14.1 Quantitative research
Quantitative research follows a very structured, reproducible and definitive stepwise approach. With quantitative analysis your next steps will be:





14.1.1 Data entry
Data entry and management can be a full time job, and for large studies this task may be contracted out to companies specialising in this area. However, for smaller studies you are likely to have to deal with your own data, using the software available in your institution.
Errors can be easily introduced during data entry and the following tips will help you to maintain accuracy:








14.1.2 Clean and check the data
Before you start to analyse your data it is essential to check it for errors. Although this is a boring task it is well worth doing to prevent much stress and re-analysis later. The method includes looking for unfeasible data, extremes within ranges, and obvious errors in data entry.
For example:





To do these checks use scatter plots to spot outliers, filter tools to spot erroneous categorical codes and audit tools to check validation rules are met; and frequency tables can also be useful to highlight mistakes. The precise methods of using computer software to undertake these checks are not covered in this text; refer to the manuals of the software you are using.
Once you have found any errors you will need to return to your data collection form to check the correct value. If you continue to be suspicious that the value is not accurate, and it is possible, check with another data source, such as the medical notes or the volunteer. If the value remains ambiguous it becomes missing data.
14.1.3 Carry out descriptive analyses
This analysis describes the characteristics of your sample and examines the distribution of data. At this stage you may also identify potential errors highlighted by unexpected average or variation values.
For all analyses you need the number of responses and the number of missing data. If you have large amounts of missing data your analysis and results may be compromised. However, small quantities of missing data are almost inevitable and are usually not a problem (although it should be reported). Of course always try to avoid missing data if you possibly can during the data collection phase.
Next, you need to characterise and describe your study group. For categorical variables you need to look at frequencies. This will give you the numbers of cases in each category, such as how many males and females. For continuous variables you need to look at the central tendency and amount of variation (see Chapter 8, Section 8.1.4 for more details of these terms).
In order to carry out the next step of analysis, you will need to investigate how your data are distributed; do they have a normal (or parametric) distribution or not (non-parametric)? This tells you which tests are suitable for your data as previously discussed in Section 8.1.5.3.
14.1.4 Analyse the data to answer the specific hypotheses you have defined in your protocol
At last you are ready to actually test the hypothesis you originally set out to examine. When designing your protocol you should have considered exactly what questions you want your data to answer and what a priori analysis you will do. You must now choose the correct tests to do these analyses and answer these questions. Since this should all be pre-planned this should not pose too many problems. However, you will need to learn how to do the right analysis with the software you are using.
14.1.5 Further analysis to explain results or to develop your theory
Your data may throw up some other interesting theories or you may have got an unexpected result and want to do further analyses to investigate why. Be careful about excessive analysis. If you analyse any set of data enough, you will by chance alone get a statistically significant result sooner or later. Be clear about what you are trying to find out and do this analysis only.
Sometimes ‘data dredging’ can show interesting relationships but these are seen as ‘hypothesis generating’ only. In other words you now need to design a new study to test your new hypothesis.
14.2 Qualitative research
Data management and analysis in qualitative research also follows a structured series of activities. However, unlike quantitative research, the analysis overlaps with data collection and processing, and the data are repeatedly re-examined as the analysis progresses. As soon as the first pieces of data are collected, analysis can begin and the initial findings may alter the focus of future data collection. As the analysis progresses, further questions may emerge and new connections may be identified, together with a deepening understanding of the data. Earlier data is re-examined to explore it in the light of the most recent understanding of the data and to confirm new connections or theories. The steps involved vary depending on the author you read or the underlying philosophy behind your research design but the following are the main steps you will need to go through (based on Colaizzi’s (1978) seven-step and Creswell’s (2003) six-step process):

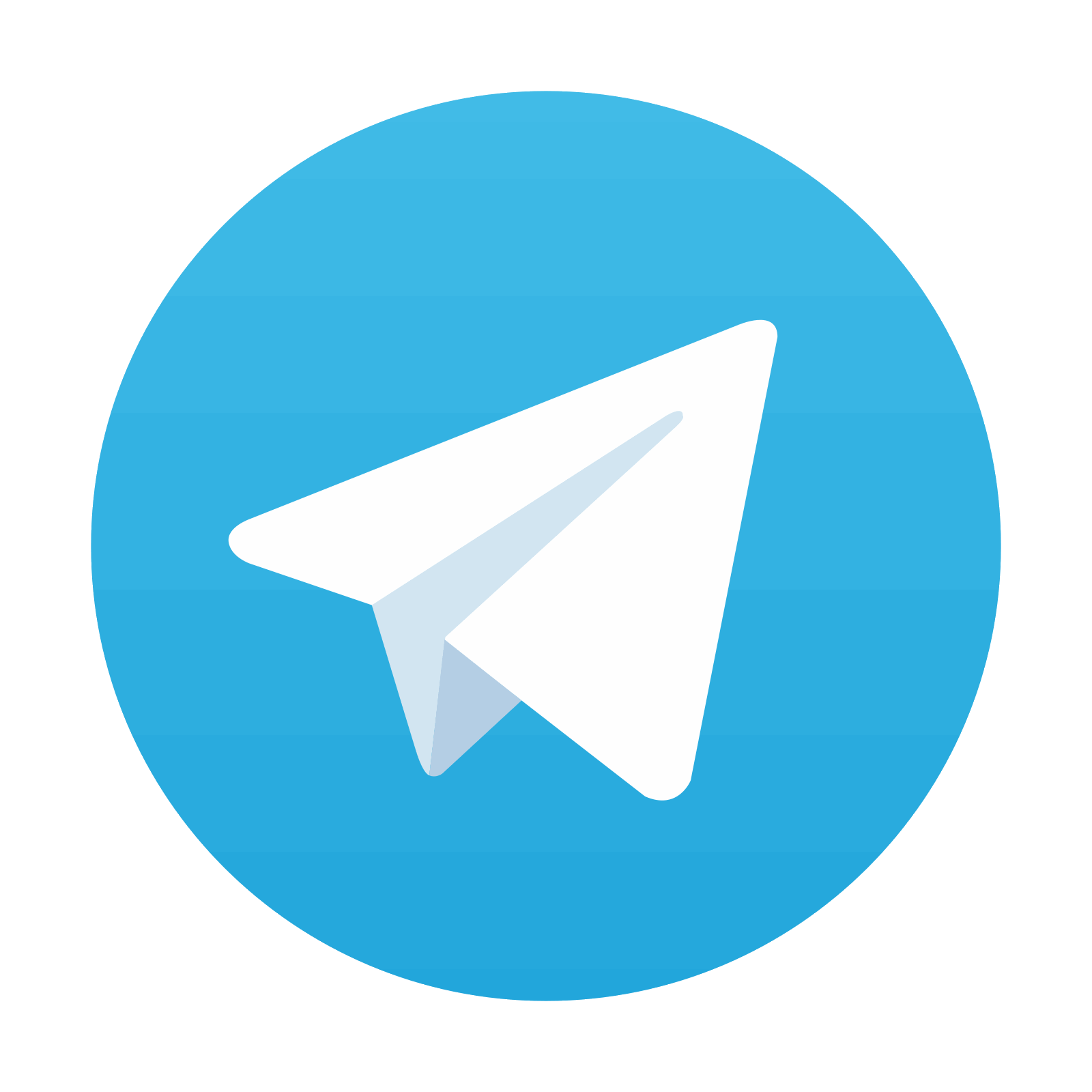
Stay updated, free articles. Join our Telegram channel

Full access? Get Clinical Tree
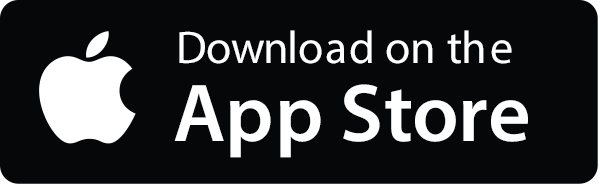
