facilitates early detection and treatment of premalignant changes of the cervix prior to development of malignancy.
Clinical Stage of Disease
In the clinical stage of disease, sufficient physiologic and/or functional changes occur, leading to the development of recognizable symptoms of disease. It might also be accurately referred to as the treatment stage. For some people, the disease may completely resolve (either spontaneously or with medical intervention), and for some it will lead to disability and/or death. It is for this reason that the clinical stage of disease is sometimes subdivided for better medical management. Staging systems used in malignancies to better define the extent of disease involvement are an example of a system that can help guide the type of treatment modality selected based on stage. In many cases, staging can provide an estimate of prognosis. Another example is the identification of disability as a specific subcategory of the treatment stage. Disability occurs when a clinical disease leaves a person either temporarily or permanently disabled. When people become disabled, the goal of treatment is to mitigate the effects of disease and to help these individuals to function to their optimal abilities. This is very different from the goal for someone who can be treated and restored to the level of functioning that he or she enjoyed prior to the illness.
The Nonclinical Disease Stage
This nonclinical or unapparent disease stage can be broken into four subparts. The first subpart is the preclinical stage, which, as mentioned earlier, is the acquisition of disease prior to development of symptoms and is destined to become disease. The second subpart is the subclinical stage that occurs when someone has the disease but it is not destined to develop clinically. The third subpart is the chronic or persistent stage of disease, which is disease that persists over time. And finally, there is the fourth subpart or latent stage in which one has disease with no active multiplication of the biologic agent (Gordis, 2014).
The Iceberg Phenomenon
For most health problems, the number of identified cases is exceeded by the number of unidentified cases. This occurrence, referred to as the “iceberg phenomenon,” makes it difficult to assess the true burden of disease. Many diseases do not have obvious symptoms, as stated earlier, and may go unrecognized for many years. Unrecognized diseases, such as diabetes, hypertension, and mental illness, create a significant problem with identifying populations at risk and estimating service needs. Complications also arise when patients are not recognized or treated during an early stage of a disease when interventions are most effective. Additionally, patients who do not have symptoms or do not recognize their symptoms do not seek medical care and, in many cases, even if they do have a diagnosis, will not take their medications as they perceive that they are healthy when they are asymptomatic.
PREVENTION
Understanding the natural history of disease is as important as understanding the causal factors of disease because it provides the APRN with the knowledge that is required to design programs or interventions that target populations at risk. Understanding how disease develops is fundamental to the concept of prevention and provides a framework for disease prevention and control. The primary goal of prevention is to prevent disease before it occurs. The concept of prevention has evolved to include measures taken to interrupt or slow the progression of disease or to lessen its impact. There are three levels of prevention.
Primary Prevention
Primary prevention refers to the process of altering susceptibility or reducing exposure to susceptible individuals and includes general health promotion and specific measures designed to prevent disease prior to a person getting disease. Interventions designed for primary prevention are carried out during the stage of susceptibility and can include such things as providing immunizations to change a person’s susceptibility. Actions taken to prevent tobacco usage are another example of primary prevention. Tobacco use is one of the 12 leading health indicators used by Healthy People 2020 to measure health. Cigarette smoking is the leading cause of preventable morbidity and mortality in the United States (Centers for Disease Control and Prevention [CDC], 2014a), and prevention or cessation of smoking can reduce the development of many smoking-related diseases. Taxes on cigarettes, education programs, and support groups to help people stop smoking and the creation of smoke-free zones are all examples of primary prevention measures. The CDC linked a series of tobacco control efforts by Minnesota to a decrease in adult smoking prevalence rates. From 1999 to 2010, Minnesota implemented a series of antismoking initiatives, including a statewide smoke-free law, cigarette tax increases, media campaigns, and statewide cessation efforts. Adult smoking prevalence decreased from 22.1% in 1999 to 16.1% in 2010 (CDC, 2011). This is an excellent example of a statewide primary prevention effort to reduce smoking prevalence through a variety of initiatives.
Secondary Prevention
The early detection and prompt treatment of a disease at the earliest possible stage are referred to as secondary prevention. The goals of secondary prevention are to either identify and cure a disease at a very early stage or slow its progression to prevent complications and limit disability. Secondary prevention measures are carried out during the preclinical or presymptomatic stage of disease. Screening programs are designed to detect specific diseases in their early stages while they are curable and to prevent or reduce morbidity and mortality related to a later diagnosis of disease. Examples of secondary prevention include the Pap smear, mentioned earlier, as well as annual testing of cholesterol levels, mammography, and rapid HIV testing of asymptomatic individuals.
Tertiary Prevention
Tertiary prevention strategies are implemented during the middle or late stages of clinical disease and refer to measures taken to alleviate disability and restore effective functioning. Attempts are made to slow the progression or to cure the disease. In cases in which permanent changes have taken place, interventions are planned and designed to help people lead a productive and satisfying life by maximizing the use of remaining capabilities (rehabilitation). Cardiac rehabilitation programs that provide physical and occupational therapies to postoperative cardiac patients are an example of tertiary prevention.
CAUSATION
The Epidemiological Triangle
The relationship between risk factors and disease is complex. Research studies may describe a relationship between a risk factor and disease, but how do we know this relationship is causal? An understanding of causation is important if APRNs want to effectively impact the health of populations. The epidemiological triangle is a model that has historically been used to explain causation. The model consists of three interactive factors: the causative agent (those factors for which presence or absence cause disease—biologic, chemical, physical, nutritional), a susceptible host (such things as age, gender, race, immune status, genetics), and the environment (including such diverse elements as water, food, neighborhood, pollution). A change in the agent, host, and environmental balance can lead to disease (Harkness, 1995). The underlying assumptions of this model are that causative factors can be both intrinsic and extrinsic to the host and that the cause of disease is related to interaction among these three factors. This model initially was developed to explain the transmission of infectious diseases and was particularly useful when the focus of epidemiology was on acute diseases. It is less helpful for understanding and explaining the more complicated processes associated with chronic disease. With the rise of chronic diseases as the primary cause of morbidity and mortality, a model that recognizes multiple causative factors was needed to better understand this complex interaction.
The Web of Causation
The dynamic nature of chronic diseases calls for a more sophisticated model for explaining causation than the epidemiological triangle. Introduction of the web of causation concept first appeared in the 1960s when chronic diseases overtook infectious diseases as the leading cause of morbidity and mortality in the United States. The foundation of this concept is that disease develops as the result of many antecedent factors and not as a result of a single, isolated cause. Each factor is itself the result of a complex pattern of events that can be best perceived as interrelated in the complex configuration of a web. The use of a web is helpful for visualizing how difficult it is to untangle the many events that can precede the onset of a chronic illness.
Critics have argued that this model places too much emphasis on epidemiological methods and too little on theories of disease causation. As theories evolved about the relationship between smoking and cancer, the U.S. surgeon general appointed a committee to review the evidence. This committee developed a set of guidelines for judging whether an observed association is causal. These guidelines include temporal relationship, strength of the association, dose–response relationship, replication of the findings, biologic plausibility, consideration of alternate explanation, cessation of exposure, consistency with other knowledge, and specificity of the association (Gordis, 2014). For a more detailed discussion on this model, see Chapter 4.
METHODS OF ANALYSIS
Successful population-based approaches depend on the ability to recognize the difference between the collection and use of data from individuals and populations and the ability to assess needs and evaluate outcomes at the population level. Several of the more recent theories of causation can be helpful in determining whether an exposure is causally related to the development of disease. In particular, calculating the strength of association using statistics is one of several criteria that can be used to determine causality. However, statistics must be used with caution. Health is a multidimensional variable: factors that affect health, and that interact to affect health, are numerous. Many relationships are possible. There are problems inherent in the use of statistics to explain differences among groups. Although statistics can describe disparities, they cannot explain them. It is left to the researchers to explain the differences. In addition to statistics, one must also be aware of the validity and reliability of the data. There are problems associated with the categorizing and gathering of statistics during the research process that can have an effect on how the data should be interpreted. In order to be successful in research, one must do more than just collect data: One must look at the theoretical issues associated with explaining the relationship among the variables. Additionally, even if a relationship is found to be statistically significant, that does not assure that it is clinically significant. Recognizing limitations in research and in practice are the most important steps prior to making conclusions in any setting. Therefore, it is important that APRNs have a commitment to higher standards with an emphasis placed on adherence to careful and thorough procedural and ethical practice.
Methods derived from epidemiology can be useful in identifying the etiology or the cause of a disease. Among the important steps in this process are the identification of risk factors and their impact in a population, determining the extent of a disease and/or adverse events found in a population, and evaluating both existing and new preventive and therapeutic measures and modes of healthcare delivery. Applying strong epidemiologic methods with a sound application and interpretation of statistics are the foundation for evidence-based practice. The integration of evidence can lead to the creation of sound public policy and regulatory decisions.
Descriptive Epidemiology
Rates
Knowledge of how illness and injury are distributed within a population can provide valuable information on disease etiology and can lay the foundation for the introduction of new prevention programs. It is important to know how to measure disease in populations, and rates are a useful method for measuring attributes over time such as disease and injury in any population. Rates can also be used to identify trends and evaluate outcomes and can allow for comparisons within and between groups. The Morbidity and Mortality Weekly Report (MMWR; located at www.cdc.gov/mmwr) is a publication of the CDC and contains updated information on incidence and prevalence of many diseases and conditions. These rates provide healthcare providers with up-to-date information on the risks and burdens of various diseases and conditions (CDC, 2014b). The information obtained from the MMWR can be used to identify trends and provide policy makers with information for designating resources. The following is an example of how such information can be used:
Hypertension is a major risk factor for heart disease and stroke. Heart disease and stroke occur in approximately 30% of adults aged 18 years and older in the United States. A comparison of rates between 2007 and 2010 revealed that Hispanic Blacks had a higher rate of hypertension (41.3%) than non-Hispanic Whites (28.6%) and Hispanics (27.7%). Adults born in the United States had a higher rate of hypertension (30.6%) than non-U.S.-born adults (25.7%). Adults younger than 65 years with public insurance had a higher rate of hypertension (28.3%) than those with private insurance (20.0%) and those with no insurance (20.4%).
This excerpt is adapted from an issue of MMWR published on November 22, 2013 (Gillespie & Hurvitz, 2013). By publishing rates in percentages and comparing those rates among groups, it highlights both the burden of heart disease and stroke and the disparity between different demographic profiles related to hypertension. This information can be useful to both clinicians and policy makers who make decisions about interventions and services.
When calculating rates, the numerator is the number of events that occur during a specified period of time and is divided by the denominator, which is the average population at risk during that specified time period. This number is multiplied by a constant—either 100, 1,000, 10,000, or 100,000—and is expressed as per that number. The purpose of expressing rates as per 100,000, for example, is to have a constant denominator, and it allows investigators to compare rates among groups with different population sizes. Simply put, the rate is calculated as follows:
Rate = Numerator/denominator × Constant multiplier
In order to calculate rates, the APRN must first have a clear and explicit definition of the patient population and of the event. An important consideration when calculating rates is that anyone represented in the denominator must have the potential to enter the group in the numerator, and all persons represented in the numerator must come from the denominator.
Rates can be either crude or specific. Crude rates apply to an entire population without any reference to any characteristics of the individuals within it. For example, to calculate the crude mortality rate, the numerator is the total number of deaths during a specific period of time divided by the denominator, which is the average number of people in the population during that specified period of time (including those who have died). Typically, the population value for a 1-year period is determined using the midyear population.
In order to compare rates in two or more groups, the events in the numerator must be defined in the same way, the time intervals must be the same, and the constant multiplier must be the same. Rates can be used to compare two different groups, or one group during two different time periods. Returning to the example about breastfeeding, the breastfeeding rates could be compared in the same hospital, but at two different times, before and after implementation of a planned intervention to increase breastfeeding rates.
Formulas for the rates discussed in this chapter can be found at the end of the chapter (Exhibit 3.1).
Incidence and Prevalence
Incidence rates describe the occurrence of new events in a population over a period of time relative to the size of the population at risk. Prevalence rates describe the number of all cases of a specific disease or attribute in a population at a given point in time relative to the size of the population at risk. Incidence provides information about the rate at which new cases occur and is a measure of risk. For example, the formula for the incidence rate for HIV is:
Incidence rates provide us with a direct measure of how often new cases occur within a particular population and provide some basis on which to assess risk. By comparing incidence rates among population groups that vary in one or more risk factors, the APRN can begin to get some idea of the association between risk factors and disease. If in the earlier example of breastfeeding, the APRN discovers breastfeeding rates are significantly different among different ethnic groups, the characteristics of the groups can be compared and the causes for this disparity can be hypothesized and tested.
Period prevalence measures the number of cases of disease during a specific period of time and is a measure of burden. The formula for the period prevalence rate for HIV in 2015 is:
An example of how prevalence rates are used in the literature is as follows:
The CDC (2010, 2014c) revealed that in 2013, no state had a prevalence of obesity less than 20% (in 2009, 28 states had a prevalence of obesity less than 20%). Also in 2013, 18 states had a prevalence of obesity between 30% and 35% (in 2009, 9 states had prevalence rates over 30%). Regionally, the South had the highest prevalence of obesity (30.2%), followed by the Midwest (30.1%), the Northeast (26.5%), and the West (24.9%).
Information on the rising prevalence of adult obesity in the United States has led to increased attention to factors that cause obesity (especially in children). This has led to the development of new programs aimed at primary and secondary prevention.
Mortality Rates
Mortality rates, also known as death rates, can be useful when evaluating and comparing populations. As stated earlier, there are many factors that can affect the natural history of disease, and measuring mortality allows investigators to compare death rates among and within populations. The formula for mortality rate is:
Standardization of crude rates is an important consideration when comparing mortality rates among populations. Standardization is used to control for the effects of age and other characteristics in order to make valid comparisons between groups. Age adjustment is an example of rate standardization and perhaps the most important one. No other factor has a larger effect on mortality than age. Consider the problem of comparing two communities with very different age distributions. One community has a much higher mortality rate for colon cancer than the other, leading investigators to consider a possible environmental hazard in that community, when in fact, that community’s population is older, which could account for the higher mortality. Direct age adjustment or standardization allows a researcher to eliminate the age disparities between two populations by using a standardized population. This allows the researcher to compare mortality or death rates between groups by eliminating age differences between populations and comparing actual age-adjusted mortality rates to determine whether age truly plays a role in the crude unadjusted mortality rates.
There are two methods of age adjustment: direct, as mentioned earlier, and indirect. The direct method applies observed age-specific mortality or death rates to a standardized population. The indirect method applies the age-specific rates of a standardized population to the age distribution of an observed population and is used to determine whether one population has a greater mortality because of an occupational hazard or risk compared to the general population (to learn how to perform age adjustment, refer to an advanced epidemiology text).
The case fatality rate (CFR) is a measure of the severity of disease (such as infectious diseases) and can be helpful when designing programs to reduce the rate or disparity in the population. It should be noted that CFR is not a true rate as it has no explicit time implication but rather is a proportion of persons with disease who died from that disease after diagnosis. It is a measure of the probability of death among diagnosed cases. Its usefulness for chronic diseases is limited because the length of time from diagnosis to death can be long. The CFR is also useful in determining when to use a screening test. Screening tests identify disease early so that an intervention or treatment can be initiated in the hopes of lessening the morbidity or mortality of that disease. Those diseases that are rapidly fatal may not necessarily be beneficial to screen unless the screening will allow for a cure or treatment to change the overall outcome or to prevent unnecessary spread of the disease. Screening is useful in identifying disease in asymptomatic individuals in whom further transmission of disease can be prevented or reduced, such as in HIV. CFRs, therefore, can be helpful for comparisons between study populations and can provide useful information that could help determine whether an intervention or treatment is working. The formula for the CFR is as follows:
The CFR is usually expressed as a percentage; so in this case, one would multiply this rate by a constant multiplier of 100 to obtain the percentage of disease that is fatal. It is important in all of these rates to include those who have died from the disease in the denominator. Removing those who have died from the denominator falsely increases the CFR, making the disease appear more fatal or severe (Gordis, 2014).
The proportionate mortality ratio is useful for determining the leading causes of death. The formula for proportionate mortality ratio is as follows:
Again, this measure is usually reported as a percentage and reflects the burden of death due to a particular disease. This information is useful for policy makers who make decisions about the allocation of resources. (See Exhibit 3.1 for a list of these formulae.)
Survival and Prognosis
Mortality rates are very helpful when comparing groups and looking at disparities among populations. One cannot discuss mortality without having an understanding of survival and prognosis. Many diseases, particularly cancer, are studied over time, with attention placed on survival. Ideally, survival should be measured from the onset of disease until death, but the true onset of disease is generally unknown. Survival rates are usually calculated at various intervals from diagnosis or initiation of treatment. Prognosis is calculated using collected data to estimate the risk of dying or surviving after diagnosis or treatment begins. As mentioned earlier, case fatality rates give a good estimate of prognosis or severity of disease. However, they are best suited for acute diseases in which death occurs relatively soon after diagnosis. Survival analysis is better suited for chronic diseases or those diseases that take time to progress.
Survival time is generally calculated from the time of diagnosis or from the start of treatment. This can vary from patient to patient as some patients may seek care immediately after symptoms present or may wait months to seek care. Some patients are diagnosed prior to symptom presentation after they screened positive on a screening test. Some may obtain a diagnosis immediately, whereas others may have poor access to care and diagnosis is delayed by weeks to months or even years. Once a diagnosis is made, treatment may or may not occur immediately. Additionally, some patients may die before diagnosis or treatment. Because these individuals are not represented in survival analysis, this can lead to a falsely increased survival time. With that said, one can see how difficult it is to establish a true survival time after diagnosis. However, we can estimate survival if we use a common denominator and consistent criteria for measurement.
Before we discuss how to calculate and interpret survival data, we must touch on two important concepts, lead time bias and overdiagnosis bias. Lead time bias is a phenomenon whereby a patient is diagnosed earlier by screening and appears to have increased survival due to screening but rather dies at the same time he or she would regardless of screening. In other words, the time from which a patient is diagnosed earlier from screening is the lead time and the bias is the error that occurs as a result of concluding that screening leads to a longer survival after diagnosis. As can be seen in the following timeline, the survival time is longer when screening is implemented, but the ultimate time of death is unchanged. Although this is not true for all screening tests, it is important to recognize the phenomenon of lead time bias as it can affect the conclusions that are made regarding survival, which ultimately can affect a patient’s perceived prognosis.
Overdiagnosis bias occurs as a result of making a diagnosis from screening for a disease or cancer that would not have manifested clinically or has a slow progression, such that the person dies from another etiology. This type of bias has the potential to increase undue stress in individuals and can also falsely increase survival times, especially for diseases with slow progression. In both these types of biases, there is no difference in overall mortality in those screened versus those who were not screened. With that said, considerations must also be made for those screening tests in which a false-negative test reassures a patient who may not seek care and ultimately develops cancer and potentially has decreased survival due to delay in diagnosis. All of these biases need to be taken into consideration when interpreting survival data.
Prognosis is calculated using survival rates. There are two methods of conducting survival analysis and estimating prognosis that are discussed. The first is the actuarial method, which measures the likelihood of surviving after each year of treatment (or a predetermined interval). This is calculated as follows: the probability of surviving 2 years if one survived 1 year, or the probability of surviving 3 years if one survived 2 years, and so on. Prognosis is most commonly described in the literature as the probability of surviving 1, 2, 3, or more years. Generally, survival is calculated as a probability P1, P2, P3, and so on. The survival after 1 year is designated as P1; if patients survived 1 year after treatment, those who survived to 2 years = P2; if patients survived 2 years after treatment, those who survived to 3 years = P3; and so on. To calculate P1, divide the number of survivors over the number of patients with the disease at the start of the study or treatment. It is important to note that those who are lost to follow-up (also known as withdrawals) or who are no longer studied must be removed from the denominator. When a study ends or is terminated, those patients are no longer followed and must be taken into consideration in your analysis, and this is called censorship. For simplicity, the following examples will assume no losses to follow-up, but it is important to recognize that those who are lost to follow-up or those who are censored must be taken into account in your calculations. Of note, in a more advanced epidemiology textbook, you will find that those who are lost to follow-up will be subtracted out of the denominator and multiplied by ½ to account for the chance they were at risk for half the interval. Again for the purposes of this text, we will use a hypothetical example in which no patients are lost to follow-up. By definition, here is how to calculate P1, P2, and so on:
• P1 = (Number alive after 1 year of treatment)/(Number who started treatment)
• P2 = (Number alive after 2 years of treatment)/(Number who survived first year of treatment – Those who dropped out or were lost to follow-up)
• P3 = (Number alive after 3 years of treatment)/(Number who survived second year of treatment – Those who dropped out or were lost to follow-up)
To calculate the probability of surviving 1, 2, 3, or more years, the calculation is as follows:
P1 = probability of surviving 1 year
P1 × P2 = probability of surviving 2 years
P1 × P2 × P3 = probability of surviving 3 years
P1 × P2 × P3 × P4 = probability of surviving 4 years
P1 × P2 × P3 × P4 × P5 = probability of surviving 5 years
Using data from Table 3.1 we can calculate and interpret these probabilities.
In this example:
P1 = 88/100 = 0.88
P2 = 76/88 = 0.86
P3 = 55/76 = 0.72
P4 = 47/55 = 0.85
P5 = 33/47 = 0.70
Probability of surviving 1 year = 0.88
Probability of surviving 2 years = 0.88 × 0.86 = 0.76
Probability of surviving 3 years = 0.88 × 0.86 × 0.72 = 0.54
Probability of surviving 4 years = 0.88 × 0.86 × 0.72 × 0.85 = 0.46
Probability of surviving 5 years = 0.88 × 0.86 × 0.72 × 0.85 × 0.70 = 0.32
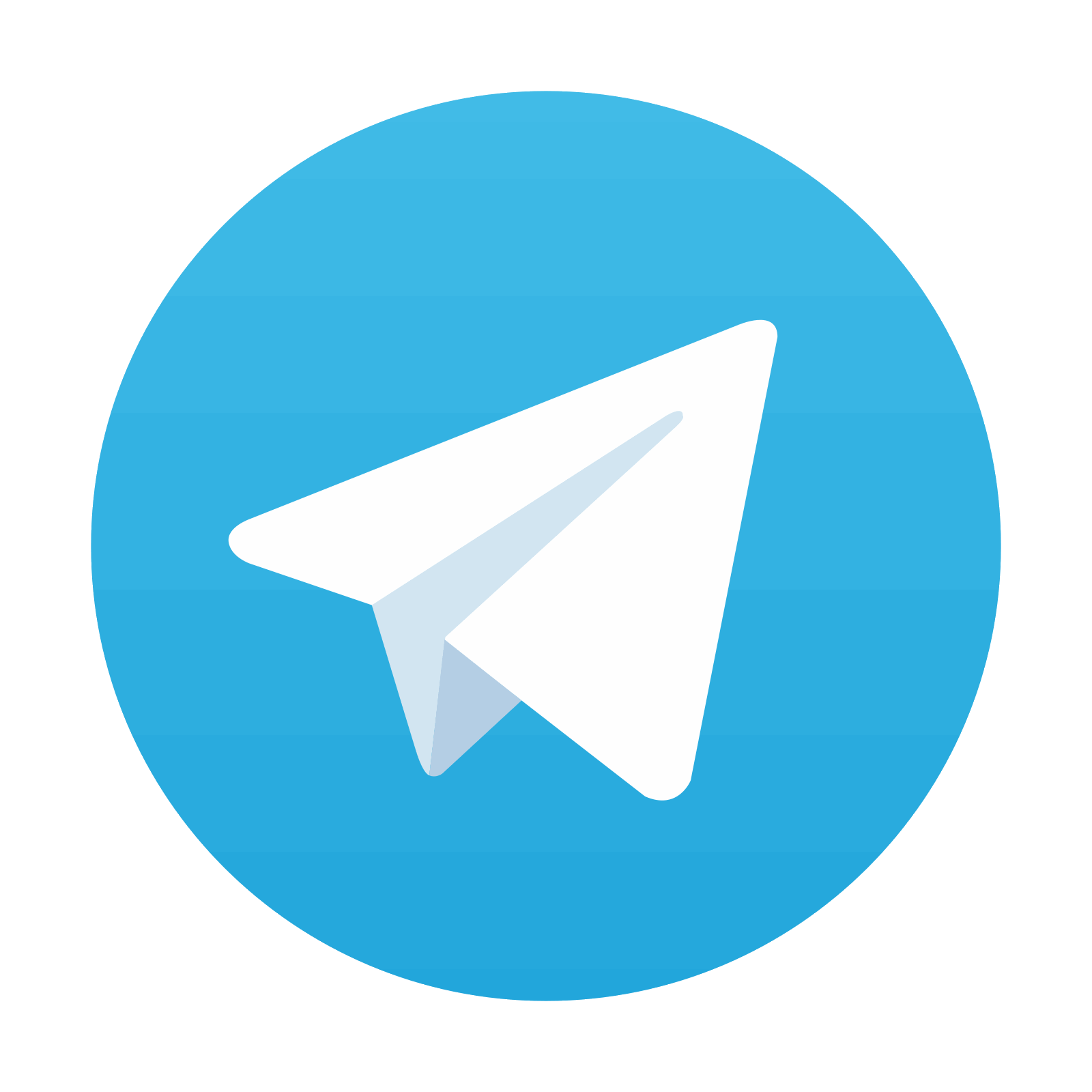
Stay updated, free articles. Join our Telegram channel

Full access? Get Clinical Tree
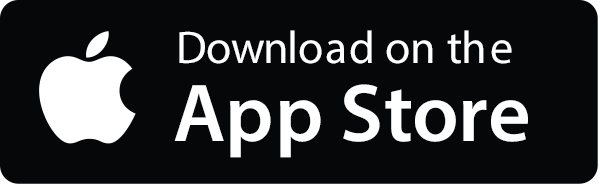
