Content validity index (CVI)
Not relevant
Item need some revision
Relevant but need minor revision
Very relevant
Not clear
Item need some revision
Clear but need minor revision
Very clear
Comment
Items
Relevancy
Clarity
1.
Item
Comment
2.
Item
Comment
The aggregated ratings can then be expressed on the item- (I-CVI) and scale-level (S-CVI). A good CVI score will indicate that the items are both understandable and cover the same content. The I-CVI is computed by dividing the number of experts who have scored the item as 3 (quite relevant) or 4 (highly relevant) by the total number of experts participating in the evaluation. For example, 11 (experts which scored an item 3–4)/12 (total number of experts) = 0.91666 = 0.92; this means that the I-CVI of the specific item is 0.92. The S-CVI is calculated by averaging the I-CVI values for all of the items included in the instrument. For example, if seven items have an I-CVI = 1.0 and three items have a I-CVI = 0.67, the S-CVI would be (1.0 × 7 + 0.67 × 3)/10 items = 0.901 = 0.90.
Items are deleted or modified according to the I-CVI and S-CVI results [11, 12]. Experts are also afforded the possibility to leave open comments about each item, for example, suggestions for how to modify the phrasing. If inter-rater agreement and/or the S-CVI score is lower than 0.70, which means that certain items will have to be deleted or modified, another round of expert evaluation is required. Furthermore, researchers should always pre-test their instrument before large-scale data collection. The purpose of the pre-test is to evaluate the practicality, understandability, and interpretations of the items, as well as assess how easily the participants can answer the questions and progress through the survey [13]. In some cases, the participants may also be asked to assess readability, questionnaire length, item wording, and clarity, as well as how time consuming the questionnaire is to answer [14].
8.1.1.3 Phase III: Psychometric Testing of the Instrument
After a developed instrument has been content validated, the instrument must also undergo a pilot test. The first version of the instrument should be pilot tested on a sample that was selected by random sampling and fit the inclusion criteria. Psychometric testing, during which various instrument properties such as reliability and validity are assessed, is performed to evaluate the quality of the instrument. Validity addresses the degree to which an instrument measures what it claims to measure [12, 15]. Construct validity can be assessed by analysing the pilot test data through exploratory factor analysis (EFA). The result of this analysis will indicate whether the instrument has good construct validity, i.e., the contents of all of the items correspond well to the concept that is being measured. Reliability, on the other hand, encompasses accuracy, consistency, and reproducibility, parameters which are measured by calculating Cronbach’s alpha values, item-total correlations, and inter-item correlations [12, 15].
After the statistical tests, the content of items with low factor loadings, cross-loading, and communalities is evaluated by a panel of experts, who provide feedback via a structured questionnaire. The expert panellists’ evaluations are once more assessed by calculating CVI. Certain items will be deleted based on these evaluations and, if necessary, additional items will be added. In addition to the written evaluations, a convenience sample of experts will assess the deleted items and the instrument verbally. This assessment may lead to the addition of items to the instrument and/or modifications in the wording of items that resulted in high levels of non-responses during the pre-test.
These changes will result in a second version of the instrument, which must be again pilot tested on a sample of the total population selected using the same sampling procedures and inclusion criteria as in the preliminary pilot test. Returned questionnaires are rejected if they have an inadequate amount of answers (<50% of the questions answered).
The data acquired during the second pilot study is then tested for construct validity by factor analysis. This phase includes checking the returned surveys for missing data, confirming participant responses to negatively worded items, and deleting variables that show no apparent correlation to any other variable [16]. It is important to note that the preliminary analysis, which was performed to assess the quality of data, is essential to reaching higher construct validity. Data missing values need to be assessed based on missing completely at random (MCAR) or missing not at random (MNAR) outcomes [17]. It has previously been recommended that listwise deletion should be applied when missing values account for more than 5% of the data, while mean imputation is a better alternative when less than 5% of the data are missing values [18]. Furthermore, uni- and multi-variate outliers should be identified and removed once the normal distribution of data has been verified. Once the data quality has been improved, researchers can perform exploratory factor analysis with different rotation methods (orthogonal- varimax or oblique- promax rotation) depending on the amount of correlation allowed between factors. EFA results are reported based on a correlation matrix of between-variable associations. General recommendations state that the data and correlation matrix should meet certain criteria, for example, satisfactory Bartlett’s test (p < 0.001) and Kaiser-Meyer-Olkin test (p > 0.60) results.
The factors identified through EFA are retained if they have eigenvalues >1.0, explain 5% of the variance in aspects of interest, or are important according to Cattell’s scree test [18, 19]. Variables are accepted if they show loadings ≥0.30 but <0.80 for at least one factor, or loadings between 0.20 and 0.30 loadings and communality ≥0.30. Hence, variables with low communality or loading values will be excluded from further analyses. Items are removed and EFA is repeated until an optimal EFA model is achieved. It is important to note that EFA needs to be performed again every time items are deleted so that the analysis can provide accurate item loadings. This demonstrates that researchers must have a good theoretical understanding of EFA before applying it to validate an instrument.
The internal consistency of an instrument can be tested by calculating Cronbach’s alpha coefficient, which is an indication of how well the items fit together conceptually. The functionality of the factors can be examined by calculating the item-total and inter-item correlations. The latter addresses the degree to which the items measure the same construct [20].
8.2 Discussion
8.2.1 Validity of the Instrument
Construct, face, and content validity all provide evidence of the overall validity of an instrument [14]. Lynn [10] recommends that content validity should be evaluated during several phases of instrument development [14] by panels comprising at least seven experts. Regarding the CVI values of items (I-CVI), several scholars have suggested that values ≥0.78 indicate excellent content validity [11, 12, 19]. The scale CVI (S-CVI), on the other hand, should exceed 0.90 to demonstrate excellent validity, while values between 0.70 and 0.80 demonstrate good content validity [11]. In cases where the expert panel includes five or fewer members, each item should have an I-CVI of 1.00 to ensure content validity. Moreover, the same experts should also unanimously agree that the instrument has good face validity, i.e., it is relevant for studying the phenomenon of interest.
The general recommendation is that the testing phase should involve more than five times the number of participants as there are items in the tested instrument. Furthermore, both the raw data and correlation matrix should meet the Bartlett and Kaiser-Meyer-Olkin criteria for factor analysis suitability (p < 0.001 and p > 0.60, respectively) [18, 21]. Researchers can also perform a scree test to avoid including non-significant factors. A factor analysis indicates good construct validity if the retained factors explain >5% of the total variance, their eigenvalues exceed 1.0, and their loadings and communalities are greater than 0.30.
The reliability of an instrument is typically assessed by testing it over two samples, during which researchers pay attention to the clarity of items as well as their logic [14, 19]. Cronbach’s alpha coefficients are calculated for the responses of both sample populations, individual items and factors based on at least three items [9]. It is generally accepted that an instrument has good internal consistency if the Cronbach’s alpha values for both sets of empirical data exceed 0.70 [21, 22], although there is disagreement about the ideal values of these coefficients. When an instrument has been designed for clinical applications, some authors have suggested that the Cronbach’s alpha values should ideally be at least 0.90 or 0.95 [9, 14]. However, DeVellis [21] suggests that Cronbach’s alpha coefficient values over 0.90 are indicative of redundancies and suggest a need to shorten the instrument.
In certain cases researchers may feel that Cronbach’s alpha coefficient has been artificially inflated by adding a large number of similar items to an instrument [21]. When this happens, it is beneficial to examine the correlation matrices of individual items as well as the item-total correlations [19, 22]. Items can then be deleted due to low (r < 0.30) inter-item correlations. In the cases in which all of these measures are examined, the item-total correlations, inter-item correlations, and alpha coefficients should all be high. Nevertheless, it should be noted that high values may potentially reflect unnecessary duplication of content across items and redundancy rather than homogeneity [22]. This is the reason that expert panels are commonly involved in instrument testing. Furthermore, alpha coefficients are sample-specific; hence, internal consistency results may substantially vary across samples.
8.3 Limitations
Defining and operationalising concepts that are relevant to nursing science is the main challenge that researchers face during instrument development. Using content analysis to operationalise a concept will also identify key contents and provide ways to measure the studied concept. Not every definition is expected to cover all of the factors of a specific phenomenon, but an instrument must include a clear definition before it can measure some construct. Another risk of instrument development is that patients’ and researchers’ understandings of the content of items may differ. Therefore, researchers should encourage respondents to contact them if any item in the questionnaire is unclear. To further mitigate this risk, researchers could ask patients to evaluate the instrument’s content at different stages of the instrument development process (e.g., pilot testing). However, it is important to keep in mind that researchers and patients have different theoretical perspectives of the studied phenomenon, which may further complicate the instrument development process.
Instrument development is also difficult in that there are no straightforward rules for how many items an instrument should include. Hence, the researcher must make some tough choices when deleting or adding items to the developed instrument. For example, retaining too many items may artificially inflate the Cronbach’s alpha value, which—even though a positive result in terms of internal reliability—may signal that the instrument includes too many items. On the other hand, deleting numerous items may improve homogeneity as well as utility in clinical practice, but may increase the risk that the instrument does not sufficiently cover each factor of the studied phenomenon.
In addition, the suitability of the applied analytical methods will inevitably affect the reliability of the results; hence, ensuring that data and correlation matrices meet Bartlett’s (p < 0.001) and Kaiser-Meyer-Olkin (p > 0.60) criteria is a good benchmark. However, it is important to note that the correlations between variables may increase when missing values are replaced with mean values. Furthermore, large sample sizes can sometimes contribute to part of the observed statistical significance and may lead to overestimates of the number of significant factors. For this reason, researchers may elect to use a scree test instead of eigenvalues to restrict the number of factors when the instrument is tested using a large sample.

Full access? Get Clinical Tree
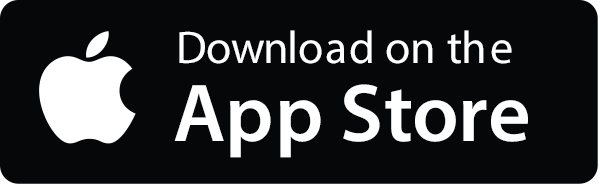
