21
Effect size and the interpretation of evidence
Effect size
Effect size expresses the size of the change or degree of association that can be attributed to a health intervention. The term effect size is also used more broadly in statistics to refer to the size of the phenomenon under study. For example, if we were studying gender effects on how long people live, a measure of effect size could be the difference in life expectancy between males and females. On average, this difference is actually around 5 years, which has real implications! In a correlational study, the effect size is the size of the correlation between the selected variables under study (e.g. r² as discussed in Chapter 18). There are many measures or indicators of effect size; the one which is relevant to analysing the results of a study is selected on the basis of the scaling of the outcome or dependent variable (Sackett et al 2000).
Effect size with continuous data
Study 1: Test–retest reliability of a force measurement machine
In this first study, the student was concerned with demonstrating the test–retest reliability of a device designed to measure maximum forces being produced by patients’ leg muscles under two conditions (flexion and extension). Twenty-one patients took part in the study and the reliability of the measurement process was tested by taking two readings for the same patients an hour apart and then calculating the Pearson correlation between the readings obtained from the machine in question during two trials separated by an hour for each patient. The results are shown in Table 21.1. Both results reached the p = 0.01 level of significance.
Study 2: A comparative study of improvement in two treatment groups
The second project was a comparative study of two groups: one group suffering from suspected repetition strain injuries (RSI) induced by frequent computer data entry and a group of ‘normals’. An activities of daily living (ADL) assessment scale was used and yielded a ‘disability’ index of between 0 and 50. There were 60 people in each group. The results are shown in Table 21.2.
where µ1−µ2 refers to the difference between the population means and σ refers to the population standard deviation.
where indicates the difference between the sample means and s1 refers to the standard deviation of the ‘normal’ or ‘control’ group. Therefore, for the above example, substituting into the equation yields:
In other words, the average ADL score of the people with suspected RSI was 2.33 standard deviations under the mean of the distribution of ‘normal’ scores. The meaning of d can be interpreted by using standardized scores. The greater the value of d, the greater the standardized difference between the means and therefore the larger the effect size.
When we say that the findings are clinically or practically significant we mean that the effect is sufficiently large to influence clinical practices. It is the health workers rather than statisticians who need to set the standards for each health and illness determinant or treatment outcome. After all, even relatively small changes can be of enormous value in the prevention and treatment of illnesses. There are many statistics currently in use for determining effect size. The selection, calculation and interpretation of various measures of effect are beyond our introductory book, but interested readers can refer to Sackett et al (2000).
Effect size with discontinuous data
As we discussed in Chapter 15, odds ratio (OR) compares the odds of the occurrence of an event for one group with the odds of the event for another group. For an RCT, the odds ratio compares the odds of an event for the intervention group with the odds for the control group. For example, the odds for each group, of having type 2 diabetes, in the hypothetical RCT of an exercise program for obese men, are given in Chapter 15. So, using these numbers, the odds ratio is:
This means that the risk of type 2 diabetes was reduced by 20% in the intervention (exercise) group.
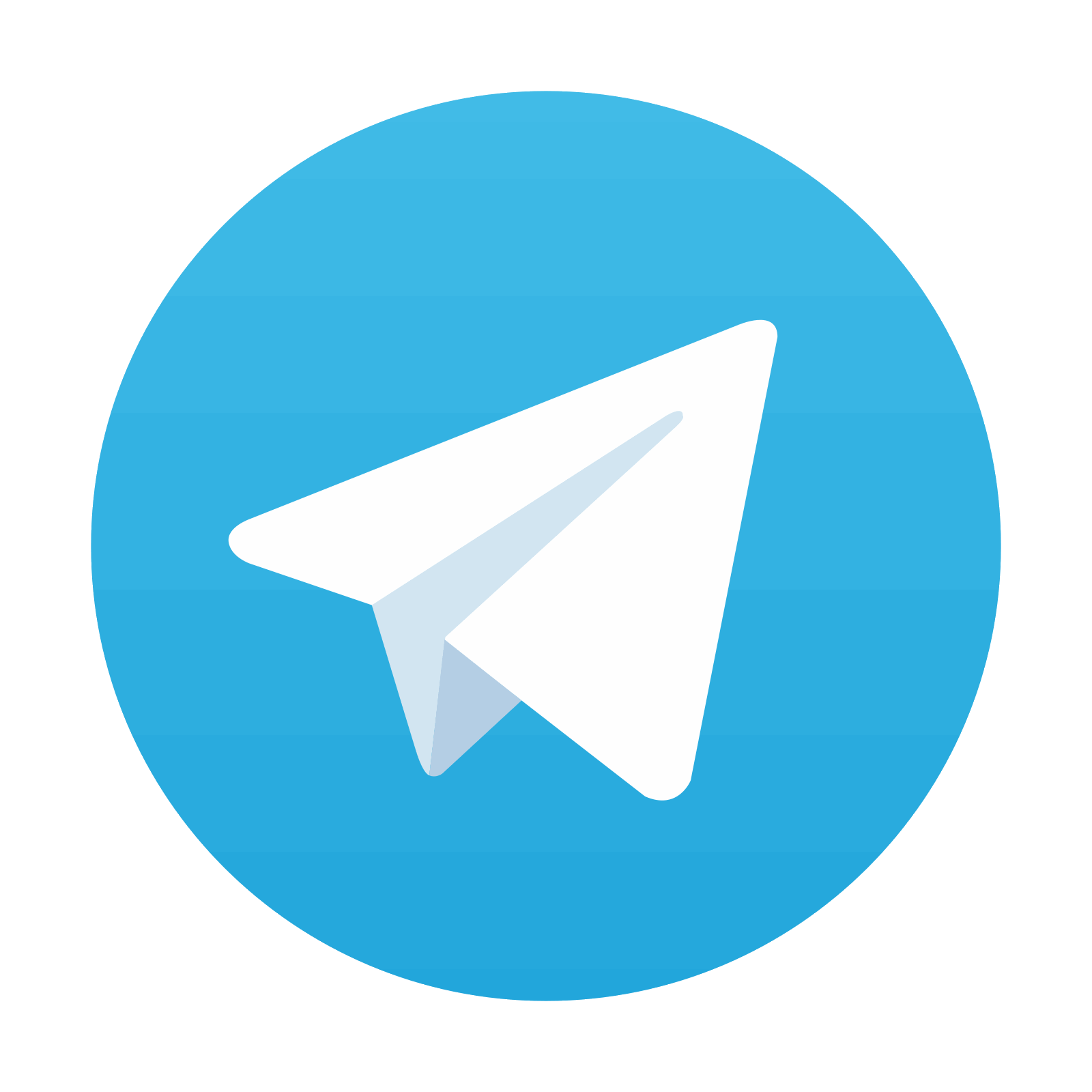
Stay updated, free articles. Join our Telegram channel

Full access? Get Clinical Tree
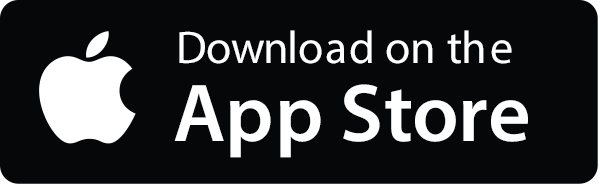
